Multiple passive-sensor distributed target tracking approach with Machine Learning Feedback
EXPERT SYSTEMS WITH APPLICATIONS(2024)
摘要
In this paper, a novel recursive state estimation approach in three-dimensional space for a non-maneuvering target is developed. The proposed technique employs angle and amplitude information in a distributed fusion setup, tackling the issue of target state estimation in cluttered environments, as well as target detection with a machine learning feedback mechanism with sequence measurements from multiple passive sensors. A new Integrated Probabilistic Data Association technique with Machine Learning Feedback (IPDA-MLF) is proposed. Most of the clutter measurements are eliminated by fusing angles and amplitude information in the Local Sensor tracker with an Integrated Probabilistic Data Association algorithm (LS-IPDA) framework. To deal with low observability issues, as well as to fuse information from multiple sensors, a Machine Learning (ML) algorithm is deployed, which utilizes a convolutional neural networks-based algorithm. The proposed IPDA-MLF algorithm resolves possible track-to-track associations and provides feedback to the local tracker for increased track retention. The refined information from local trackers is again converted to heat maps and the ML-based solution is used to fuse the local tracks at a central level. Simulation results show that the performance of the proposed algorithm is improved by the incorporation of the machine learning feedback, reducing false tracks, increasing true track retention, and eliminating mismatched fusion at the central tracker.
更多查看译文
关键词
Multi-sensor target tracking,Distributed fusion,False track discrimination,Convolutional neural networks,Machine Learning,Mismatched fusion
AI 理解论文
溯源树
样例
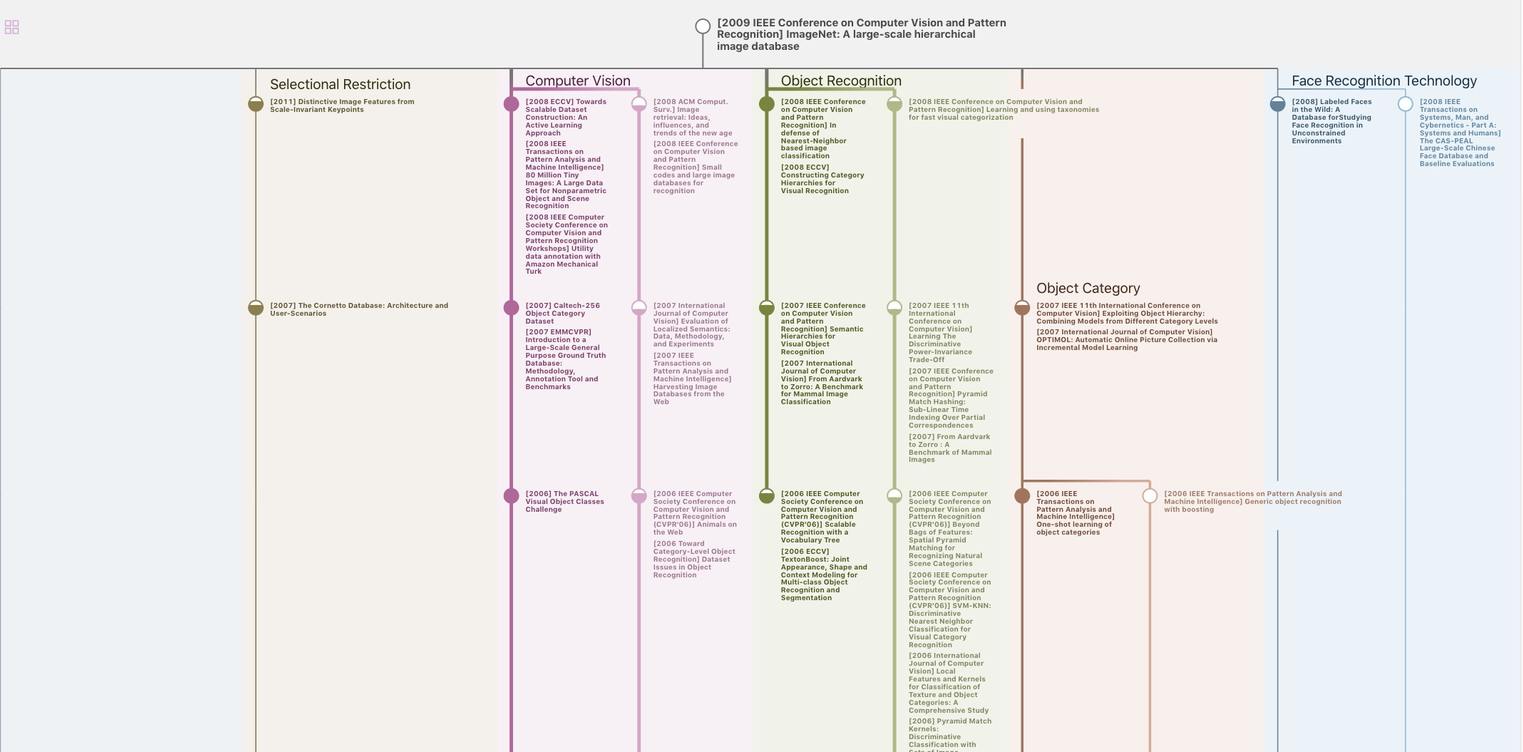
生成溯源树,研究论文发展脉络
Chat Paper
正在生成论文摘要