Adaptive Sampling and Quick Anomaly Detection in Large Networks
IEEE TRANSACTIONS ON AUTOMATION SCIENCE AND ENGINEERING(2023)
摘要
The monitoring of data streams with a network structure have drawn increasing attention due to its wide applications in modern process control. In these applications, high-dimensional sensor nodes are interconnected with an underlying network topology. In such a case, abnormalities occurring to any node may propagate dynamically across the network and cause changes of other nodes over time. Furthermore, high dimensionality of such data significantly increased the cost of resources for data transmission and computation, such that only partial observations can be transmitted or processed in practice. Overall, how to quickly detect abnormalities in such large networks with resource constraints remains a challenge, especially due to the sampling uncertainty under the dynamic anomaly occurrences and network-based patterns. In this paper, we incorporate network structure information into the monitoring and adaptive sampling methodologies for quick anomaly detection in large networks where only partial observations are available. We develop a general monitoring and adaptive sampling method and further extend it to the case with memory constraints, both of which exploit network distance and centrality information for better process monitoring and identification of abnormalities. Theoretical investigations of the proposed methods demonstrate their sampling efficiency on balancing between exploration and exploitation, as well as the detection performance guarantee. Numerical simulations and a case study on power network have demonstrated the superiority of the proposed methods in detecting various types of shifts.
更多查看译文
关键词
Adaptive sampling,anomaly detection,network,statistical process control
AI 理解论文
溯源树
样例
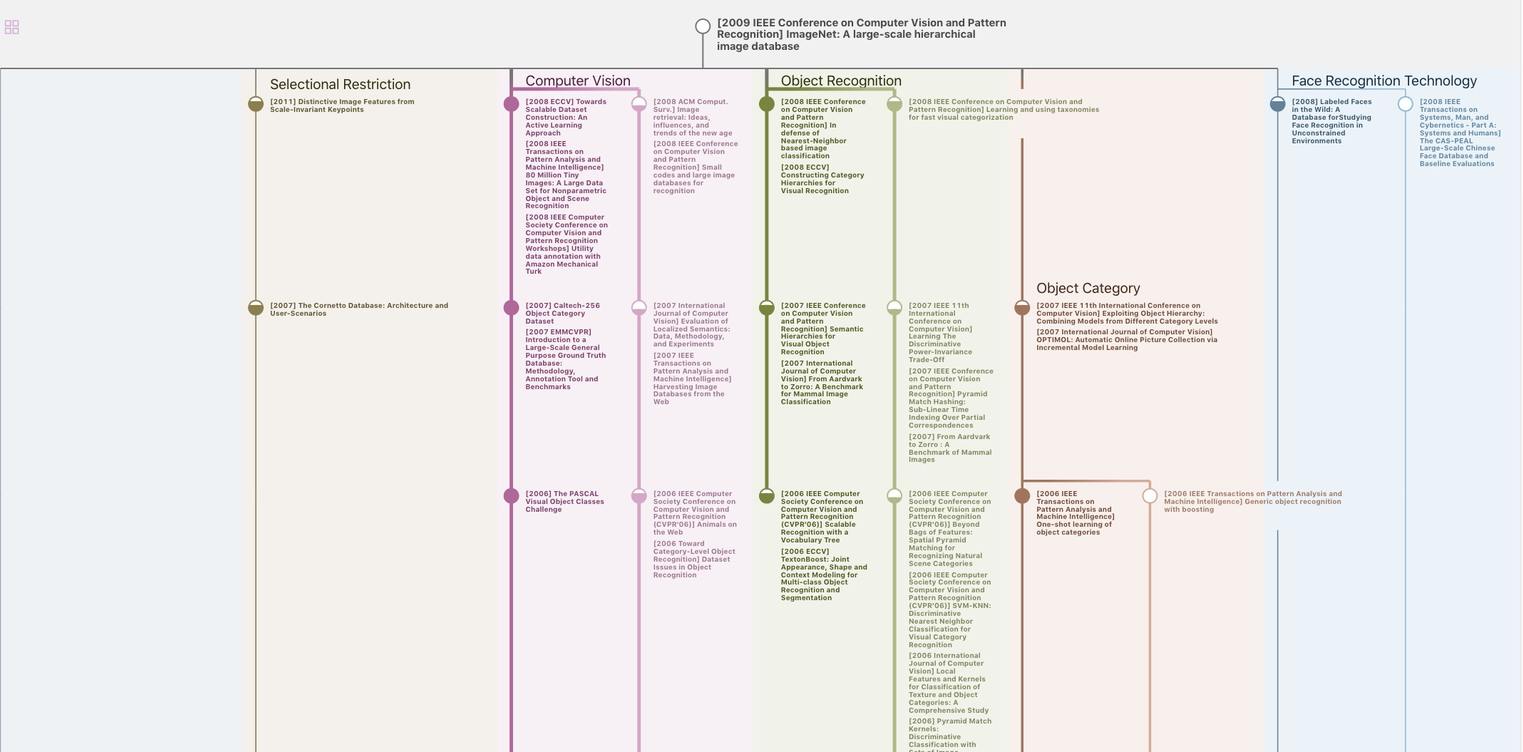
生成溯源树,研究论文发展脉络
Chat Paper
正在生成论文摘要