An Evolutionary Learning Approach Towards the Open Challenge of IoT Device Identification
Lecture Notes of the Institute for Computer Sciences, Social Informatics and Telecommunications EngineeringSecurity and Privacy in Communication Networks(2023)
摘要
Internet of Things (IoT) device identification has become an indispensable prerequisite for secure network management and security policy implementation. However, existing passive device identification methods work under a “closed-world” assumption, failing to take into account the emergence of new and unfamiliar devices in open scenarios. To combat the open-world challenge, we propose a novel evolutionary model which can continuously learn with new device traffic. Our model employs a decoupled architecture suitable for evolutionary learning, which consists of device feature representation and device inference. For device feature representation, an auto-encoder based on metric learning is innovatively introduced to mine latent feature representation of device traffic and form independent compact clusters for each device. For device inference, the nearest class mean (NCM) classification strategy is adopted on the feature representation. In addition, to alleviate the forgetting of old devices during evolutionary learning with new devices, we develop a less-forgetting constraint based on spatial knowledge distillation and impose control on the distribution distance between clusters to reduce inter-class interference. We evaluate our method on the union of three public IoT traffic datasets, in which the accuracy is as high as 87.9% after multi-stage evolutionary learning, outperforming all state-of-the-art methods under diverse experimental settings.
更多查看译文
关键词
evolutionary learning approach,iot,identification,open challenge,device
AI 理解论文
溯源树
样例
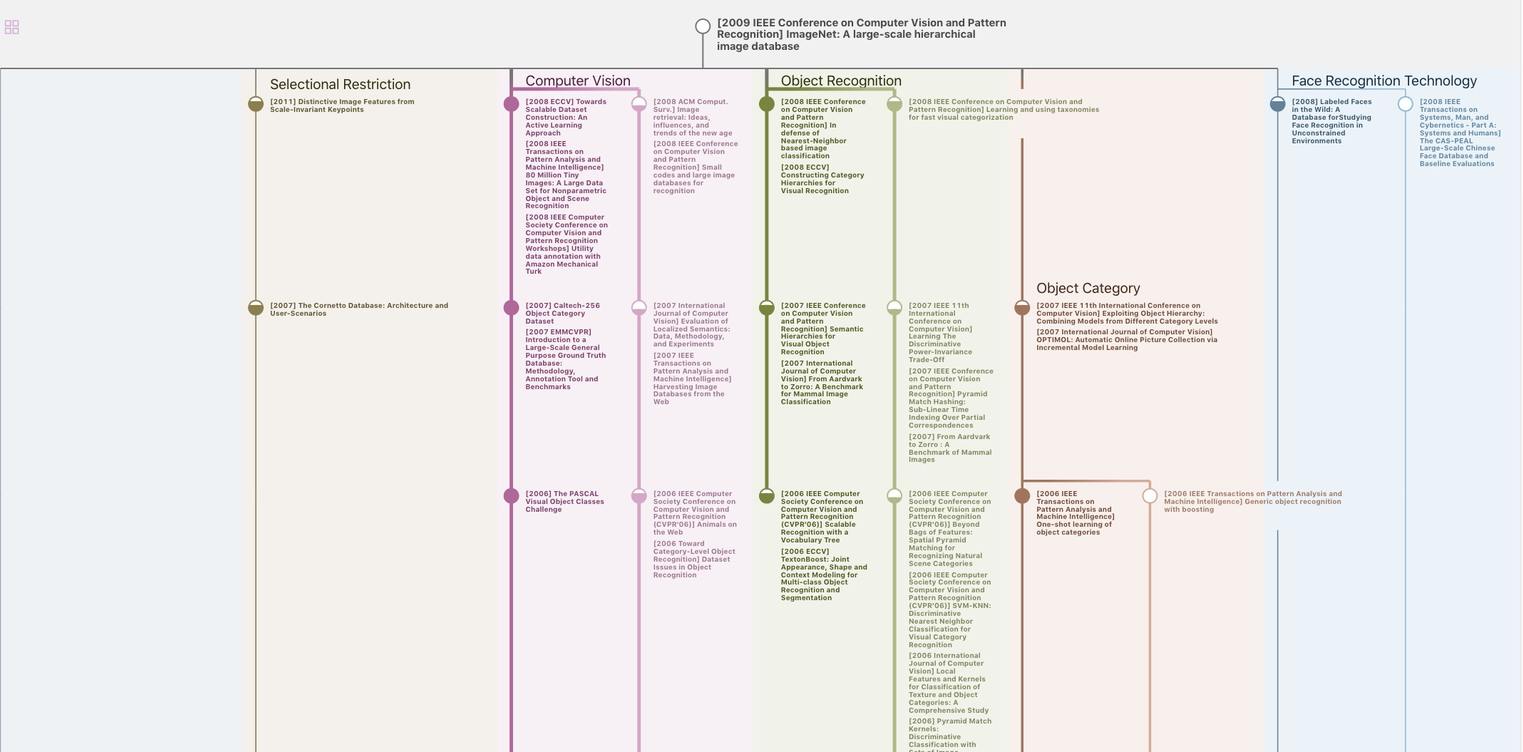
生成溯源树,研究论文发展脉络
Chat Paper
正在生成论文摘要