How far can the error estimation problem in data assimilation be closed by collocated data?
crossref(2022)
摘要
Abstract. Accurate specification of error statistics required for data assimilation remains an ongoing challenge, partly because their estimation is an ill-posed problem that requires statistical assumptions. Even with the common assumption that background and observation errors are uncorrelated, the problem remains underdetermined. One natural question that could arise is: Can the increasing amount of overlapping observations or other datasets help to reduce the total number of statistical assumptions, or do they introduce more statistical unknowns? In order to answer this question, this paper provides a conceptual view on the statistical error estimation problem for multiple collocated datasets, including a generalized mathematical formulation, an exemplary demonstration with synthetic data as well as a formulation of the minimal and optimal conditions to solve the problem. It is demonstrated that the required number of statistical assumptions increases linearly with the number of datasets. However the number of error statistics that can be estimated increases quadratically, allowing for an estimation of an increasing number of error cross-statistics between datasets for more than three datasets. The presented optimal estimation of full error covariance and cross-covariance matrices between dataset does not accumulate uncertainties of assumptions among estimations of subsequent error statistics.
更多查看译文
AI 理解论文
溯源树
样例
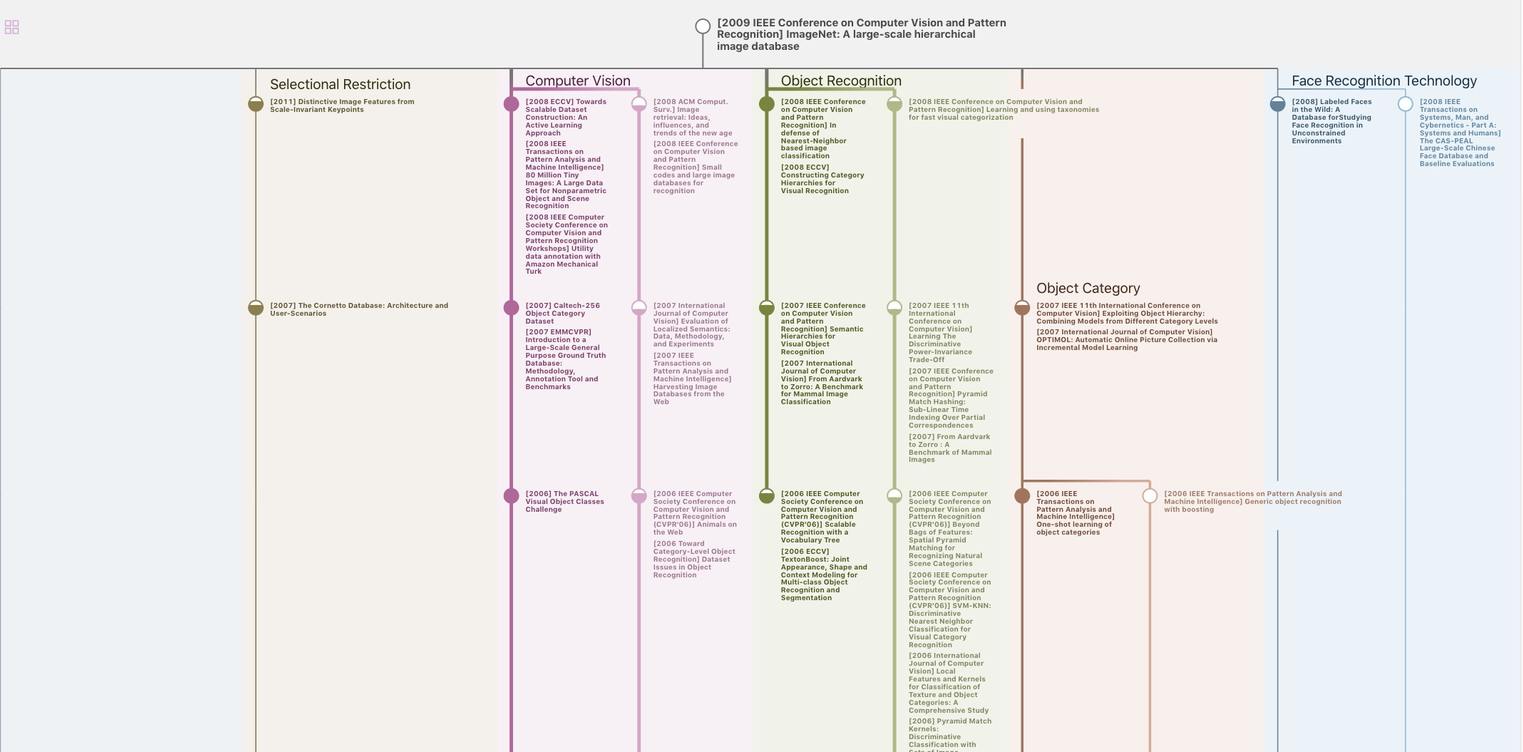
生成溯源树,研究论文发展脉络
Chat Paper
正在生成论文摘要