A machine learning study of grain boundary damage in Mg alloy
Materials Science and Engineering: A(2023)
摘要
Understanding the initiation of grain boundary (GB) damage is useful for designing Mg alloys with improved ductility. To this end, in-situ tensile tests were conducted for two Mg alloy samples of different textures. Prior to the two samples’ fracture, damages were observed at 5.8% and 7.5% of the GBs, respectively. Statistical correlations between GB damage and some GB features were identified. A machine learning model was further built for predicting whether a GB would initiate damage based on its feature values. After parameter optimization, that model achieves AUC (area under the receiver operator characteristic curve) scores of 73.7% and 84.1% for predicting the observed GB damages in the two samples. The most influential features are the crystallographic c-axis misalignment between the two adjacent grains, the average size of the two grains, and the GB inclination with respect to the loading axis. The reasons for those unpredicted GB damages are also discussed.
更多查看译文
关键词
Micromechanics, Fracture behavior, Magnesium alloys, Grains and interfaces
AI 理解论文
溯源树
样例
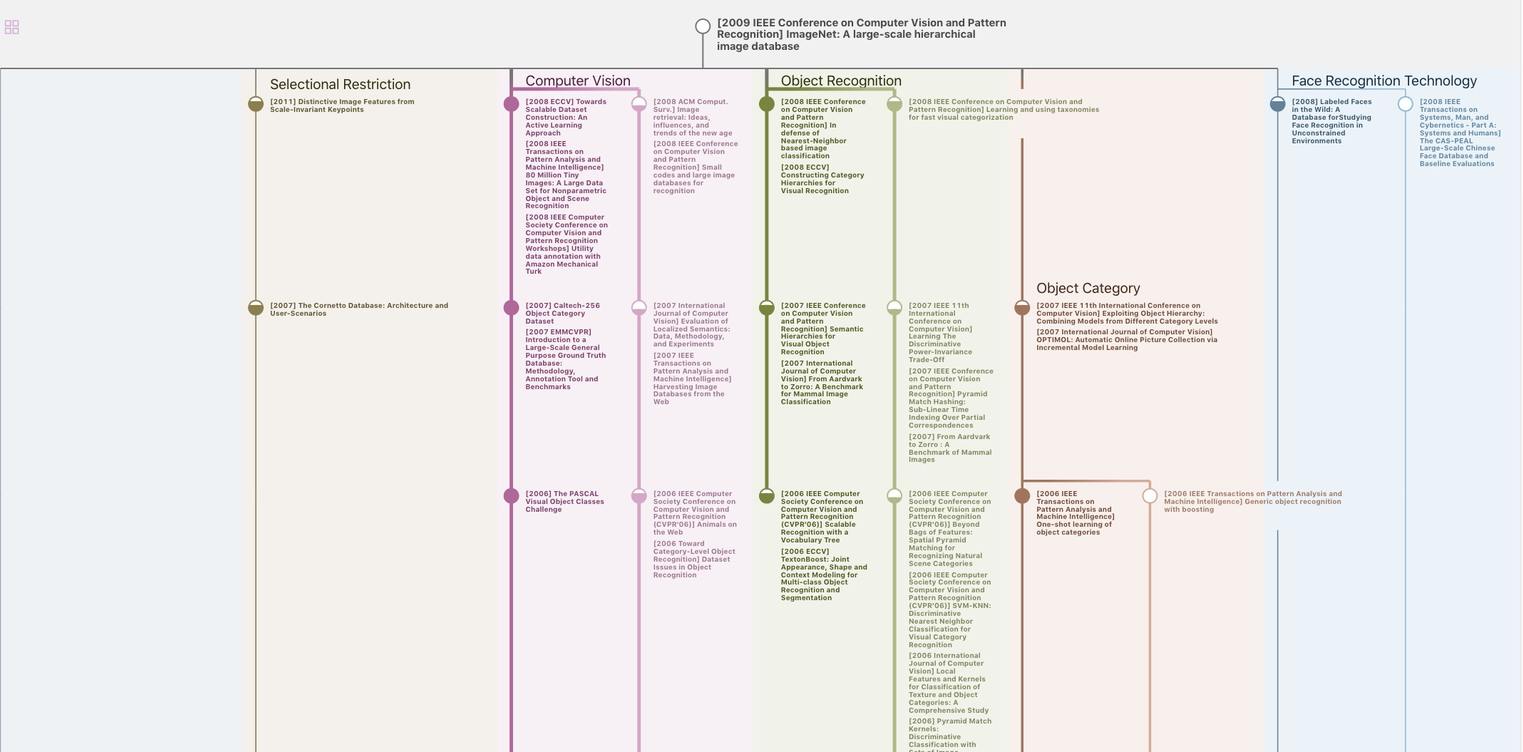
生成溯源树,研究论文发展脉络
Chat Paper
正在生成论文摘要