Semantic-aware Retrieval and Recommendation based on the Dirichlet Compound Language Model
Research Square (Research Square)(2022)
摘要
Abstract Traditional bag-of-word Information Retrieval (IR) has led to quasi-standard models (e.g. TF-IDF, BM25) that are applied in the context of recommendation. While the benefits of semantic-aware models are clear, there is no standard semantic IR model, and this prevents the usage of semantic retrieval for tasks such as recommendation. We propose a ranking framework by quantifying parameters of the Dirichlet Compound Model (DCM) and using query performance prediction to aggregate scores of semantic extensions of DCM. Instead of employing heuristics, we introduce reasonable aggregation standards which are expandable to the recommendation task by considering user interaction (e.g. rating) as a semantic event. This approach helps to bridge the gap between advanced IR and recommendation. One of the main findings of this paper is that the concept-based extension of DCM consistently outweighed both terms-only and concept-only baselines concerning biomedical data. The DCM-based recommender system is also shown to be effective when applied on MovieLens, Book Crossing and LastFM. By bringing together recommendation (prediction) and retrieval, we lay the grounds for generalizable and hybrid algorithms. This paper shows how advanced but well-established probability distributions can be transparently leveraged in the standardization of semantic ranking methods, especially for applications in which recommendation (notification) is critical.
更多查看译文
关键词
dirichlet compound language model,retrieval,recommendation,semantic-aware
AI 理解论文
溯源树
样例
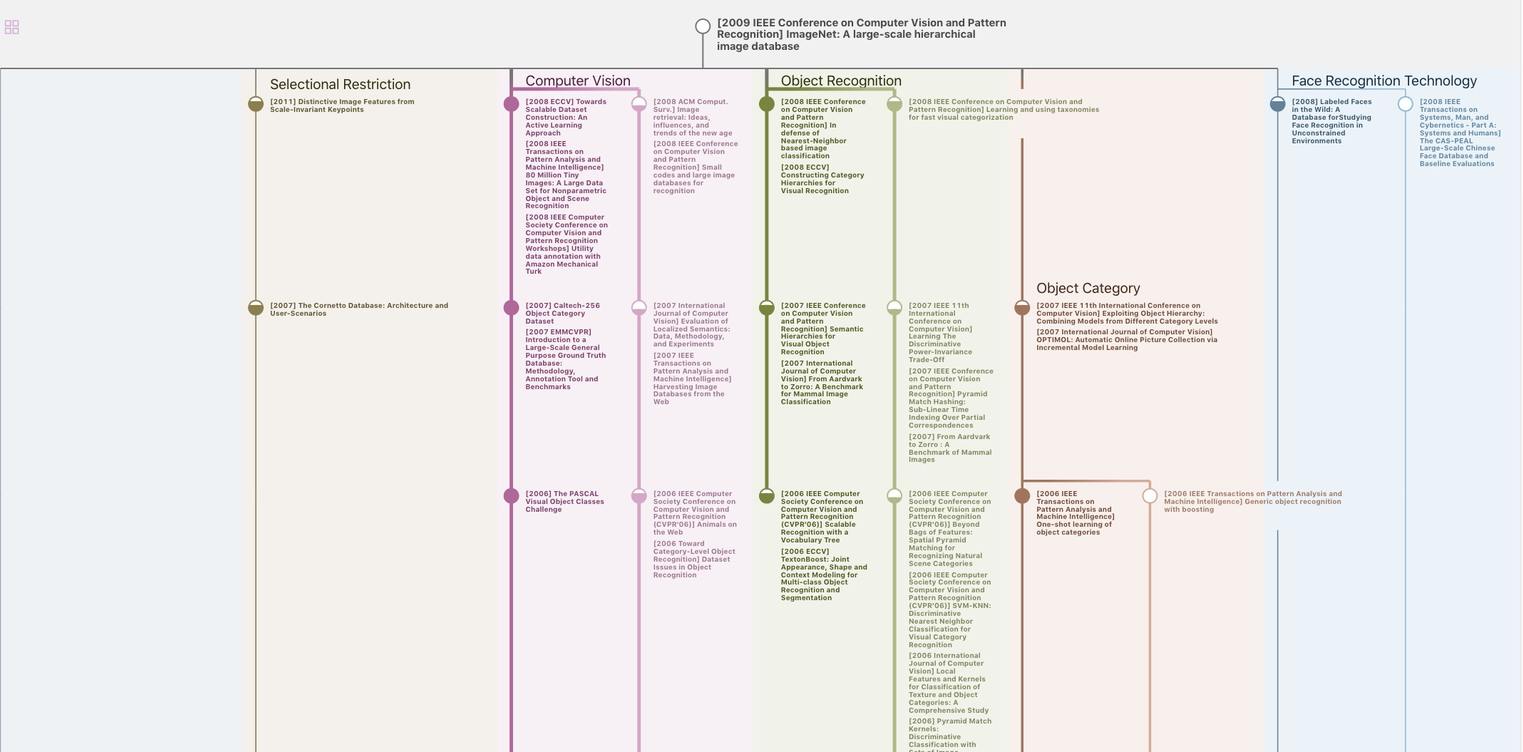
生成溯源树,研究论文发展脉络
Chat Paper
正在生成论文摘要