Beyond Traditional Doe: Deep Reinforcement Learning for Optimizing Experiments in Model Identification of Battery Dynamics
SSRN Electronic Journal(2022)
摘要
Model identification of battery dynamics is a central problem in energy research; many energy management systems and design processes rely on accurate battery models for efficiency optimization. The standard methodology for battery modelling is traditional design of experiments (DoE), where the battery dynamics are excited with many different current profiles and the measured outputs are used to estimate the system dynamics. However, although it is possible to obtain useful models with the traditional approach, the process is time consuming and expensive because of the need to sweep many different current-profile configurations. In the present work, a novel DoE approach is developed based on deep reinforcement learning, which alters the configuration of the experiments on the fly based on the statistics of past experiments. Instead of sticking to a library of predefined current profiles, the proposed approach modifies the current profiles dynamically by updating the output space covered by past measurements, hence only the current profiles that are informative for future experiments are applied. Simulations and real experiments are used to show that the proposed approach gives models that are as accurate as those obtained with traditional DoE but by using 85\% less resources.
更多查看译文
关键词
deep reinforcement learning,model identification,dynamics
AI 理解论文
溯源树
样例
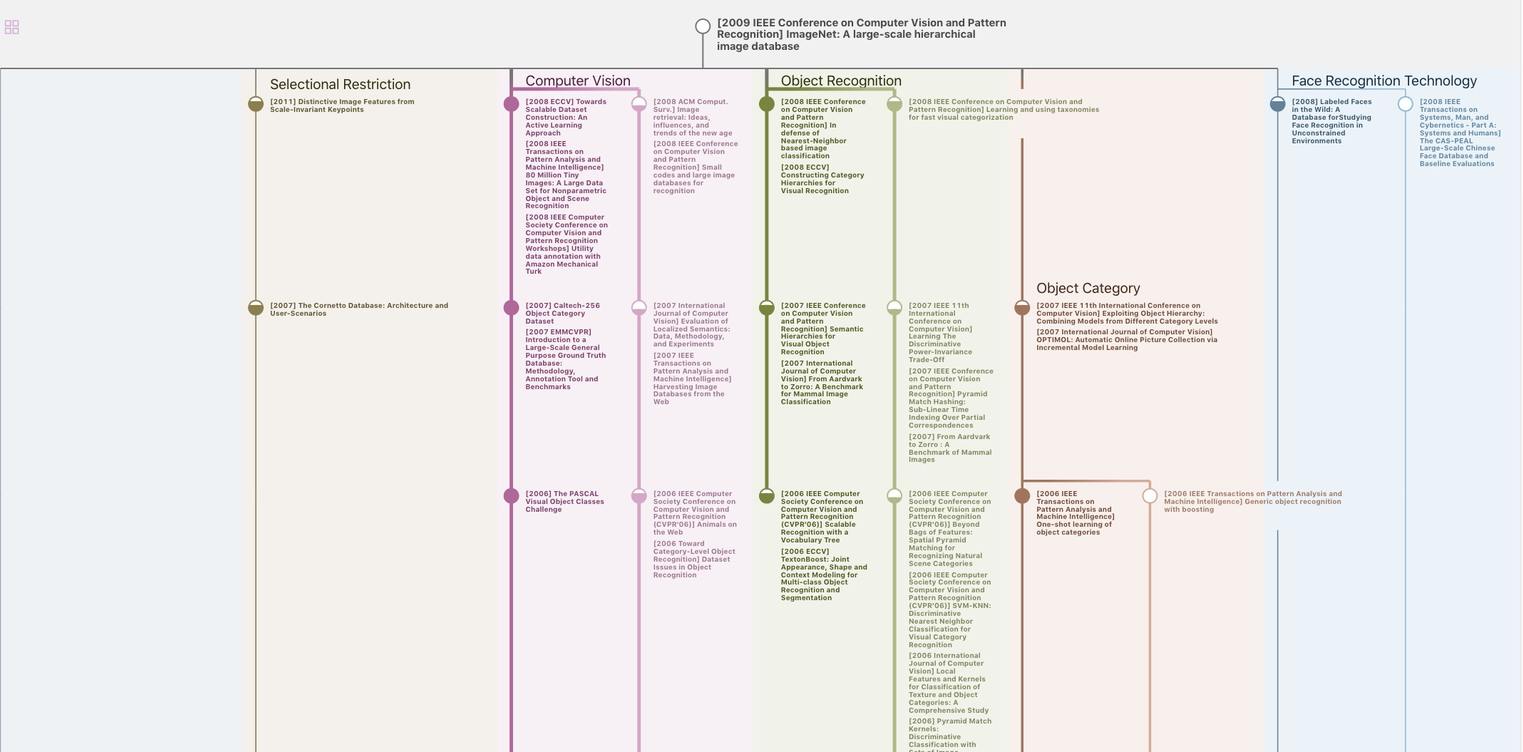
生成溯源树,研究论文发展脉络
Chat Paper
正在生成论文摘要