A Prognostics Approach Based on Feature Fusion and Deep BiLSTM Neural Network for Aero-Engine
2022 Global Reliability and Prognostics and Health Management (PHM-Yantai)(2022)
摘要
Aero-engines are prone to inaccurate remaining useful life prediction during operation due to the complexity of the degradation mechanism. Therefore, this paper proposes a multi-information fusion remaining useful lifetime prediction model based on simple moving average (SMA), principal component analysis (PCA) and deep bidirectional long short-term memory (DBiLSTM) neural network. Firstly, SMA is used for noise reduction of multi-dimensional degraded data of aero-engine, then PCA is used for dimensionality reduction and information fusion, finally DBiLSTM neural network model is used to predict the remaining useful life of engine for the above reduced data set, and eventually the mapping relationship between monitoring data and remaining life is obtained. This study uses the C-MAPSS aero-engine degradation dataset to experimentally validate the proposed multi-information fusion life prediction model and to compare it with other classical models and advanced methods that have emerged in recent years. The results show that the proposed method can accurately predict the remaining life under multi-dimensional degradation information, and the error and score of the prediction model are reduced up to 10.28% and 31.65% for RMSE and Score, respectively, compared with other model methods.
更多查看译文
关键词
prognostics approach,deep bilstm neural network,feature fusion,aero-engine
AI 理解论文
溯源树
样例
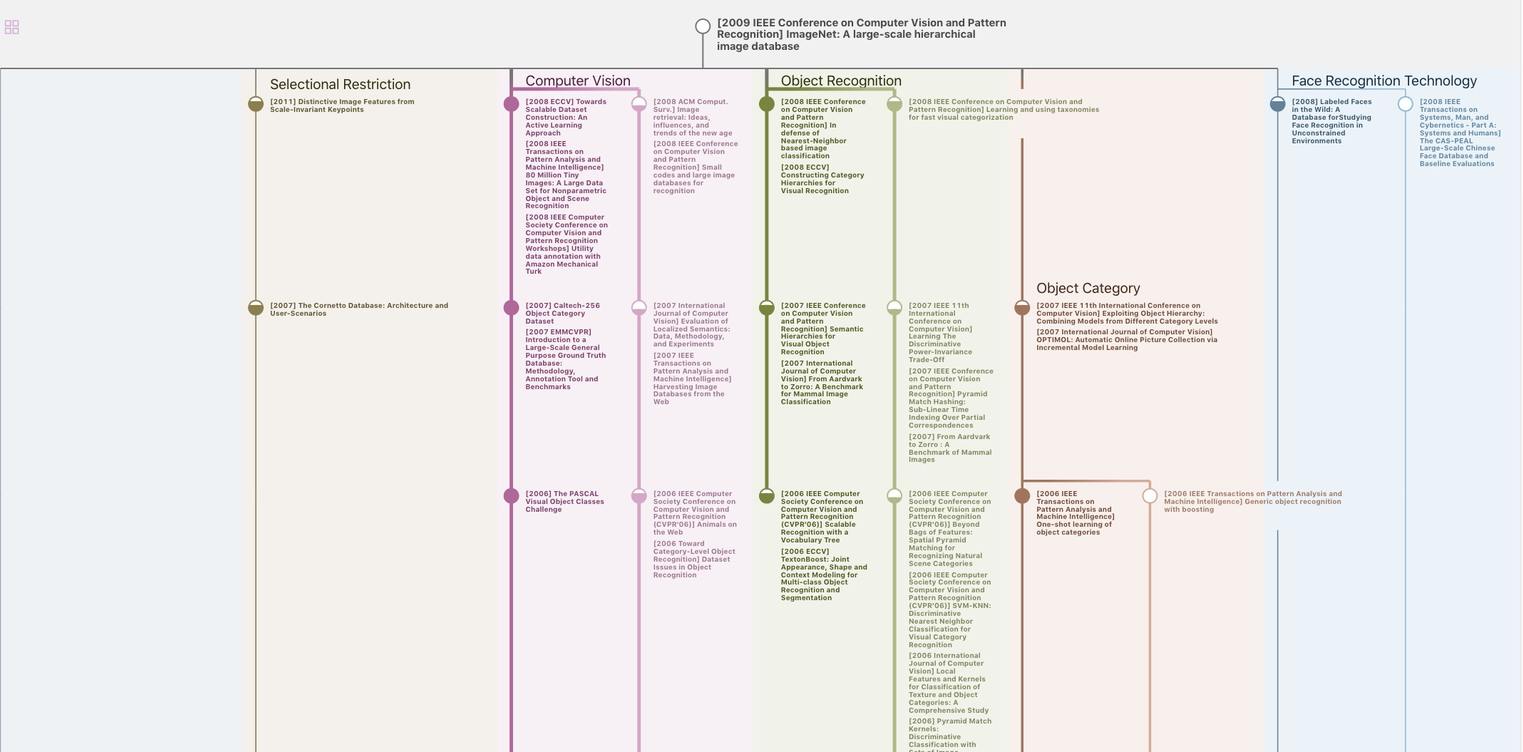
生成溯源树,研究论文发展脉络
Chat Paper
正在生成论文摘要