Multimodal Core Tensor Factorization and its Applications to Low-Rank Tensor Completion
IEEE TRANSACTIONS ON MULTIMEDIA(2023)
摘要
Low-rank tensor completion has been widely used in computer vision and machine learning. This paper develops a novel multimodal core tensor factorization (MCTF) method combined with a tensor low-rankness measure and a better nonconvex relaxation form of this measure (NC-MCTF). The proposed models encode low-rank insights for general tensors provided by Tucker and T-SVD and thus are expected to simultaneously model spectral low-rankness in multiple orientations and accurately restore the data of intrinsic low-rank structure based on few observed entries. Furthermore, we study the MCTF and NC-MCTF regularization minimization problem and design an effective block successive upper-bound minimization (BSUM) algorithm to solve them. Theoretically, we prove that the iterates generated by the proposed models converge to the set of coordinatewise minimizers. This efficient solver can extend MCTF to various tasks such as tensor completion. A series of experiments including hyperspectral image (HSI), video and MRI completion confirm the superior performance of the proposed method.
更多查看译文
关键词
Tensors,Matrix decomposition,Correlation,Task analysis,Hyperspectral imaging,Data models,Minimization,Low-rankness,nonconvex optimization,tensor,tensor factorization
AI 理解论文
溯源树
样例
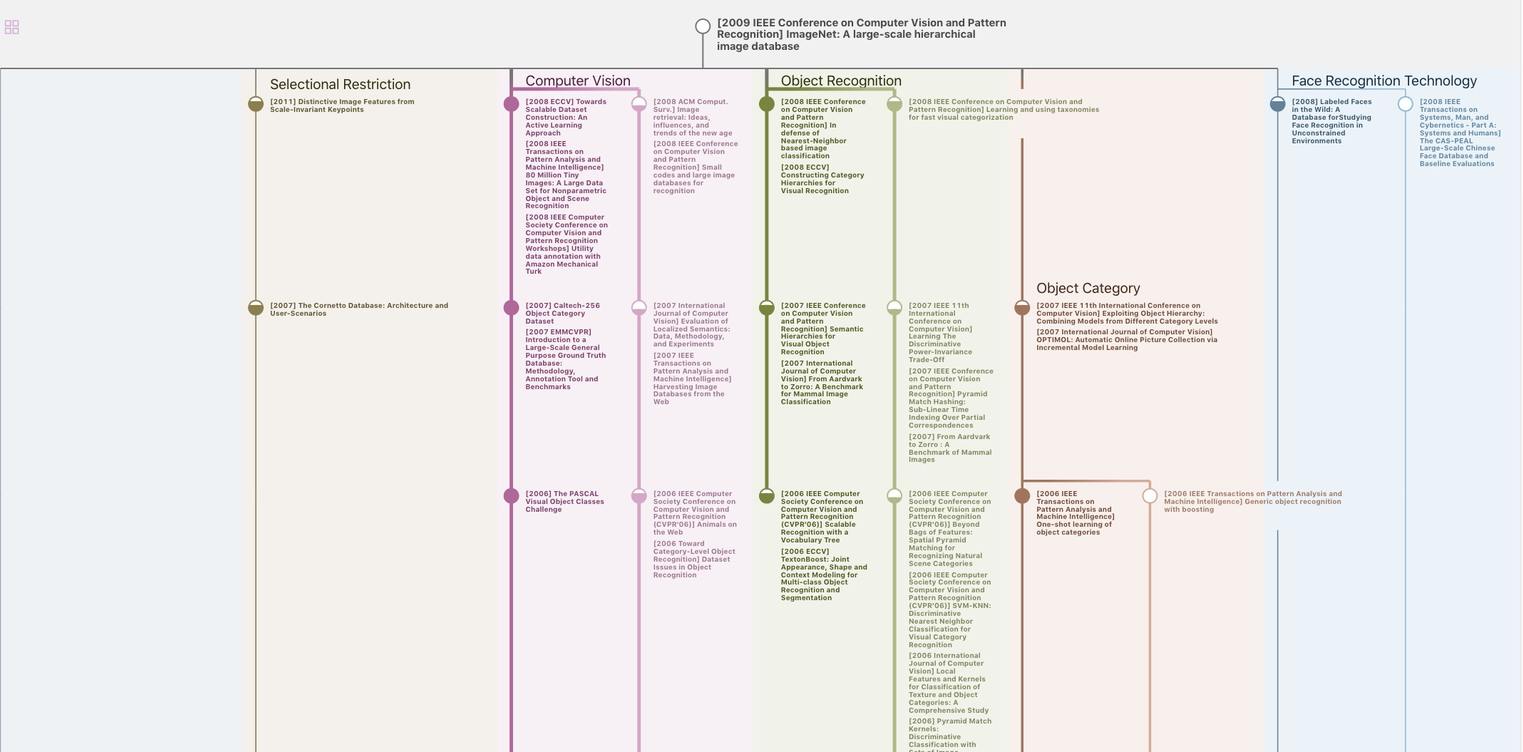
生成溯源树,研究论文发展脉络
Chat Paper
正在生成论文摘要