AttackMiner: A Graph Neural Network Based Approach for Attack Detection from Audit Logs
Lecture Notes of the Institute for Computer Sciences, Social Informatics and Telecommunications EngineeringSecurity and Privacy in Communication Networks(2023)
Abstract
In an enterprise environment, intrusion detection systems generate many threat alerts on anomalous events every day, and these alerts may involve certain steps of a long-dormant advanced persistent threat (APT). In this paper, we present AttackMiner, an attack detection framework that combines contextual information from audit logs. Our main observation is that the same attack behavior may occur in various possible contexts, and combining various possible contextual information can provide more effective information for detecting such attacks. We utilize a combination of provenance graph causal analysis and deep learning techniques to build a graph-structure-based model that builds key patterns of attack graphs and benign graphs from audit logs. During detection, the detection system creates provenance graphs using the input audit logs. After being optimized by our customized graph optimization mechanism, it identifies whether an attack has occurred. Our evaluations on the DARPA TC dataset show that AttackMiner can successfully detect attack behaviors with high accuracy and efficiency. Through this effort, we provide security investigators with a new approach of identifying attack activity from audit logs.
MoreTranslated text
Key words
attackminer detection,neural network
AI Read Science
Must-Reading Tree
Example
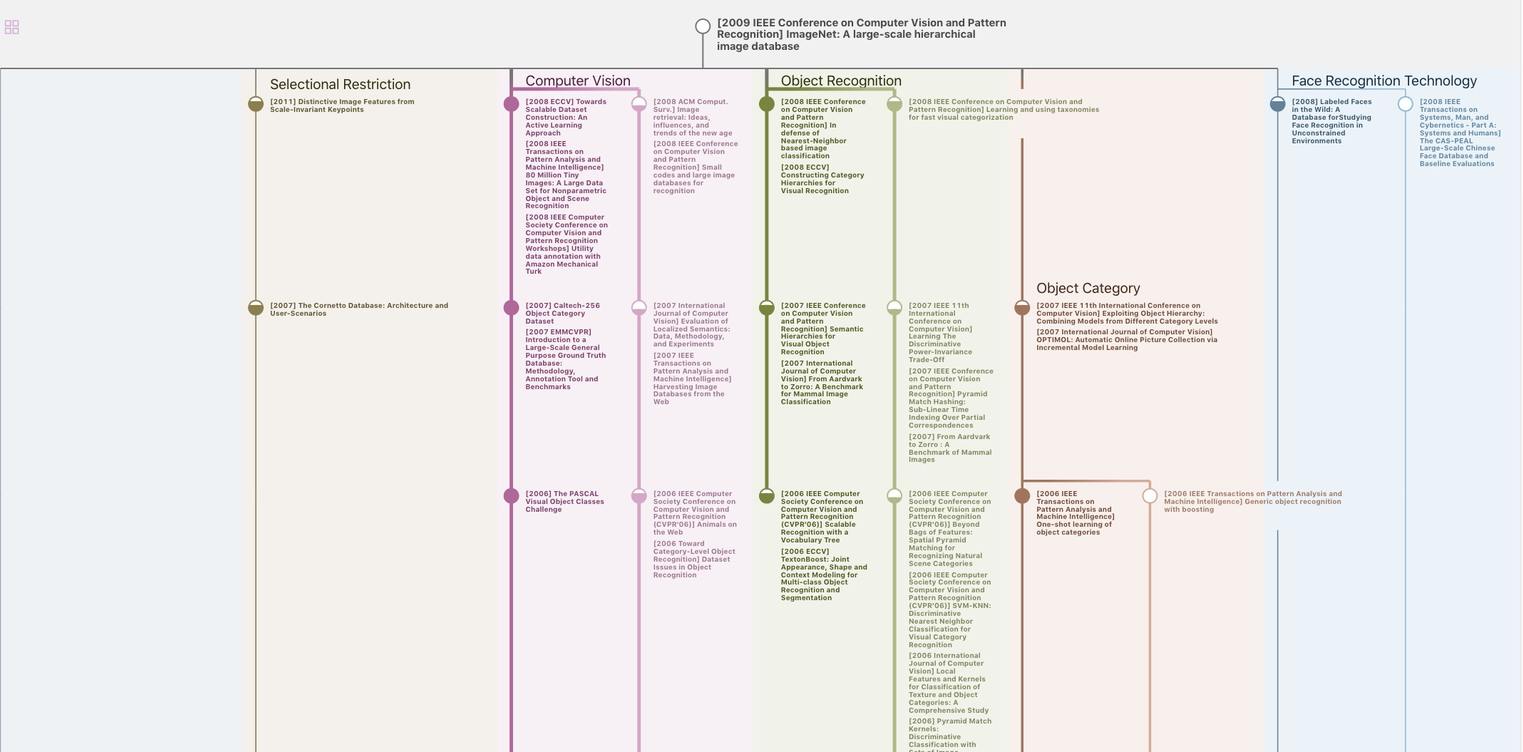
Generate MRT to find the research sequence of this paper
Chat Paper
Summary is being generated by the instructions you defined