DeepEIT: Deep Image Prior Enabled Electrical Impedance Tomography
IEEE Transactions on Pattern Analysis and Machine Intelligence(2023)
摘要
Neural networks (NNs) have been widely applied in tomographic imaging through data-driven training and image processing. One of the main challenges in using NNs in real medical imaging is the requirement of massive amounts of training data – which are not always available in clinical practice. In this article, we demonstrate that, on the contrary, one can directly execute image reconstruction using NNs without training data. The key idea is to bring in the recently introduced deep image prior (DIP) and merge it with electrical impedance tomography (EIT) reconstruction. DIP provides a novel approach to the regularization of EIT reconstruction problems by compelling the recovered image to be synthesized from a given NN architecture. Then, by relying on the NN's built-in back-propagation and the finite element solver, the conductivity distribution is optimized. Quantitative results based on simulation and experimental data show that the proposed method is an effective unsupervised approach capable of outperforming state-of-the-art alternatives.
更多查看译文
关键词
deepeit image prior,tomography,electrical
AI 理解论文
溯源树
样例
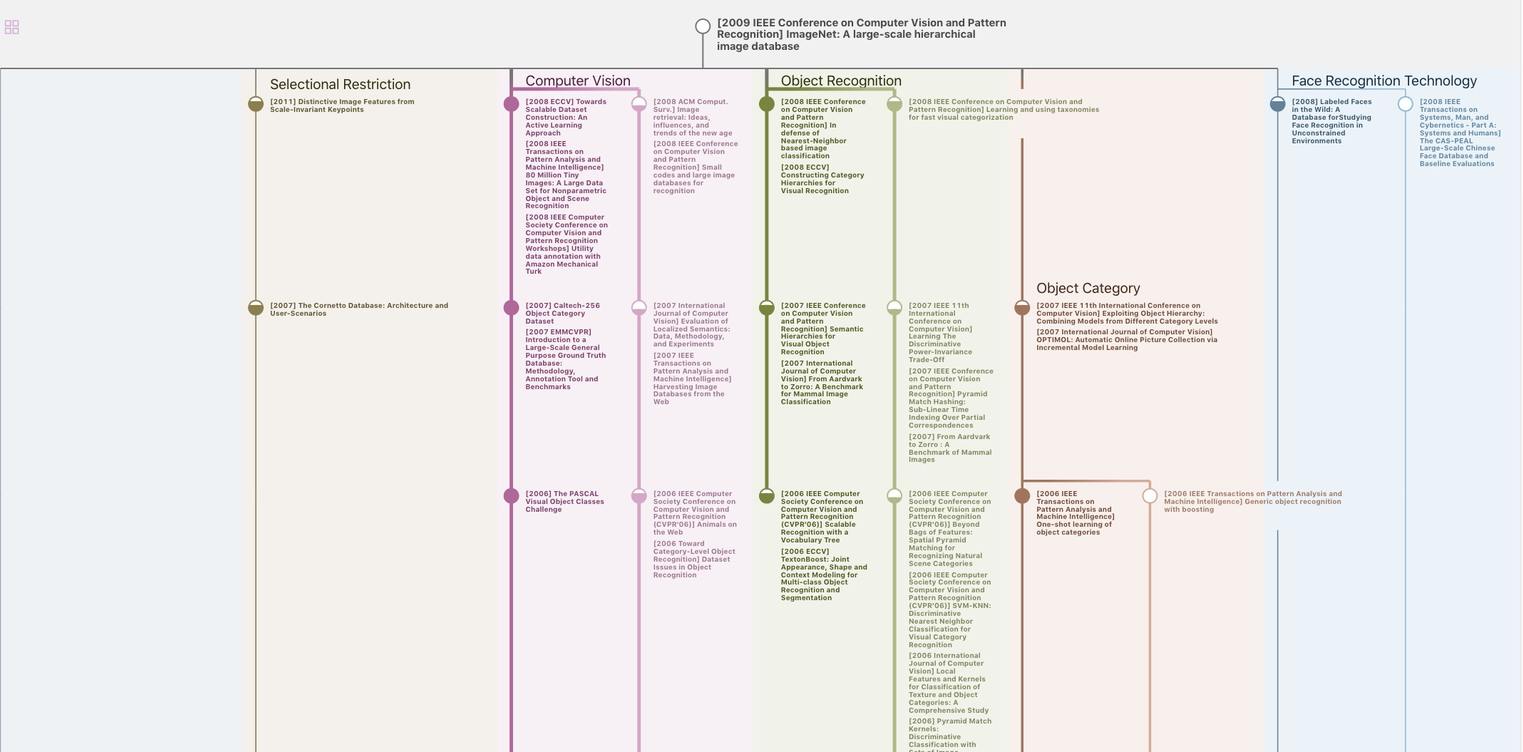
生成溯源树,研究论文发展脉络
Chat Paper
正在生成论文摘要