Learning-based non-fragile state estimation for switching complex dynamical networks
Discrete and Continuous Dynamical Systems - S(2023)
摘要
. This paper focuses on the state estimation problem for a class of switching complex dynamical networks (CDNs) with unknown nonlinear dynamics. A novel neural network based (NN-based) state estimator with non-fragility is constructed to fulfill the state estimation task. In order to improve the efficiency of communication resource utilization, an event-triggered mechanism is applied in designing the estimator. By employing the Lyapunov stability theory and inequality technique, sufficient criteria are derived for the existence of the desired exponentially ultimately bounded NN-based state estimator for CDNs. It is also proven that the proposed event-triggered mechanism can avoid Zeno phenomenon. In addition, the gains of the desired estimators are characterized by the explicit expressions. Finally, the effectiveness of the proposed NN-based state estimator is verified by a simulation example.
更多查看译文
关键词
complex dynamical networks,state estimation,learning-based,non-fragile
AI 理解论文
溯源树
样例
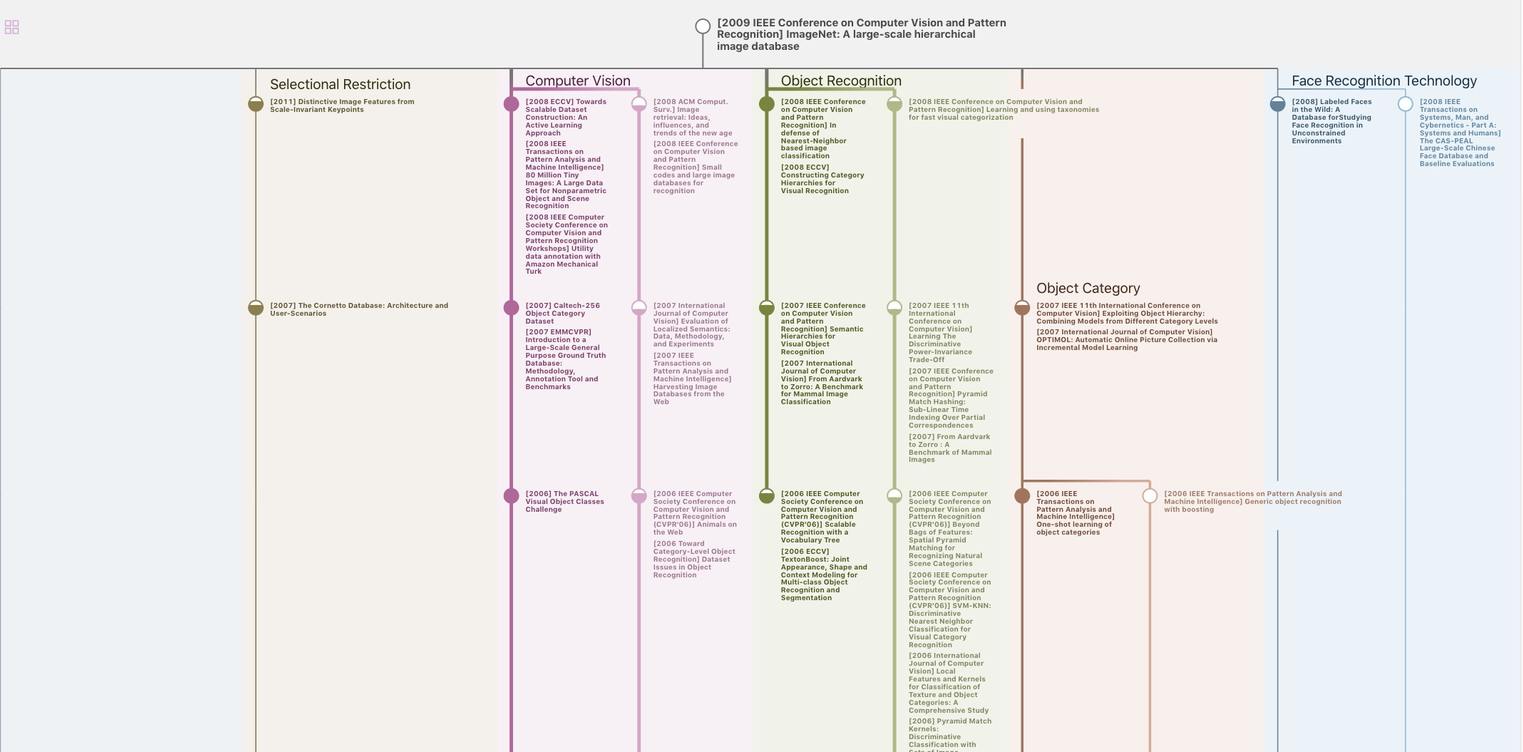
生成溯源树,研究论文发展脉络
Chat Paper
正在生成论文摘要