Open Set Recognition for Encrypted Traffic using Intra-class Partition and Boundary Sample Generation
2022 IEEE 5th Advanced Information Management, Communicates, Electronic and Automation Control Conference (IMCEC)(2022)
摘要
Existing deep learning-based encrypted traffic recognition methods can achieve high precision identification performance while protecting user privacy, but almost all of them focus on closed sets, in which training data and test data come from the same label space. However, the real network environment is open, and it is necessary for traffic identification systems to deal with previously unseen categories. To classify known categories at a fine granularity and identify unknown ones, we propose a novel open-set recognition method for encrypted traffic. The decision boundary sample generation method based on constrained Generative Adversarial Networks is presented to generate samples from low-density regions in the known data space. Furthermore, an end-to-end open-set classifier is built using the accurate and diverse simulative samples of unknown classes through intra-class partition and boundary sample generation. Finally, the effectiveness of the proposed method is validated through numerical experiments.
更多查看译文
关键词
encrypted traffic recognition,open set,sample generation,deep learning
AI 理解论文
溯源树
样例
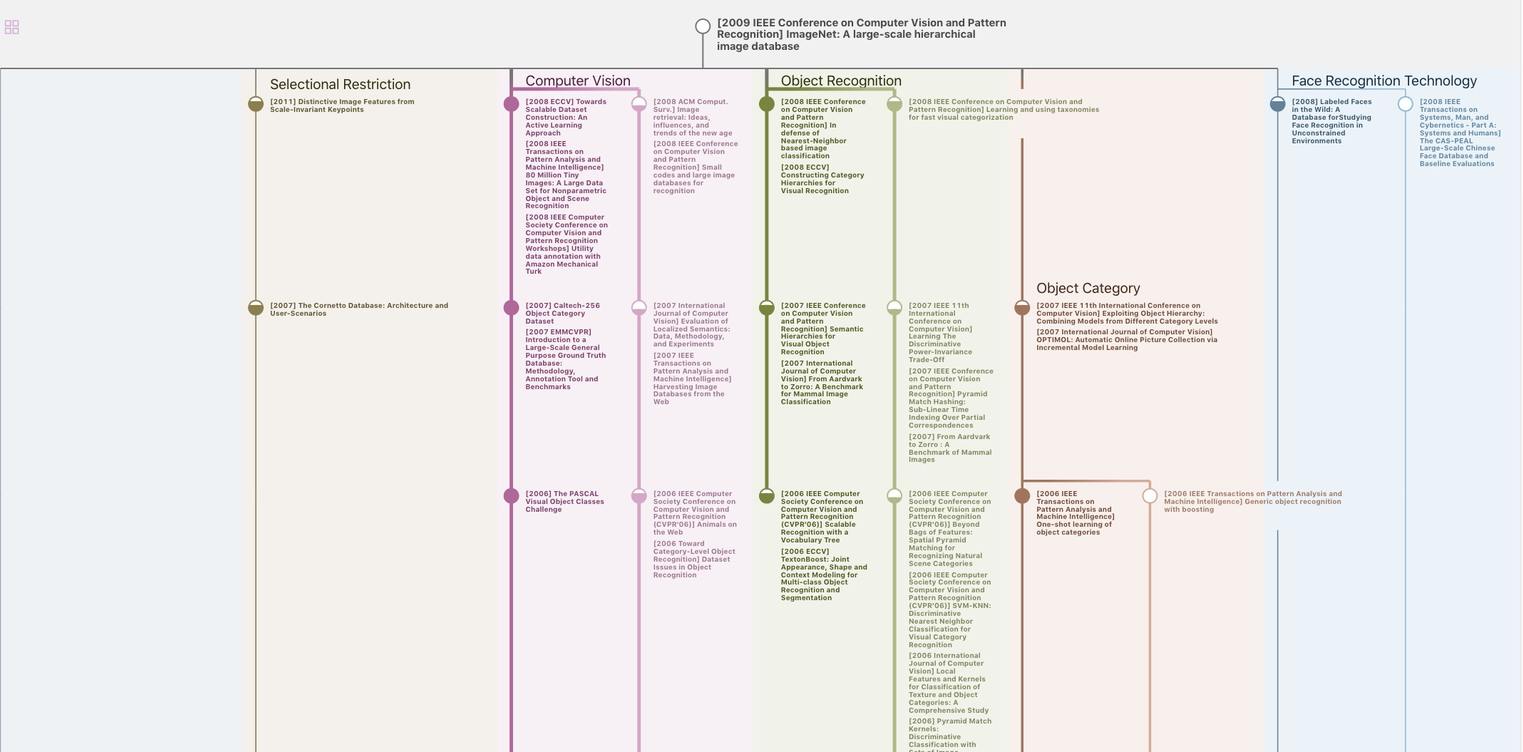
生成溯源树,研究论文发展脉络
Chat Paper
正在生成论文摘要