A coverless image steganography method based on invertible neural network
Third International Symposium on Computer Engineering and Intelligent Communications (ISCEIC 2022)(2023)
摘要
Recently, coverless image steganography (CIS) has become a hot topic in the field of image steganography. However, currently most CIS methods do not build an invertible two-way mapping between the secret information and image, so that the steganography capacity of CIS is limited. Moreover, the generated stego-images have a visible distinction between real-images, and it is difficult to deceive the deep-learning-based steganalysis, which makes CIS unsafe. To address the above issues, we propose a coverless image steganography method based on invertible neural networks, we call it as CIS-INN. Our method uses an invertible flow-based model, Glow model, and we combine it with adversarial generative network (GAN) to improve generated images quality. In encryption phase, secret information is encoded into latent variables by proposed Gray-code-based coding method, then the Glow model takes these latent variables as a prior and generates stego-images. To against steganalysis, we make CIS-INN more secure by introducing adversarial examples when generating stego-images. In decryption phase, secret information can be recovered through the reverse process of the glow model. Experiments demonstrate the CIS-INN achieves significant improvement of the steganography capacity (4 BPP) and maintains reliable security when confronted with multiple steganalysis methods.
更多查看译文
关键词
coverless image steganography method,invertible neural network,neural network
AI 理解论文
溯源树
样例
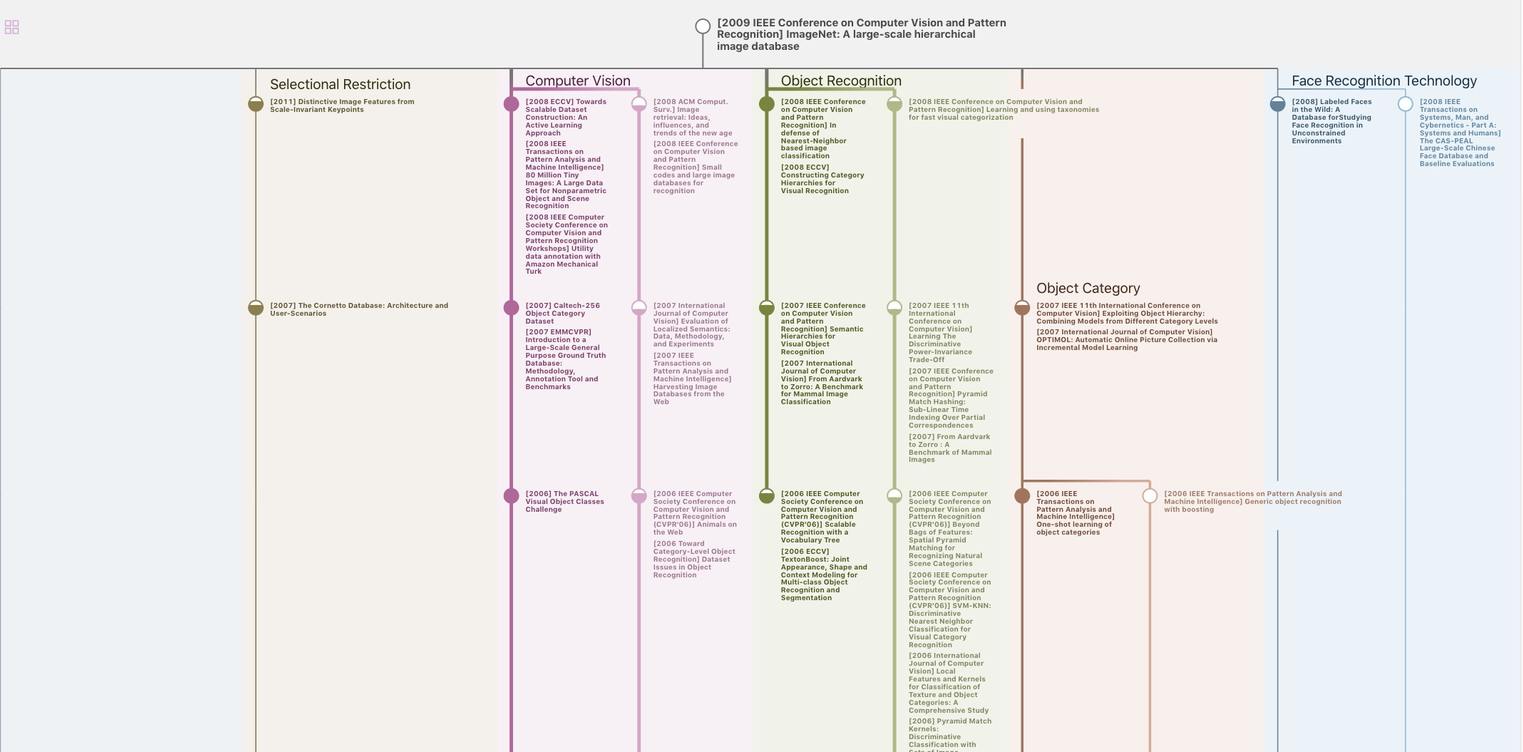
生成溯源树,研究论文发展脉络
Chat Paper
正在生成论文摘要