Abstract TP100: Transfer Learning Based Cerebral Microbleed Detection As An Mri Biomarker For Cerebral Amyloid Angiopathy Spectrum Diseases
Stroke(2023)
摘要
Objectives: Quantifying cerebral microbleeds (CMBs) in cerebral amyloid angiopathy (CAA) is important, however manual counting of CMBs on brain MRI is laborious. This work aims to develop a novel automatic deep learning segmentation algorithm for fast and accurate quantification of CMBs on brain MRIs. Method: We used (a) internal dataset (susceptibility-weighted imaging [SWI] clinical scans from 83 patients with CAA) with heterogeneous protocols on 1.5T and 3T scanners and manual CMB segmentation by 2 readers, and (b) public dataset (57 SWI scans from 30 patients with Alzheimer’s and CMB locations). The data was split into training, validation, and testing subsets (internal: 50/20/13; Public: 38/9/10). Preprocessing included N3 bias field correction, brain extraction, and up-sampling to a fixed in-plane resolution (0.2mm). Model used multi-channel 2D input (Fig1.b) and mimicked manual CMB segmentation. We trained base-model initially using the public dataset and then applied transfer learning using the internal dataset to segment CMBs in clinical scans. We evaluated model’s performance by sensitivity (S), precision (P), number of false positives per scan (FPC) and F1-score (F1). Results: On 10 public test scans, base model performed well (S 0.81, P 0.74, FPC 0.60, F1 0.77) but had high FPC for 13 internal test scans (S 1.00, P 0.15, FPC 4.31, F1 0.26). After transfer learning, the precision, FPC and F1-score were improved for internal scans (S 0.80, P 0.80, FPC 0.15, F1 0.80). Conclusion: Refined deep-learning based CMB counting algorithms could quantify CMBs for heterogeneous clinical MRI dataset with good sensitivity, precision, and false positivity. This method may accelerate further CAA clinical diagnosis and research.
更多查看译文
关键词
mri biomarker,amyloid,transfer learning
AI 理解论文
溯源树
样例
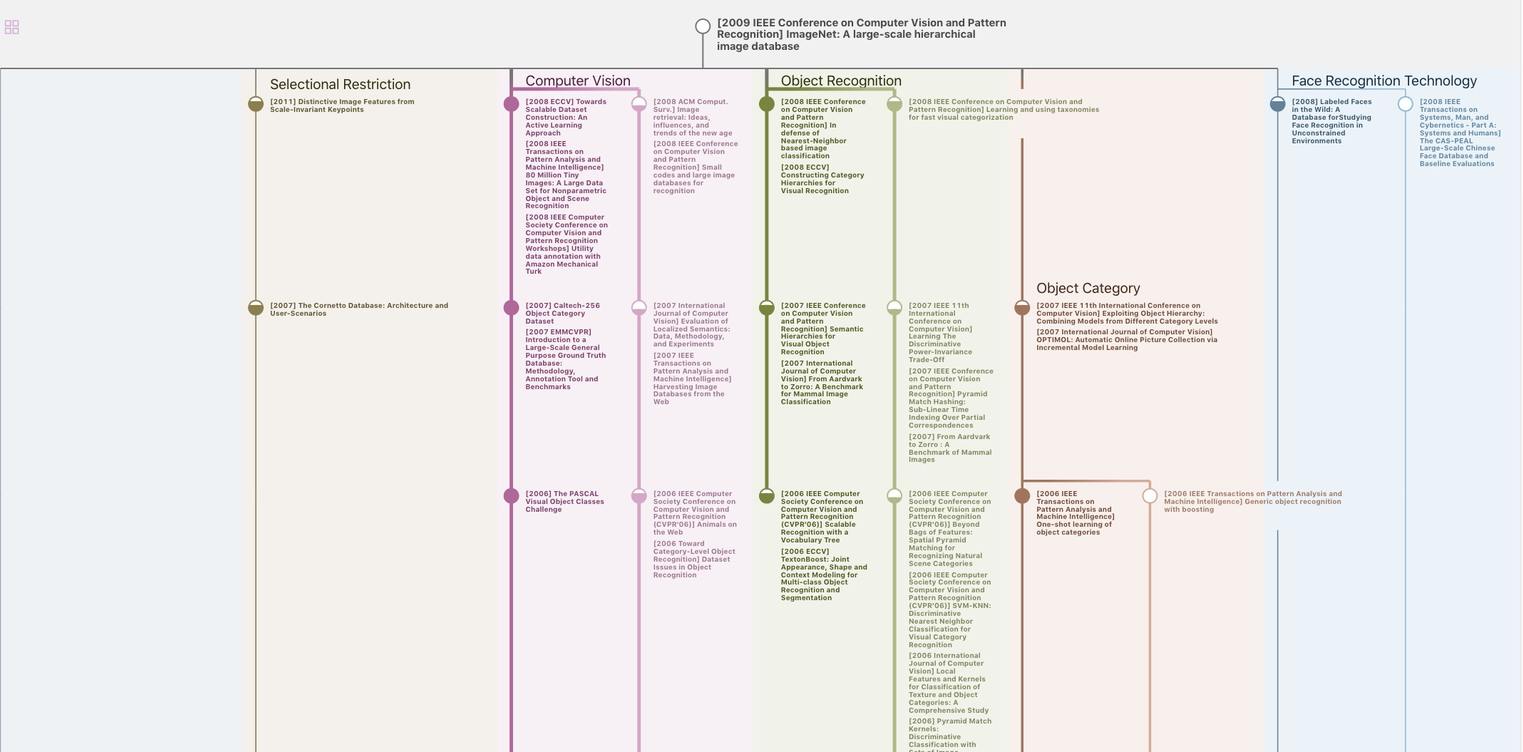
生成溯源树,研究论文发展脉络
Chat Paper
正在生成论文摘要