Reinforcement learning to help intensivists optimize mechanical ventilation settings (EZ-Vent): Derivation and validation using large databases
Research Square (Research Square)(2022)
摘要
Abstract Background Mechanical ventilation is the cornerstone of critical care medicine. However, choosing the optimal ventilator strategy for a patient remains imprecise. Existing guidelines provide one-size-fits-all recommendations, but do not personalize treatments for different intensive care unit (ICU) patients. In this study, we aimed to design and evaluate an artificial intelligence (AI) solution that could tailor an optimal ventilator strategy for each critically ill patient who requires mechanical ventilation. Methods We proposed a reinforcement learning-based AI solution using observational data from multiple ICUs in the US. The primary outcome was hospital mortality. Secondary outcomes were the proportion of optimal oxygen saturation and the proportion of optimal mean arterial blood pressure. We trained our AI agent to learn each patients’ treatment trajectory and thus to recommend low/medium/high levels of three ventilator settings, namely the positive end-expiratory pressure, fraction of inspired oxygen and ideal body weight-adjusted tidal volume. Off-policy evaluation metrics were applied to evaluate the AI policy. Results We studied 5105 and 21595 patients’ ICU stays from the Multiparameter Intelligent Monitoring in Intensive Care (MIMIC-IV) and eICU Collaborative Research (eICU) databases respectively. Observed hospital mortality rates were 18.2% (eICU) and 31.1% (MIMIC-IV). For the learnt AI policy, we estimated the hospital mortality rate (eICU 14.7\(\pm\)0.7%; MIMIC-IV 29.1\(\pm\)0.9%), proportion of optimal oxygen saturation (eICU 57.8\(\pm\)1.0%; MIMIC-IV 49.0\(\pm\)1.0%), and proportion of optimal mean arterial blood pressure (eICU 34.7 \(\pm\) 1.0%; MIMIC-IV 41.2\(\pm\)1.0%). Based on multiple quantitative and qualitative evaluation metrics, our proposed AI solution has potential to outperform observed clinical practice. Conclusions Our proposed approach has potential to be applied as a clinical decision support tool that helps intensivists make better treatment decisions and to improve the survival and prognosis of critically ill patients who require invasive respiratory support.
更多查看译文
关键词
mechanical ventilation settings,reinforcement,learning,ez-vent
AI 理解论文
溯源树
样例
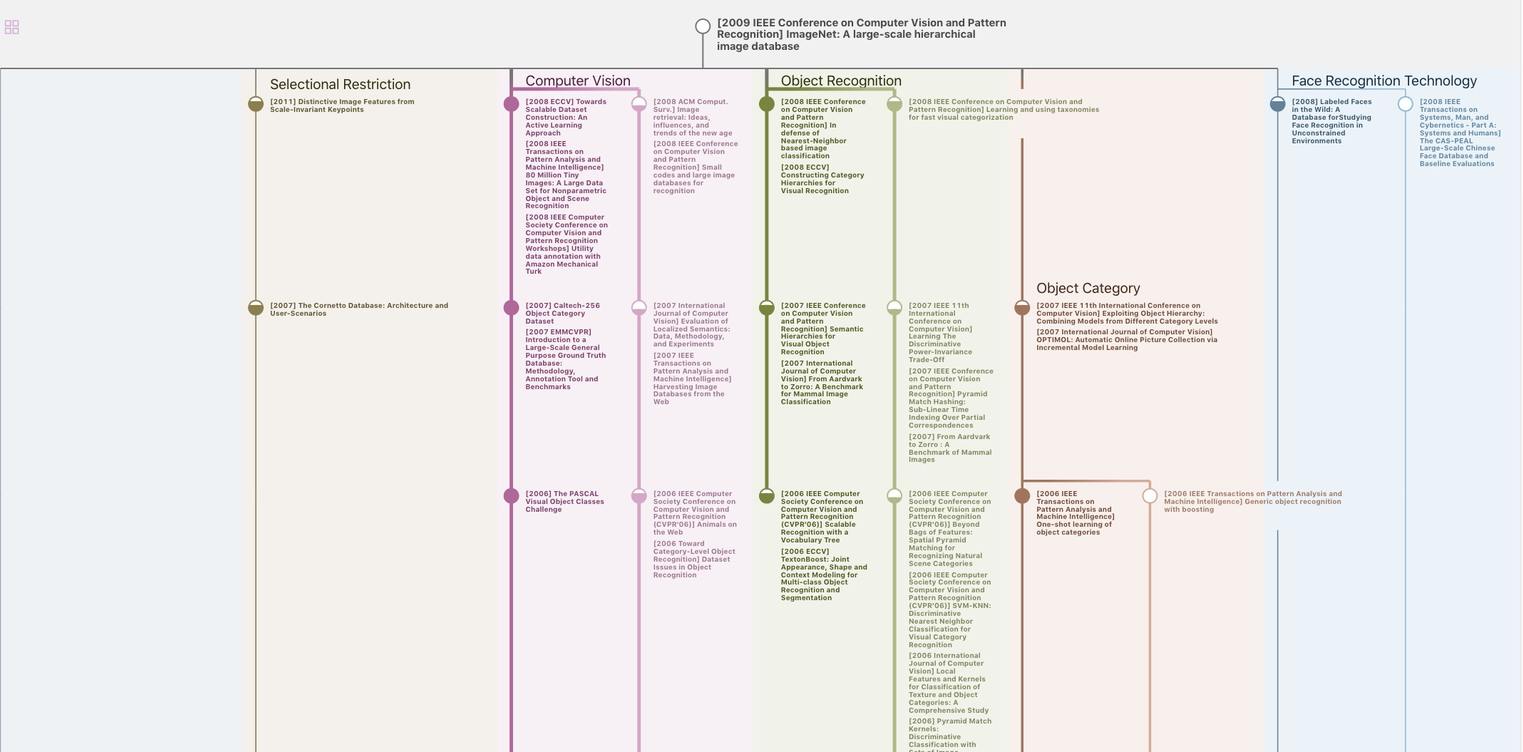
生成溯源树,研究论文发展脉络
Chat Paper
正在生成论文摘要