Auto-Associative LSTM for Multivariate Time Series Imputation
2022 41st Chinese Control Conference (CCC)(2022)
摘要
The existence of missing values increases the difficulty of data mining. In this paper, we design an auto-associative long short-term memory network (AALSTM) for handling time series, and proposed an AALSTM based imputation method (AALSTM-I) to estimate missing values of multivariate time series. The output of AALSTM is the estimation of its input. So not only history information but also current information will be utilized to produce estimation. The network of AALSTM is divided into several groups in case of identity mapping, and these networks share one group of weights to reduce the number of parameters. For handling missing values, an imputation unit is added to AALSTM to form AALSTM-I. In imputation unit, history information and current observed values are both used to estimate missing values. AALSTM-I can directly be trained with incomplete data without setting the initial values of missing values. The experiment results on several datasets verify the effectiveness of AALSTM and AALSTM-I.
更多查看译文
关键词
auto-associative
AI 理解论文
溯源树
样例
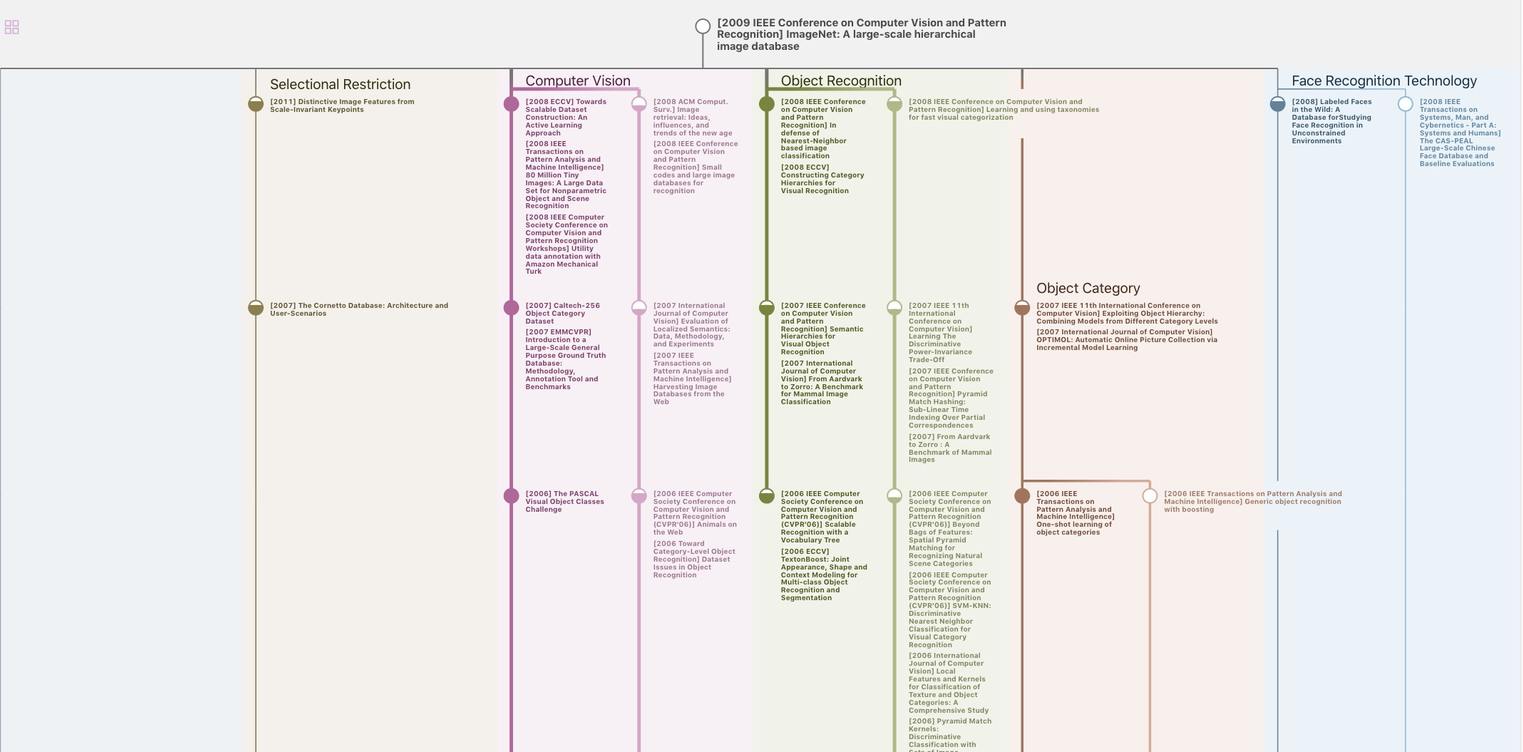
生成溯源树,研究论文发展脉络
Chat Paper
正在生成论文摘要