Semantic Relationship-based Embedding Models for Text Classification
crossref(2022)
摘要
Embedding representation models characterize each word as a vector of numbers with a fixed length. These models have been used in tasks involving text classification, such as recommen- dation and question-answer systems. Semantic relationships are words with a relationship between them providing a complete idea to a text. Therefore, it is hypothesized that an embedding model involving semantic relationships will provide better performance for tasks that use them. This paper presents three embedding models based on semantic relations extracted fromWikipedia to classify texts. The synonym, hyponym, and hyperonym semantic relationships were the ones considered in this work since previous experiments have shown that they are the ones that provide the most semantic knowledge. Lexical-syntactic patterns present in the literature were implemented and subsequently applied to the Wikipedia corpus to obtain the semantic relationships present in it. Several semantic relationships are used in different models: synonymy, hyponym-hyperonym, and a combination of the first two. A convolutional neural network was trained for text classification to evaluate the performance of each model. The results obtained were evaluated with the metrics of precision, accuracy, recall, and F1-measure. The best values obtained with the second model were accuracy of 0.79 for the 20-Newsgroup corpus. F1-measure and recall of 0.87 respectively for the Reuters corpus.
更多查看译文
AI 理解论文
溯源树
样例
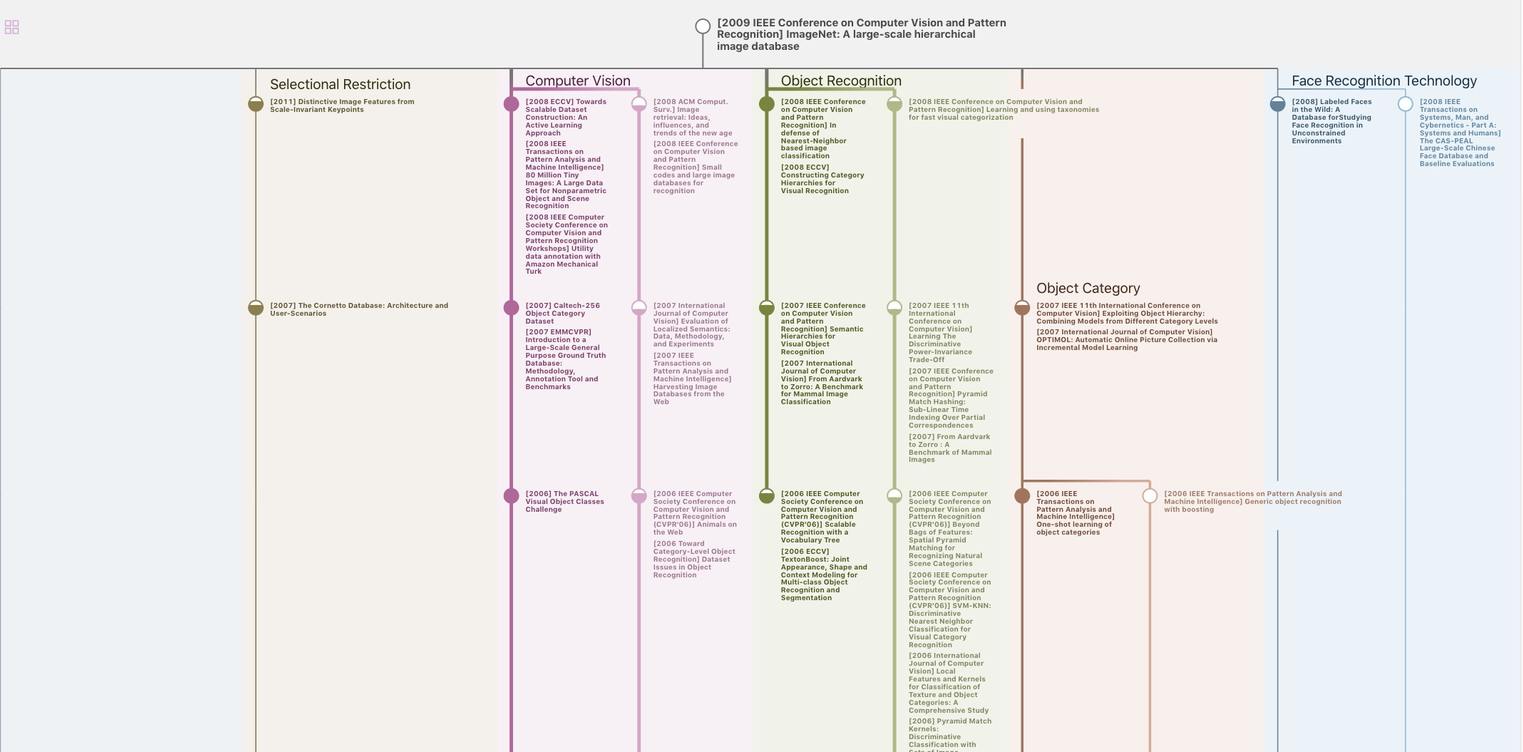
生成溯源树,研究论文发展脉络
Chat Paper
正在生成论文摘要