Multi-Objective Optimization of Local Overlapping Community Detection: A Formal Model and Novel Evolutionary Algorithm
IEEE Transactions on Network Science and Engineering(2023)
摘要
Local overlapping community detection (LOCD), which aims to discover the multiple communities containing a given starting node, becomes a hot research problem in community detection area. However, existing LOCD methods only consider the quality of each local community containing the starting node, while ignoring the information between the multiple local communities. To this end, in this paper, we first formulate a novel multi-objective model to better assess the quality of local overlapping communities, where two novel objectives are suggested by fully utilizing the information within and between the multiple local communities. Afterward, we propose a tri-division representation-based multi-objective evolutionary algorithm (TDR-MOEA) for effectively finding multiple local overlapping communities for a given node under the formulated multi-objective model. In TDR-MOEA, a tri-division individual representation scheme is designed to effectively encode and decode the local overlapping communities. Based on the representation, two novel crossover and mutation operators are then suggested. In addition, an effective population initialization strategy is designed to obtain an initial population with better diversity and convergence. The effectiveness of the proposed multi-objective model and TDR-MOEA is verified on several synthetic networks and real-world networks. The experimental results show that TDR-MOEA is superior to state-of-the-art algorithms for solving LOCD.
更多查看译文
关键词
Heuristic algorithms, Optimization, Linear programming, Clustering algorithms, Detection algorithms, Social networking (online), Evolutionary computation, Community detection, individual representation, local overlapping community detection, multi-objective evolutionary algorithm (MOEA)
AI 理解论文
溯源树
样例
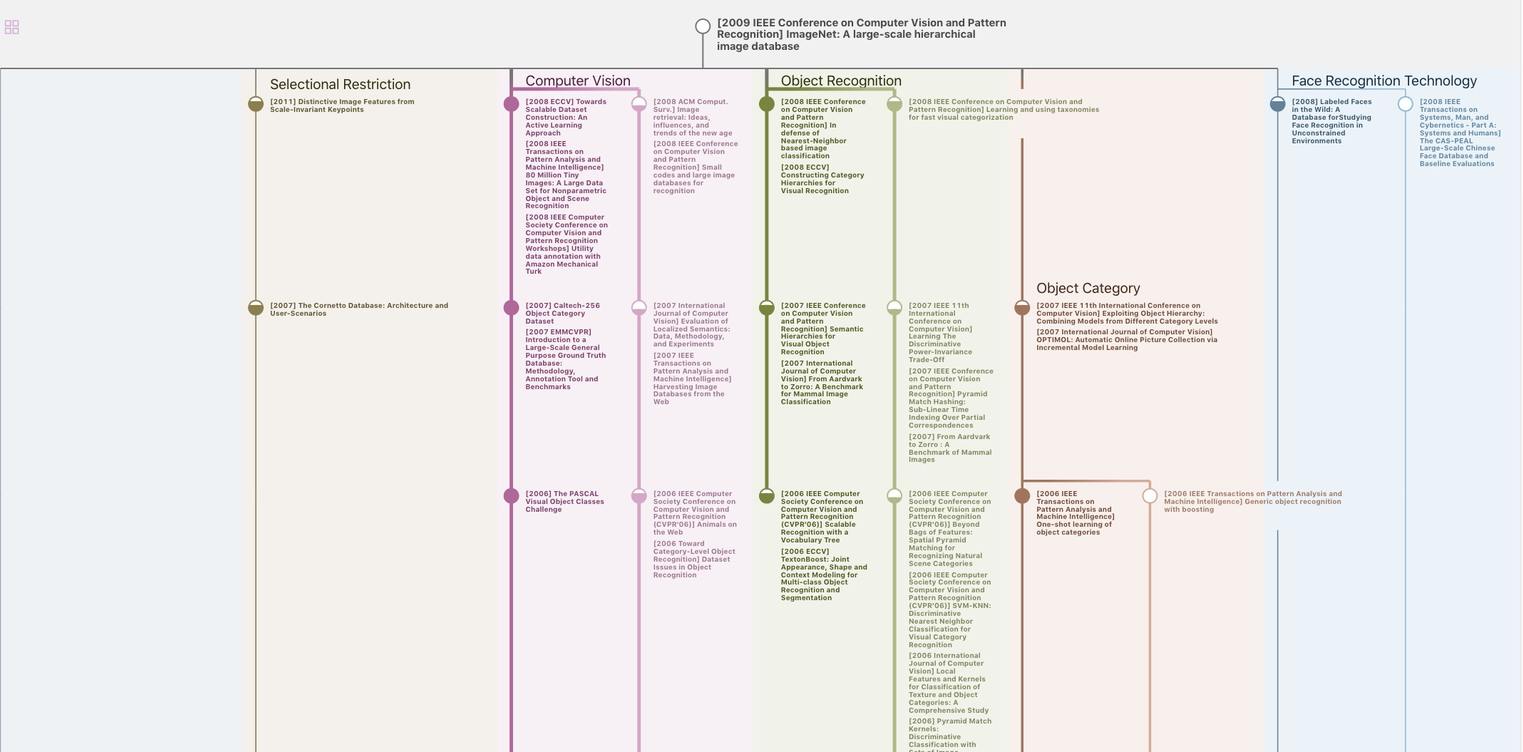
生成溯源树,研究论文发展脉络
Chat Paper
正在生成论文摘要