MELLODDY: Cross-pharma Federated Learning at Unprecedented Scale Unlocks Benefits in QSAR without Compromising Proprietary Information
JOURNAL OF CHEMICAL INFORMATION AND MODELING(2023)
摘要
Federated multipartner machine learning has been touted as an appealing and efficient method to increase the effective training data volume and thereby the predictivity of models, particularly when the generation of training data is resource-intensive. In the landmark MELLODDY project, indeed, each of ten pharmaceutical companies realized aggregated improvements on its own classification or regression models through federated learning. To this end, they leveraged a novel implementation extending multitask learning across partners, on a platform audited for privacy and security. The experiments involved an unprecedented cross-pharma data set of 2.6+ billion confidential experimental activity data points, documenting 21+ million physical small molecules and 40+ thousand assays in on-target and secondary pharmacodynamics and pharmacokinetics. Appropriate complementary metrics were developed to evaluate the predictive performance in the federated setting. In addition to predictive performance increases in labeled space, the results point toward an extended applicability domain in federated learning. Increases in collective training data volume, including by means of auxiliary data resulting from single concentration high-throughput and imaging assays, continued to boost predictive performance, albeit with a saturating return. Markedly higher improvements were observed for the pharmacokinetics and safety panel assay-based task subsets.
更多查看译文
关键词
qsar,learning,unprecedented scale unlocks benefits,cross-pharma
AI 理解论文
溯源树
样例
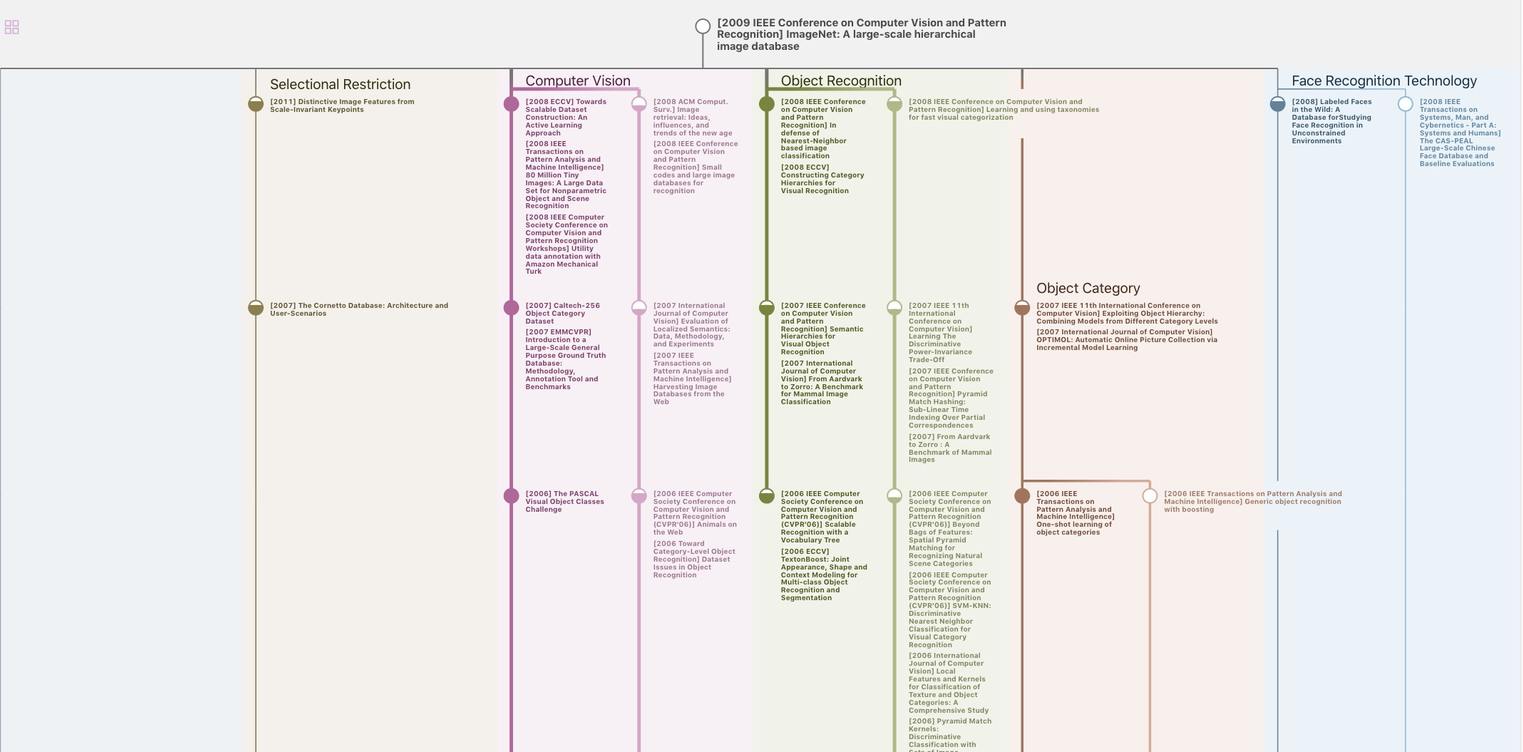
生成溯源树,研究论文发展脉络
Chat Paper
正在生成论文摘要