Imbalanced Clustering With Theoretical Learning Bounds
IEEE Transactions on Knowledge and Data Engineering(2023)
摘要
Imbalanced clustering, where the number of samples varies in different clusters, has arisen from many real data mining applications. It has gained increasing attention. Nevertheless, due to its unsupervised nature, imbalanced clustering is more challenging than its supervised counterpart, i.e., imbalanced classification. Furthermore, existing imbalanced clustering methods are empirically designed and they often lack solid theoretical guarantees, e.g., the excess risk estimation. To solve these important but rarely studied problems, we first propose a novel
$k$
-Means algorithm for imbalanced clustering problem with Adaptive Cluster Weight (MACW), together with its excess clustering risk bound analysis. Inspired by this theoretical result, we further propose an improved algorithm called Imbalanced Clustering with Theoretical Learning Bounds (ICTLB). It refines the weights and encourages the optimal trade-off among per-cluster weights by optimizing the excess clustering risk bound. A theoretically-principled justification of ICTLB is provided for verification. Comprehensive experiments on many imbalanced datasets verify the effectiveness of ICTLB in solving cluster imbalanced problems.
更多查看译文
关键词
Index Terms-Clustering, excess risk, imbalanced data, learning bound
AI 理解论文
溯源树
样例
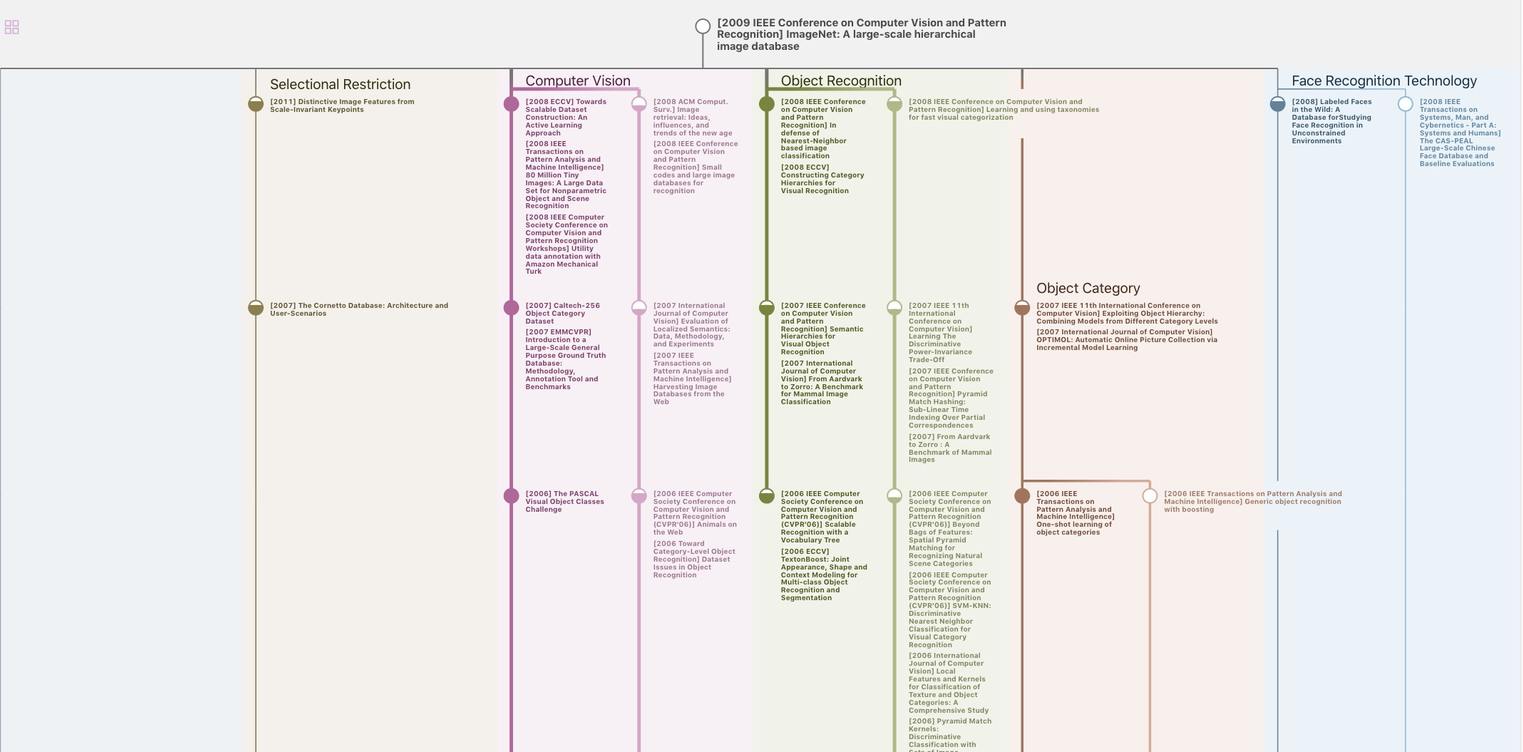
生成溯源树,研究论文发展脉络
Chat Paper
正在生成论文摘要