Generating Views Using Atmospheric Correction for Contrastive Self-supervised Learning of Multi-spectral Images
crossref(2022)
In remote sensing, plenty of multi-spectral images are publicly available from various landcover satellite missions. Since labeling remote sensing images is time-consuming, contrastive self-supervised learning is commonly applied to unlabeled data but relies on domain-specific transformations used for learning. When focusing on vegetation, the current contrastive learning frameworks cannot apply their transformations to the NIR channel, which carries valuable information about the vegetation state. Therefore, we use contrastive learning, relying on different views of unlabelled, multi-spectral images to obtain a pre-trained model to improve the accuracy scores on small-sized remote sensing datasets. This study presents the generation of additional views tailored to remote sensing images using atmospheric correction as an alternative transformation to color jittering. The proposed transformation can be easily integrated with multiple channels to exploit spectral signatures of objects. We found this proposed alternative transformation to improve performance compared to the baseline transformation.
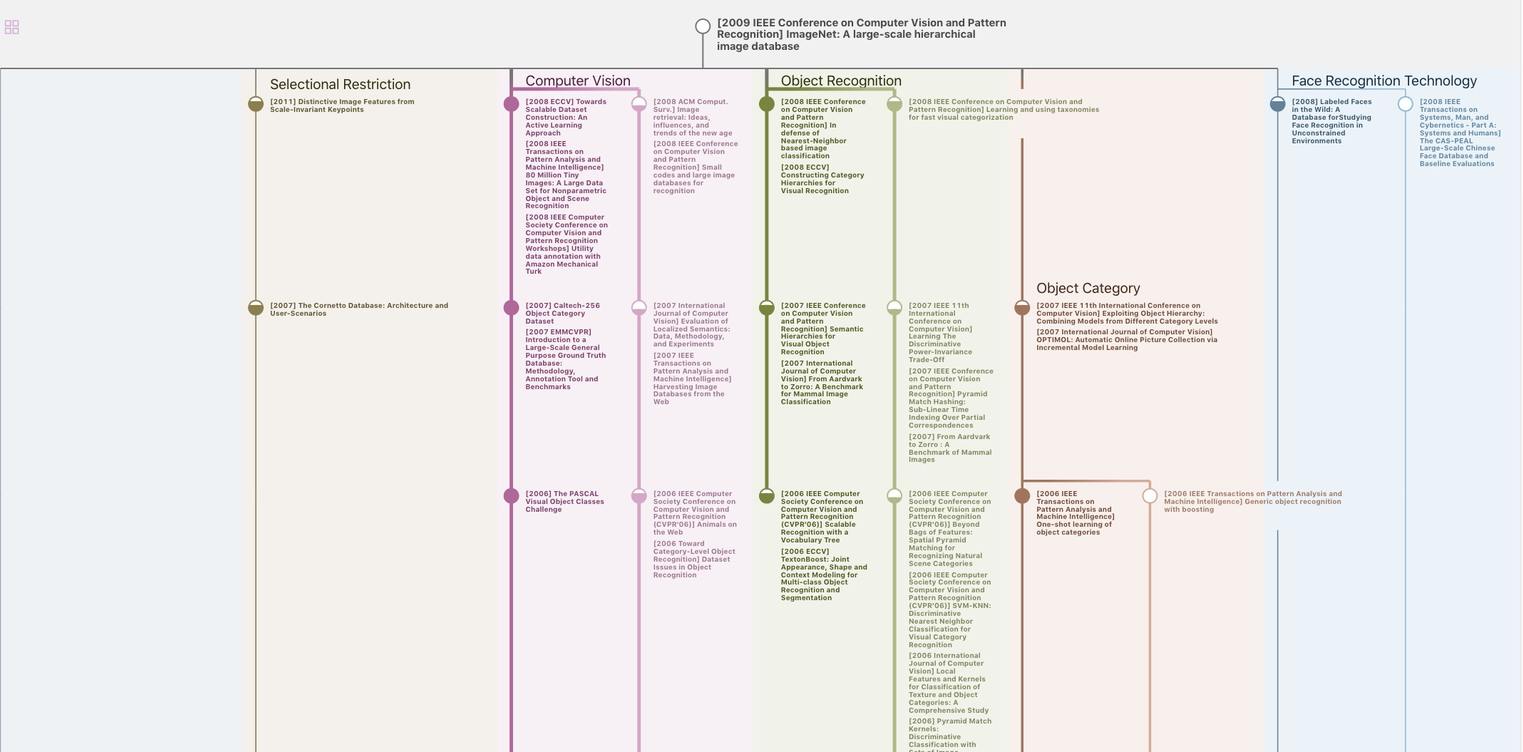