Data fusion uncertainty-enabled methods to map street-scale hourly NO2 in Barcelona city: a case study with CALIOPE-Urban v1.0
crossref(2022)
摘要
Abstract. Comprehensive monitoring of NO2 exceedances is imperative for protecting human health, especially in trafficked urban areas. However, accurate spatial characterization of exceedances is challenging due to the typically low density of air quality monitoring stations and the inherent uncertainties of urban air quality models. We study how observational data from different sources and time scales can be combined with a dispersion air quality model to obtain bias-corrected NO2 hourly maps at the street scale. We present a kriging-based data-fusion workflow that merges a dispersion model output with continuous hourly observations, and uses a machine-learning-based Land Use Regression (LUR) model constrained with past short intensive passive dosimeter campaigns observations. While the hourly observations allow to bias-adjust the temporal variability of the dispersion model, the microscale-LUR model adds information on the NO2 spatial patterns. Our method includes uncertainty calculation based on the estimated error variance of the Universal Kriging technique, which is subsequently used to produce urban maps of probability of exceeding the 200 µg /m3 hourly and the 40 µg /m3 NO2 annual average limits. We assess the statistical performance of this approach in the city of Barcelona for the year 2019. Our results show that simply merging the monitoring stations with the model output already significantly increases the correlation coefficient (r) by +29 % and decreases the Root Mean Square Error (RMSE) by -32 %. When adding the time-invariant LUR model in the data-fusion workflow, the improvement is even more remarkable: +46 % and -48 % for the r and RMSE, respectively. Our work highlights the usefulness of high-resolution spatial information in data-fusion methods to estimate exceedances at the street scale better.
更多查看译文
AI 理解论文
溯源树
样例
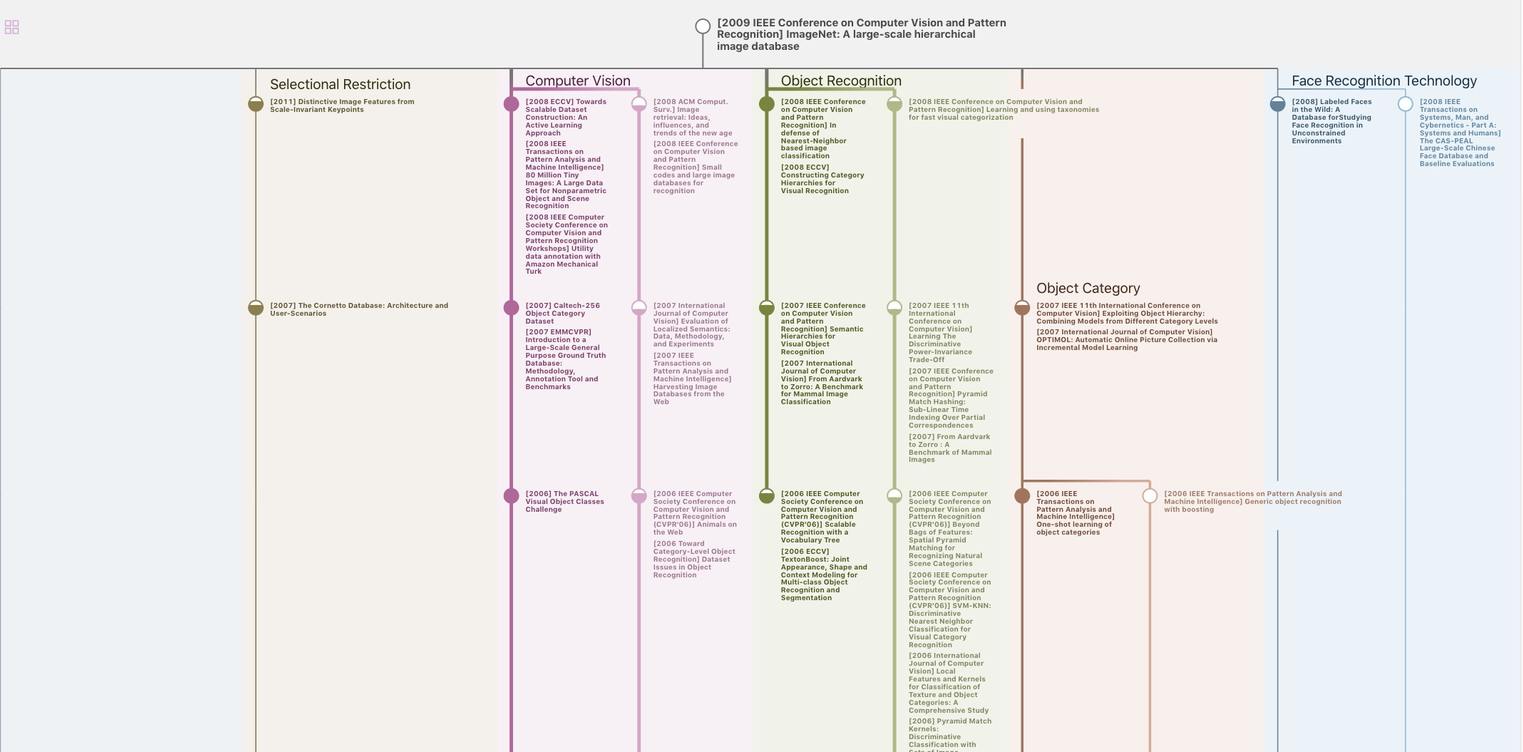
生成溯源树,研究论文发展脉络
Chat Paper
正在生成论文摘要