Partially Oblivious Congestion Control for the Internet via Reinforcement Learning
IEEE Transactions on Network and Service Management(2023)
摘要
Despite years of research on transport protocols, the tussle between in-network and end-to-end congestion control has not been solved. This debate is due to the variance of conditions and assumptions in different network scenarios, e.g., cellular versus data center networks. Recently, the community has proposed a few transport protocols driven by machine learning, nonetheless limited to end-to-end approaches. In this paper, we present Owl, a transport protocol based on reinforcement learning, whose goal is to select the proper congestion window learning from end-to-end features and network signals, when available. We show that our solution converges to a fair resource allocation after the learning overhead. Our kernel implementation, deployed over emulated and large scale virtual network testbeds, outperforms all benchmark solutions based on end-to-end or in-network congestion control.
更多查看译文
关键词
oblivious congestion control,reinforcement learning,internet
AI 理解论文
溯源树
样例
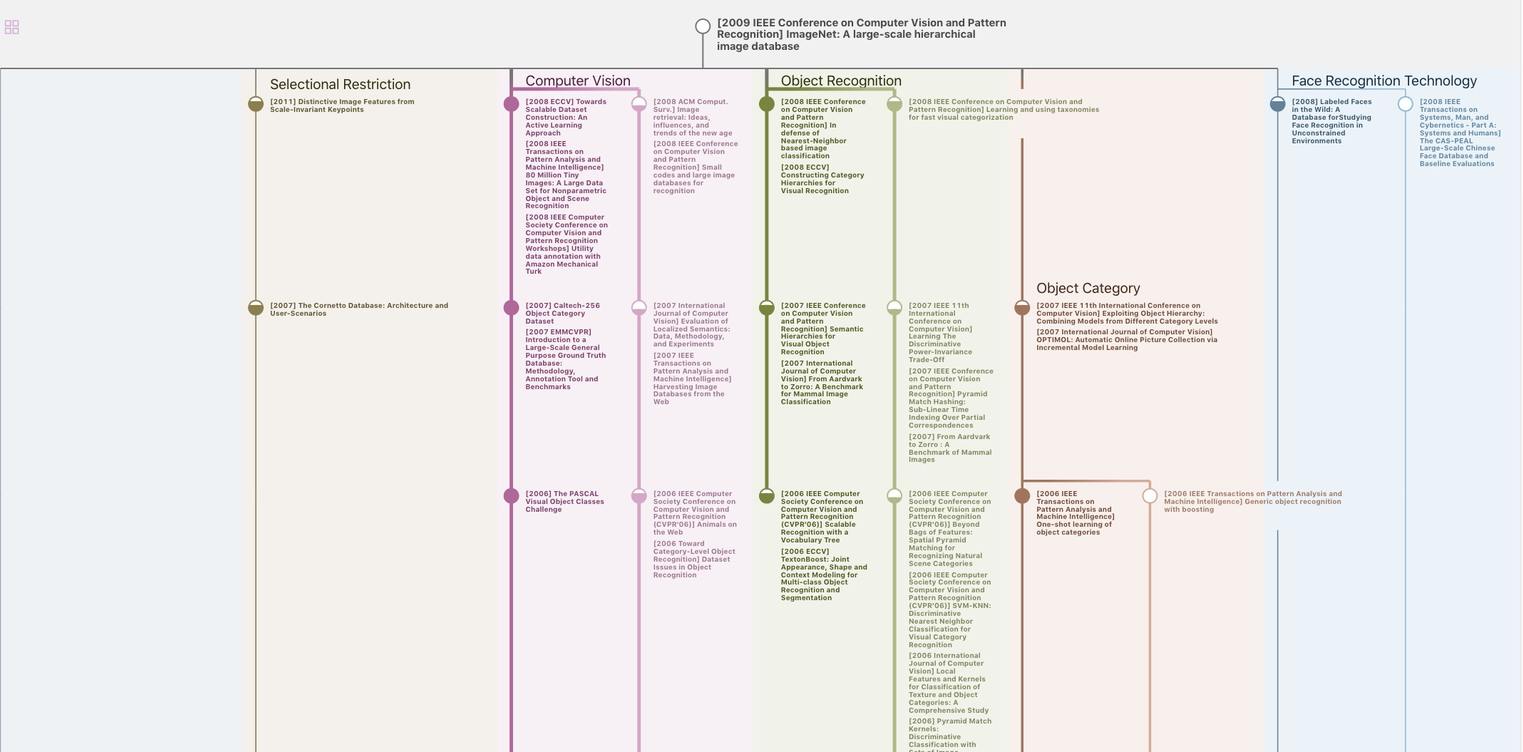
生成溯源树,研究论文发展脉络
Chat Paper
正在生成论文摘要