Short-term Probabilistic Trajectory Prediction Approach for Intelligent Connected Vehicle
Research Square (Research Square)(2022)
摘要
Abstract Efficient, high-precision and real-time vehicle trajectory prediction contributes more feasibilities to Intelligent Connected Vehicle (ICV) applications. Existing classic time series trajectory prediction methods attempt to directly extrapolate the exact future trajectory distribution from an extensive amount of trajectory data. However, these methods are sensitive to noise, require time series linearity and they are difficult to integrate traffic rules and potential environmental constraints, which makes multimodal prediction unreliable. For this purpose, this paper proposed a Probabilistic trajectory Prediction approach for ICV (PPIV), which is mainly applied to predict the vehicle trajectory within a short-term. Based on a large amount of real vehicle trajectory data, we explored and analyzed the vehicle motion features. Specifically, we divide the vehicle motion modes into two categories: single mode and complicated mode, and construct different trajectory proposal models for the two motion modes to predict the future trajectory location of the vehicle. Furthermore, we comprehensively consider the influence of vehicle location relationships and external hidden factors on vehicle trajectory prediction to improve prediction accuracy. The advantage of PPIV algorithm is that it not only predicts the future trajectory proposal of the vehicle under different motion modes but also predicts the probability distribution of all future trajectories of the vehicle. Simulation results with extensive real trajectory datasets show that the PPIV algorithm is superior to other probabilistic trajectory prediction algorithms.
更多查看译文
关键词
intelligent connected vehicle,prediction,short-term
AI 理解论文
溯源树
样例
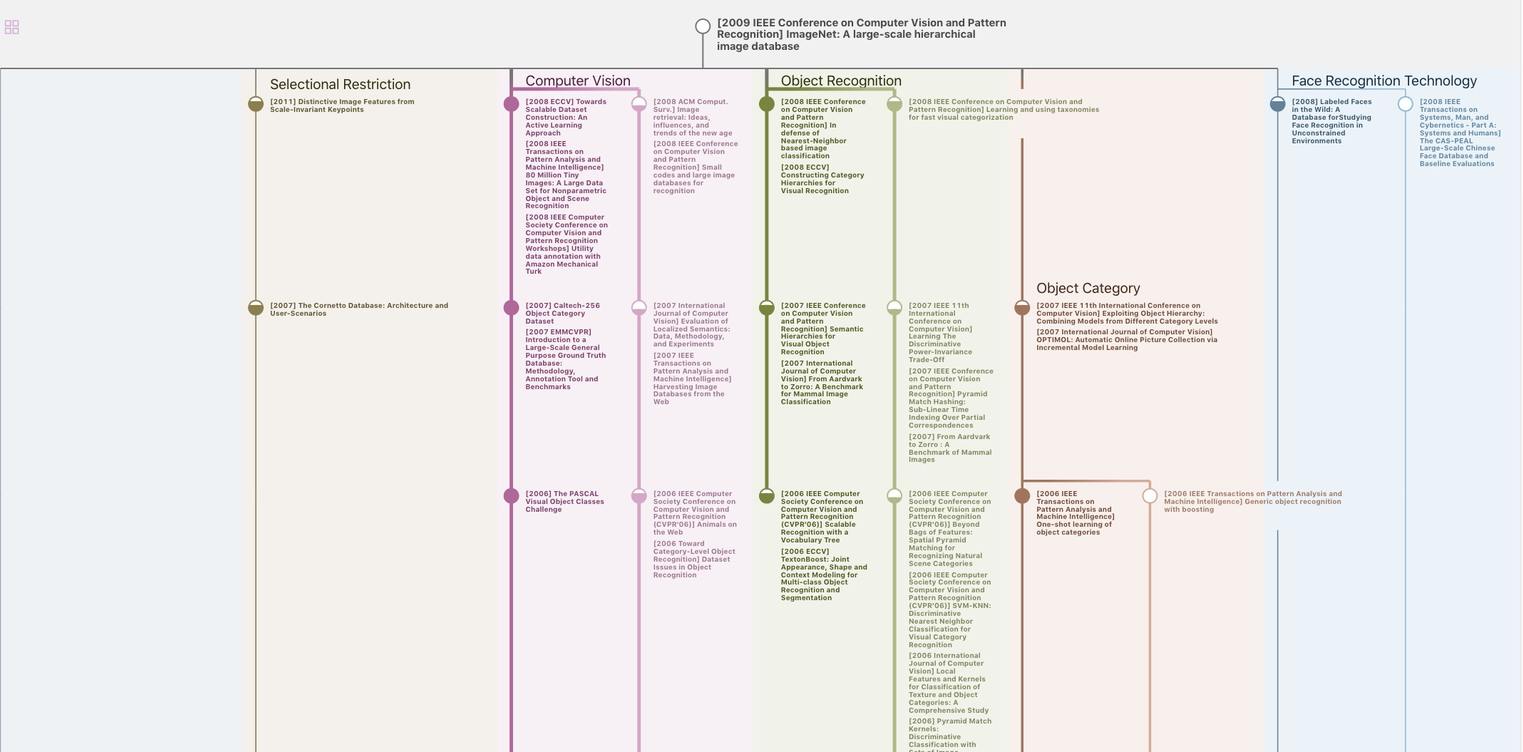
生成溯源树,研究论文发展脉络
Chat Paper
正在生成论文摘要