Distributed Cognitive Learning Strategy for Cooperative-Competitive Multiagent Systems.
IEEE Transactions on Cognitive and Developmental Systems(2024)
摘要
In this paper, the distributed cognitive learning algorithm of cooperative-competitive multi-agent systems (MASs) on undirected graphs is studied, using parabolic equations as a model. Two cases are considered: without disturbance and with boundary disturbance. A bipartite consensus learning strategy is designed for the disturbance-free case, enabling agents to cognitively learn cooperative and adversarial information transmitted by their neighbors, thus reaching a synergistic consensus with other agents. For the case with boundary disturbance, an auxiliary system is first introduced and a disturbance observer is designed to estimate the disturbance. Then a cognitive learning algorithm is designed, in which agents constantly adjust their behavior by sensing the external environment to make complex behavioral decisions. Based on the proposed algorithms, the well-posedness of the closed-loop system is verified using semi-group theory, and the bipartite consensus of the MASs is discussed using Lyapunov’s direct method. Finally, the effectiveness of the proposed algorithms is verified using the finite difference method and Chebyshev spectral method.
更多查看译文
关键词
Bipartite consensus,multi-agent systems,cognitive learning,antagonistic information,parabolic PDEs
AI 理解论文
溯源树
样例
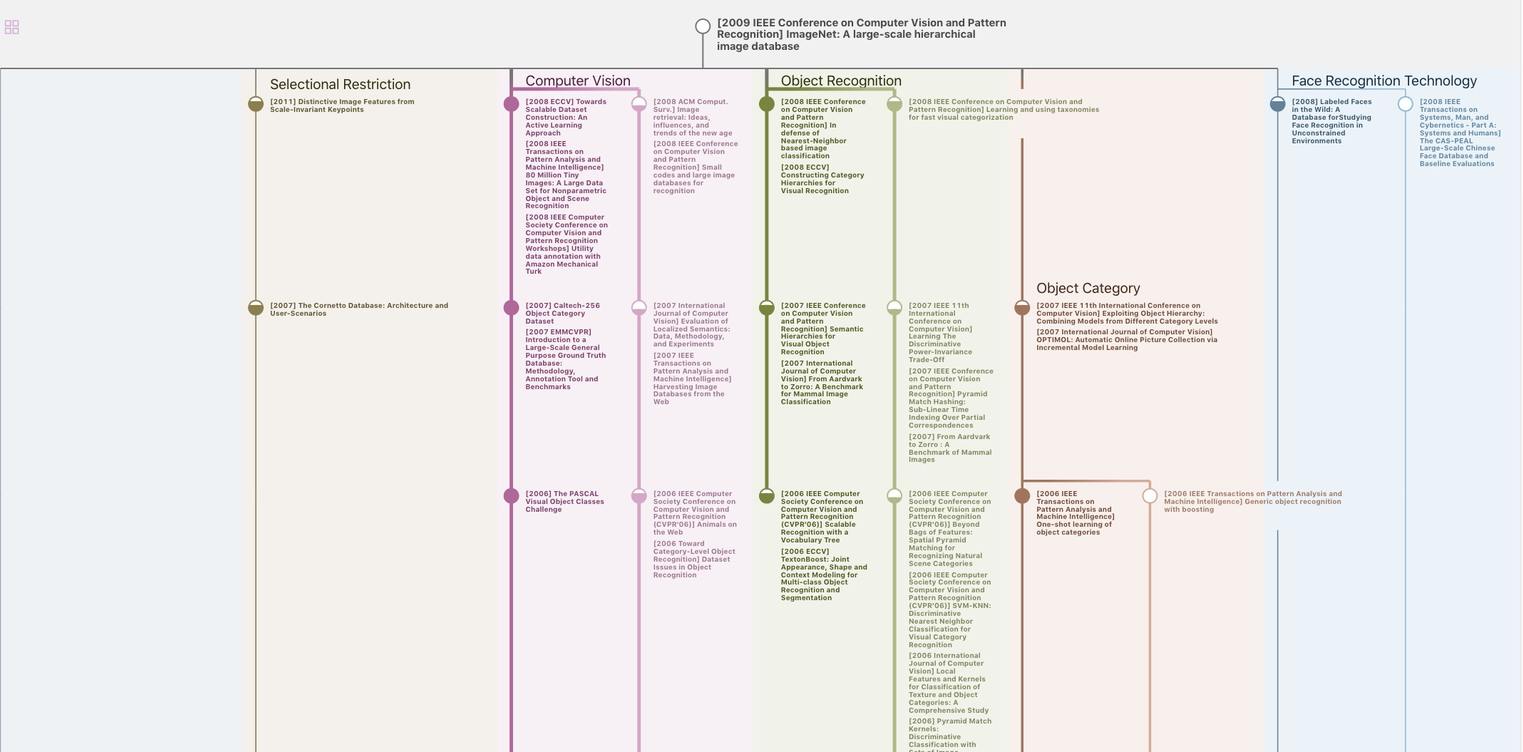
生成溯源树,研究论文发展脉络
Chat Paper
正在生成论文摘要