Spatiotemporal Residual Graph Attention Network for Traffic Flow Forecasting
IEEE Internet of Things Journal(2023)
摘要
Accurate spatiotemporal traffic flow forecasting is significant for the modern traffic management and control. In order to capture the spatiotemporal characteristics of the traffic flow simultaneously, we propose a novel spatiotemporal residual graph attention network (STRGAT). First, the network adopts a deep full residual graph attention block, which performs a dynamic aggregation of spatial features regarding the node information of the traffic network. Second, a sequence-to-sequence block is designed to capture the temporal dependence in the traffic flow. The traffic flow data with weekly periodic dependencies are also integrated and STRGAT is used for traffic forecasting of traffic road networks. The experiments are conducted on three real data sets in California, USA. Results verify that our proposed STRGAT is able to learn the spatiotemporal correlation of traffic flow well and outperforms the state-of-the-art methods.
更多查看译文
关键词
traffic flow forecasting,graph,attention
AI 理解论文
溯源树
样例
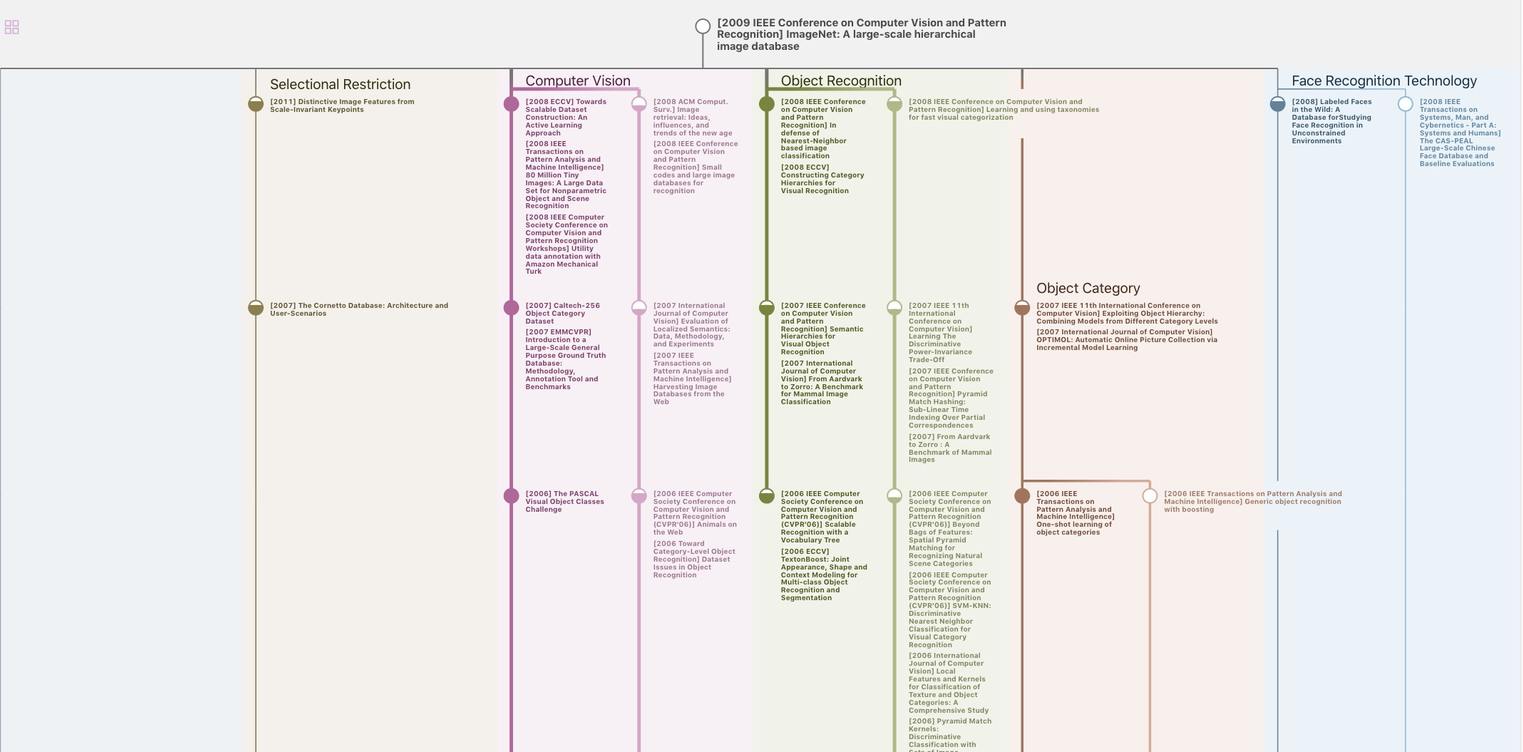
生成溯源树,研究论文发展脉络
Chat Paper
正在生成论文摘要