Comparative evaluation of geometrical, Zernike moments, and volumetric features of the corpus callosum for discrimination of ASD using machine learning algorithms
International Journal of Biomedical Engineering and Technology(2022)
摘要
In this study, we compared the performance of geometrical, Zernike moments, and volumetric features of the corpus callosum (CC) to diagnose autism spectrum disorder (ASD). Initially, the CC was segmented from the midsagittal view of 2D structural magnetic resonance images using the distance regularised level set evolution (DRLSE). The segmented images were validated with the ground truth using similarity measures. The geometrical and Zernike moments were extracted from the 2D segmented region, and the volumetric features were extracted from 3D images of CC. The features extracted were then used to train classifiers. The segmented images were highly matched with the ground truth with mean similarity measure values of Sokal and Sneath-II = 0.9928 and Pearson and Heron-II = 0.9924. We achieved the highest site-specific classification accuracy of 72.69% using the random forest (RF) classifier. The pipeline followed in this study can be used for mass screening of ASD-like neurodevelopmental disorders.
更多查看译文
关键词
corpus callosum,zernike moments,asd,machine learning algorithms
AI 理解论文
溯源树
样例
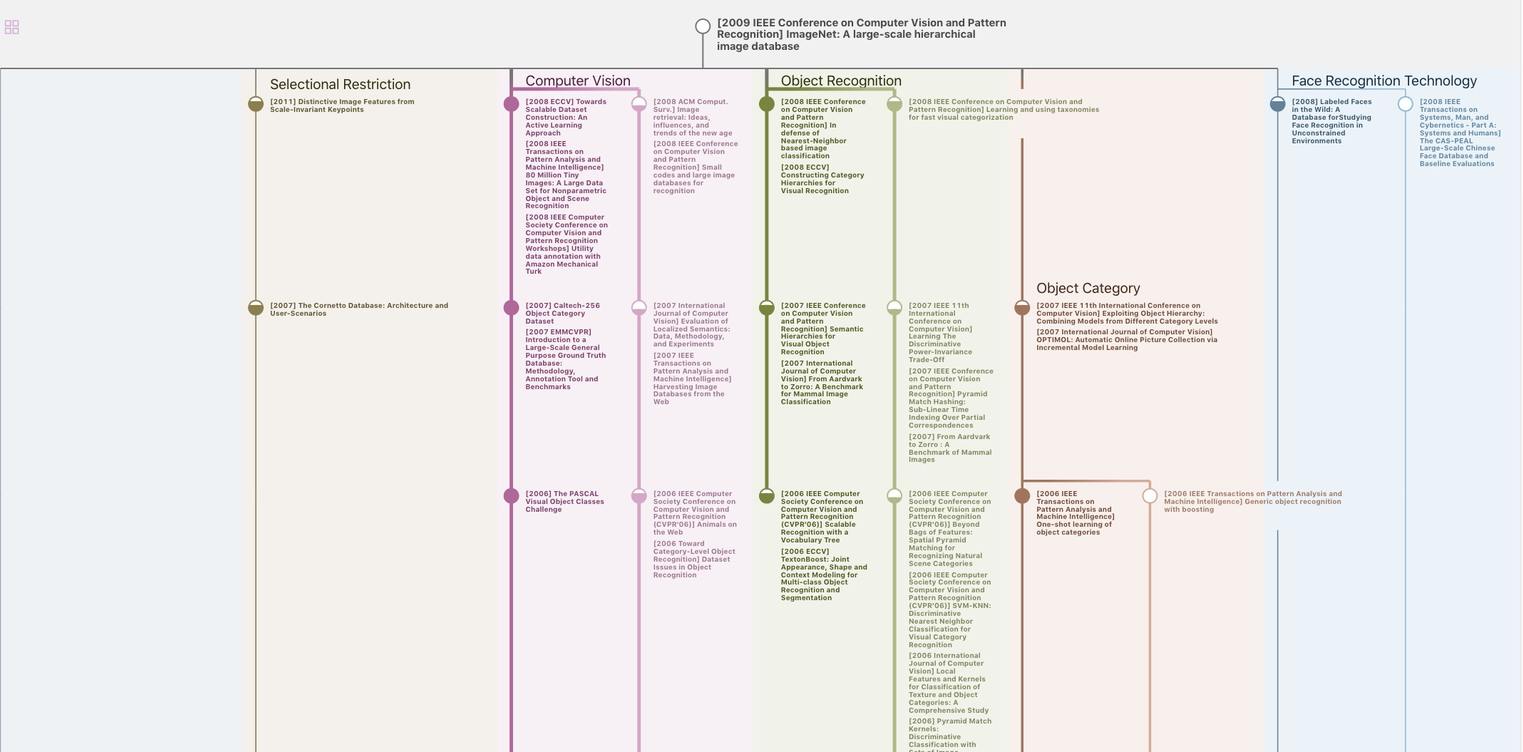
生成溯源树,研究论文发展脉络
Chat Paper
正在生成论文摘要