Abstract WP123: Machine Learning To Predict High Risk Adverse Events In Treatment Trials For Intracerebral Hemorrhage
Stroke(2023)
摘要
Background: ICH clinical trials have shown that aggressive blood pressure (BP) lowering can lead to adverse events (AEs) such as renal failure (RF) or new stroke. These studies focused on whether the study intervention causes AEs on average. By combining trials, we estimate each individual’s risk of developing AEs with BP lowering. Methods: We augmented data from ATACH2 by adding patients from the observational ERICH study and performed propensity score matching with a radius (radius=0.4, ratio=1). We estimated the individualized counterfactual outcome of renal AEs using augmented ATACH2. ERICH had 127 patients (out of 1,706) with new onset of RF during hospital stay. ATACH2 had 68 patients (out of 1000) with renal AEs (recorded by MedDRA) within 7d. We built the counterfactual prediction model using a neural network model (FlexTENet). We extracted feature importance score using Shap Deep Explainer. Results: We borrowed 1,706 patients to augment ATACH2. The augmented cohort had 2706 patients in total. FlexTENet captured the heterogeneity fairly. For factual outcome prediction accuracy, the FlexTENet achieved the best area under precision-recall curve (0.203) and the area under receiver operating curve (0.703). High diastolic BP at admission and low platelets increased the counterfactual effect of BP reduction on occurrence of renal AEs. In addition, high systolic BP and high glucose decreased the counterfactual effect on the occurrence of renal AEs. Conclusions: Identifying treatment-related AEs at an individual level has rarely been studied. Our counterfactual machine learning shows potential to predict individual patient’s risk of treatment-related adverse events.
更多查看译文
关键词
intracerebral hemorrhage,high risk adverse events,machine learning,treatment trials
AI 理解论文
溯源树
样例
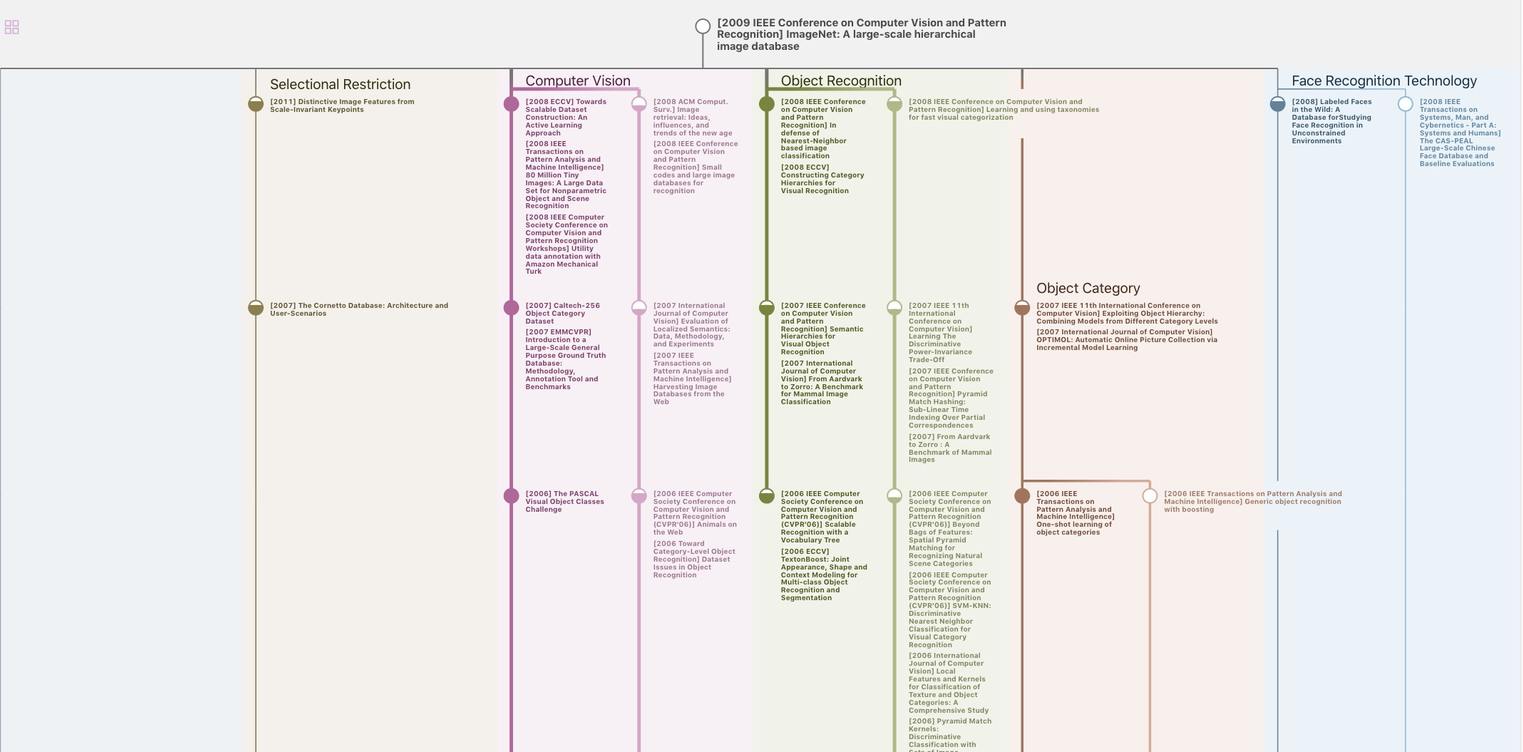
生成溯源树,研究论文发展脉络
Chat Paper
正在生成论文摘要