RAHG: A Role-Aware Hypergraph Neural Network for Node Classification in Graphs
IEEE Transactions on Network Science and Engineering(2023)
摘要
Graph neural networks have been widely studied and applied to node classification in graph-format datasets in recent years. Traditional graph neural networks mainly consider the adjacency characteristics of nodes and fail to learn rich role representations of nodes. Existing role representations methods of nodes are mostly in unsupervised approaches, resulting in unsatisfactory performance in downstream tasks. A graph can be reorganized as a hypergraph, in which the role characteristics of nodes are more intuitively represented. Based on this, we propose a role-aware hypergraph neural network (RAHG) that utilizes hypergraphs and an attention mechanism to fuse nodes' role and adjacency representations. A residual network is also applied to relieve the smoothing problem between layers in the model. The model adjusts the weights on the role and adjacency representations according to the characteristics of the graphs. RAHG significantly improves the prediction performance compared with existing graph neural networks on seven datasets, with accuracy increased by up to 12.1% on the node classification task in graphs.
更多查看译文
关键词
Graph neural networks,graph representation,node classification,hypergraph
AI 理解论文
溯源树
样例
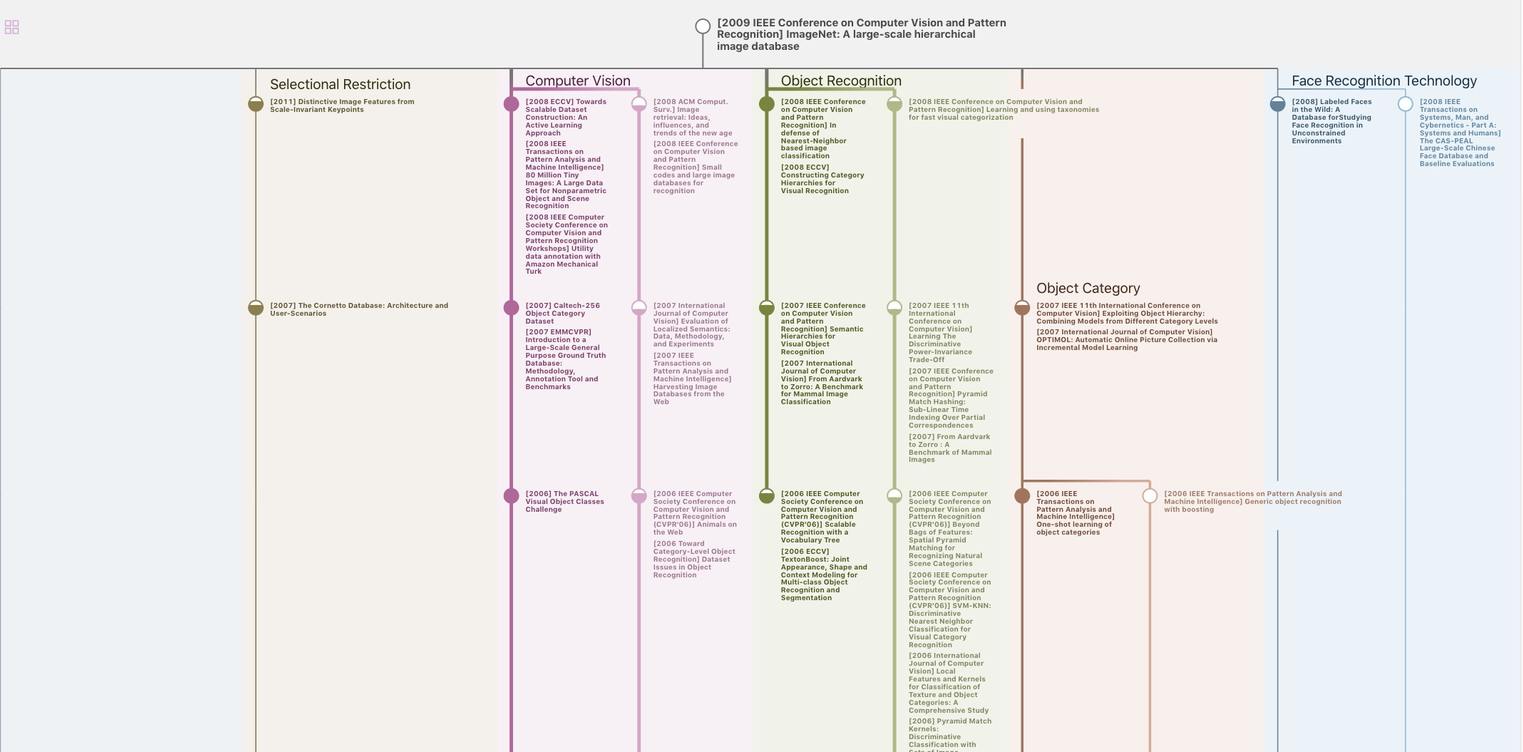
生成溯源树,研究论文发展脉络
Chat Paper
正在生成论文摘要