Multi-objective optimization of IoT-based green building energy system using binary metaheuristic algorithms
Journal of Building Engineering(2023)
摘要
Green building energy optimization systems (GBEOS) are of great interest to academics and in-dustry professionals. One of the main challenges of GBEOS is optimizing energy consumption while maintaining people's comfort level. This paper proposes a metaheuristic-based GBEOS for optimizing energy consumption within a smart green building operating under a smart grid paradigm while considering the local environment and energy demand behaviors. In this regard, two complementary control procedures are developed: the supply-part optimizer (SPO) and the demand-part optimizer (DPO). By combining SPO and DPO, six binary multi-objective optimi-zation algorithms, including Xtreme gradient boosting (XGBoost), multiverse optimizer (MVO), moth flame optimization (MFO), shuffled frog leaping algorithm (SFLA), moth flame optimiza-tion, imperialist competitive algorithm (ICA), and chimp optimization algorithm (ChOA) are developed to simultaneously minimizes costs and maximizes client comfort satisfaction (CS). First of all, considering the importance of convergence speed, seven unimodal, six multimodal, and twenty complex benchmark optimization functions are used to evaluate the proposed approach's convergence behavior. Afterward, an efficient weighting mechanism is used to handle the cost -comfort trade-off, allowing the user to discover a reasonable trade-off between monetary expense and comfort level. Then, in order to determine the influence of the pricing scheme's nature on the potential savings achieved through EMS, three individual models named real-time pricing (RTP), time-of-utilize pricing (TUP), and fixed-pricing plan (FPP) are proposed. The re-sults show that ChOA presents the best result in terms of convergence, with 19 out of 33 test functions; also, XGBoost has the second-best performance. In addition, results show that sug-gested metaheuristic algorithms significantly increase PV energy penetration for self-consumption and lower power costs while keeping an optimal balance between CS and economical costs. Moreover, using dynamic pricing systems, i.e., RTP or TUP, results in great user price savings than FPP. To sum up, ChOA is found to be the best algorithm in this challenge, followed by XGBoost in second place.
更多查看译文
关键词
Metaheuristic algorithm,Multi-objective optimization,Internet of things,Green buildings
AI 理解论文
溯源树
样例
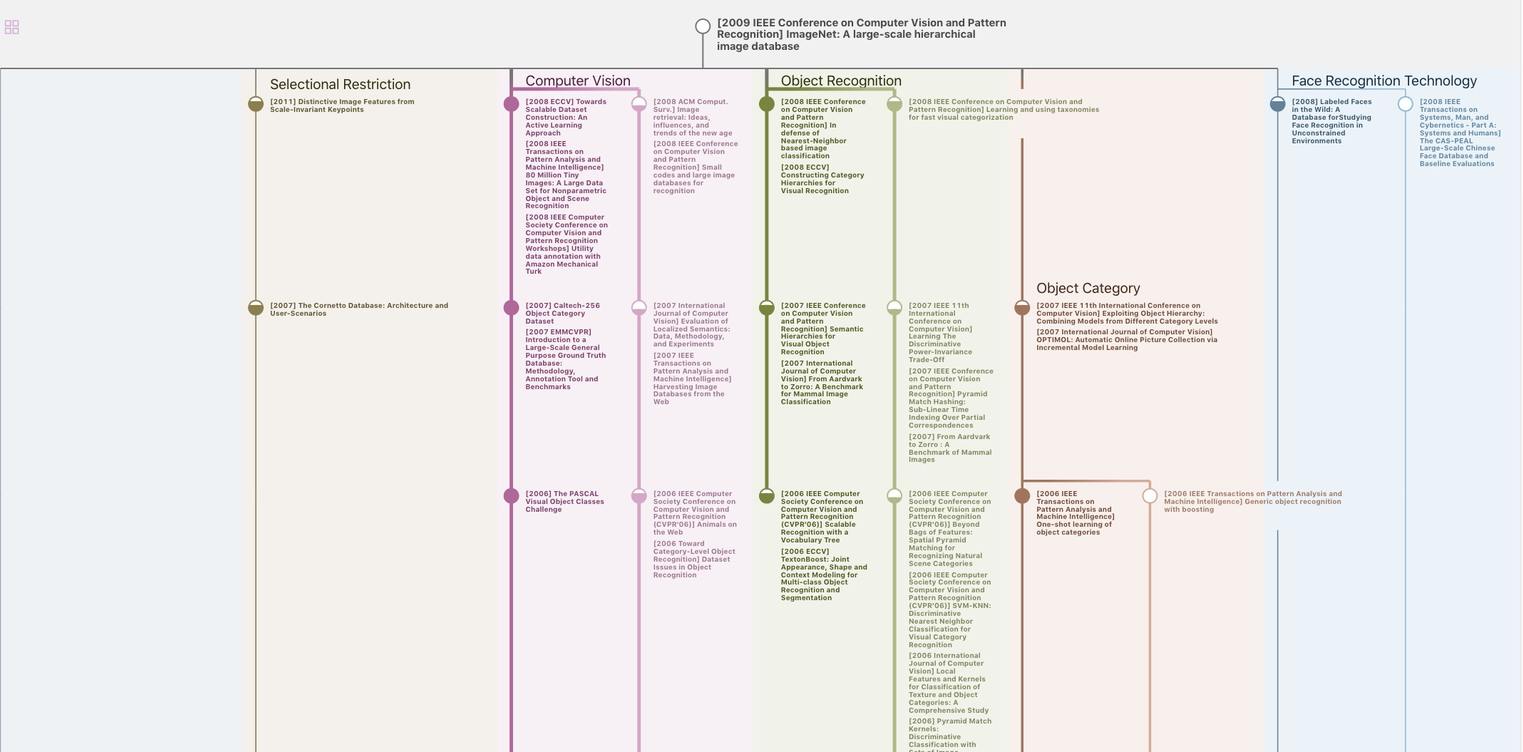
生成溯源树,研究论文发展脉络
Chat Paper
正在生成论文摘要