Defect detection network based on feature fusion and parallel cascade design
International Conference on Mechanisms and Robotics (ICMAR 2022)(2022)
摘要
At present, the defect detection link is indispensable in industrial production, and for the problem of low accuracy of existing neural networks in steel surface defect detection, a Faster-RCNN-based steel surface defect detection network (P-RCNN) is proposed in this paper. First, a new backbone network (Resnet-P2) is formed by adding feature fusion and attention mechanism to the original Faster-RCNN backbone network Resnet50, which can make the features transfer more effectively in the backbone network; second, a parallel cascade network is introduced, which aims to train a high-quality detector without reducing the number of high-quality samples. The purpose is to train a high quality detector without reducing the number of high quality samples, which makes the detection accuracy better. The experimental results show that the P-RCNN algorithm is used for steel surface defect detection with better detection performance and the accuracy is improved by 7.6% to 83.4% compared with Faster-RCNN, which can be used as a reference for steel surface defect identification applications.
更多查看译文
关键词
defect detection network,feature fusion,parallel cascade design
AI 理解论文
溯源树
样例
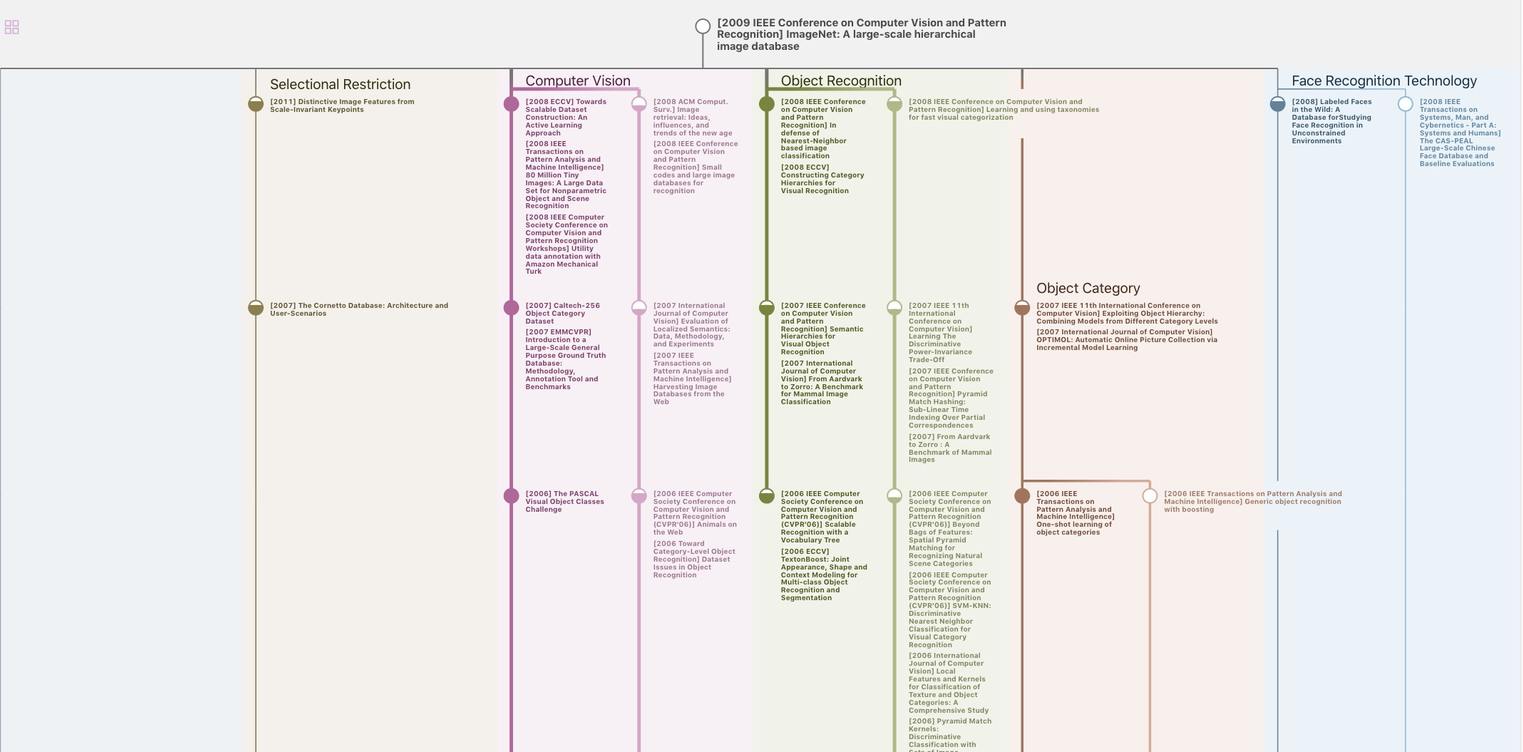
生成溯源树,研究论文发展脉络
Chat Paper
正在生成论文摘要