Redefined decision variable analysis method for large-scale optimization and its application to feature selection
SSRN Electronic Journal(2023)
摘要
Decision variable analysis (DVA) methods have provided the promising direction in solving large-scale multiobjective optimization problems (LMOPs). However, most existing DVA-based methods still have the problem of high evaluation consumption, which limits the performance of solving LMOPs. To alleviate this issue, this paper proposes a new redefined DVA method with low-consumption for solving LMOPs, called R-DVA. Specifically, R-DVA detects the interrelationship among convergent decision variables by using a distance-based hierarchical clustering method, which does not need to generate lots of solutions, thus saving a large number of evaluation consumption. In addition, to improve the performance of population, an evolutionary-state-oriented evolutionary (EsoE) strategy with two search models is designed in R-DVA, which can monitor the evolutionary state of population and perform different search models according to different evolutionary states. Specifically, the intra-group optimization model is used to accelerate the convergence speed when the population is in the exploration state. Moreover, a quality-based segmentation competitive swarm optimizer is designed in the inter-group optimization model, aiming to enhance the diversity of the population when the population is in the exploitation state. In this way, a good trade-off between the convergence and diversity can be achieved via the EsoE strategy. Finally, extensive experiments demonstrate that our proposed R-DVA achieves the best results in comparison to five state-of-the-art competitive algorithms on most adopted benchmark LMOPs and a real-world large-scale application, i.e., feature selection.
更多查看译文
关键词
Large-scale optimization,Decision variable analysis,Low consumption,Feature selection
AI 理解论文
溯源树
样例
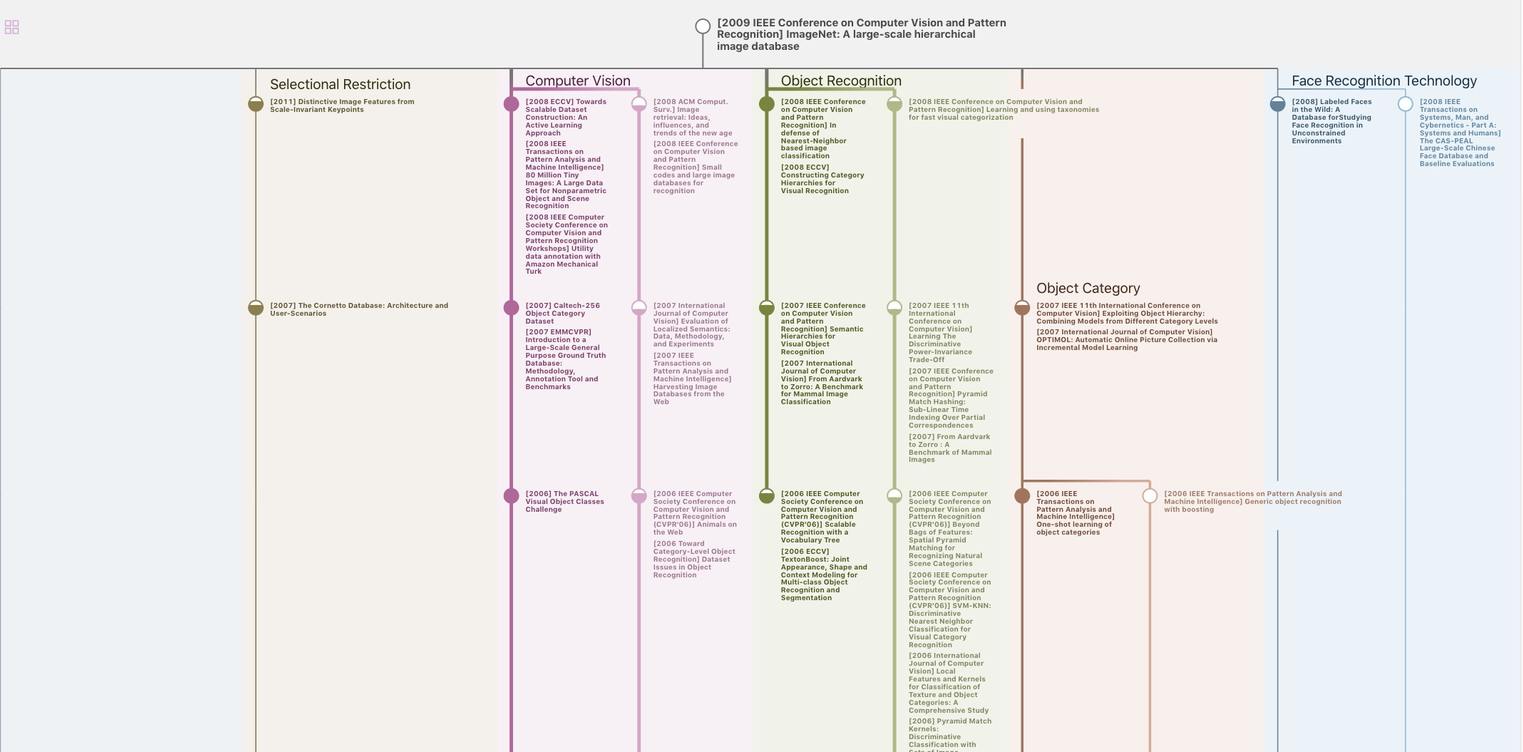
生成溯源树,研究论文发展脉络
Chat Paper
正在生成论文摘要