Exploring aesthetic procedural noise for crafting model-agnostic universal adversarial perturbations
SSRN Electronic Journal(2023)
摘要
The research on universal adversarial perturbations (UAPs) is significant to trustworthy deep learning. To disentangle the UAPs with the training data dependency and the target model dependency, the exploration of procedural noise functions is a feasible method. However, the current procedural adversarial noise attack method has several characteristics like visually significant anisotropy and gradient artifacts that may impact the stealthiness of adversarial examples. This study proposes a novel model-free and data-free UAP method based on the procedural noise functions with two variants: Simplex noise attack and Worley noise attack. The attack method can achieve deceit on the neural networks with a more aesthetic rendering effect. A detailed empirical study is provided to validate the effectiveness of the proposed attack method. The extensive experiments show that the UAPs generated by the proposed method achieve considerable attack performance on the ImageNet dataset and the CIFAR-10 dataset. Moreover, this study implements the performance evaluation and robustness analysis of existing defense methods against the proposed UAPs. It has the potential to enhance research on the robustness of neural networks in real applications. The code is available at https: //github.com/momo1986/adversarial_example_simplex_worley.
更多查看译文
关键词
Adversarial examples,Universal adversarial perturbations,Security,Computer vision,Deep learning,Robustness
AI 理解论文
溯源树
样例
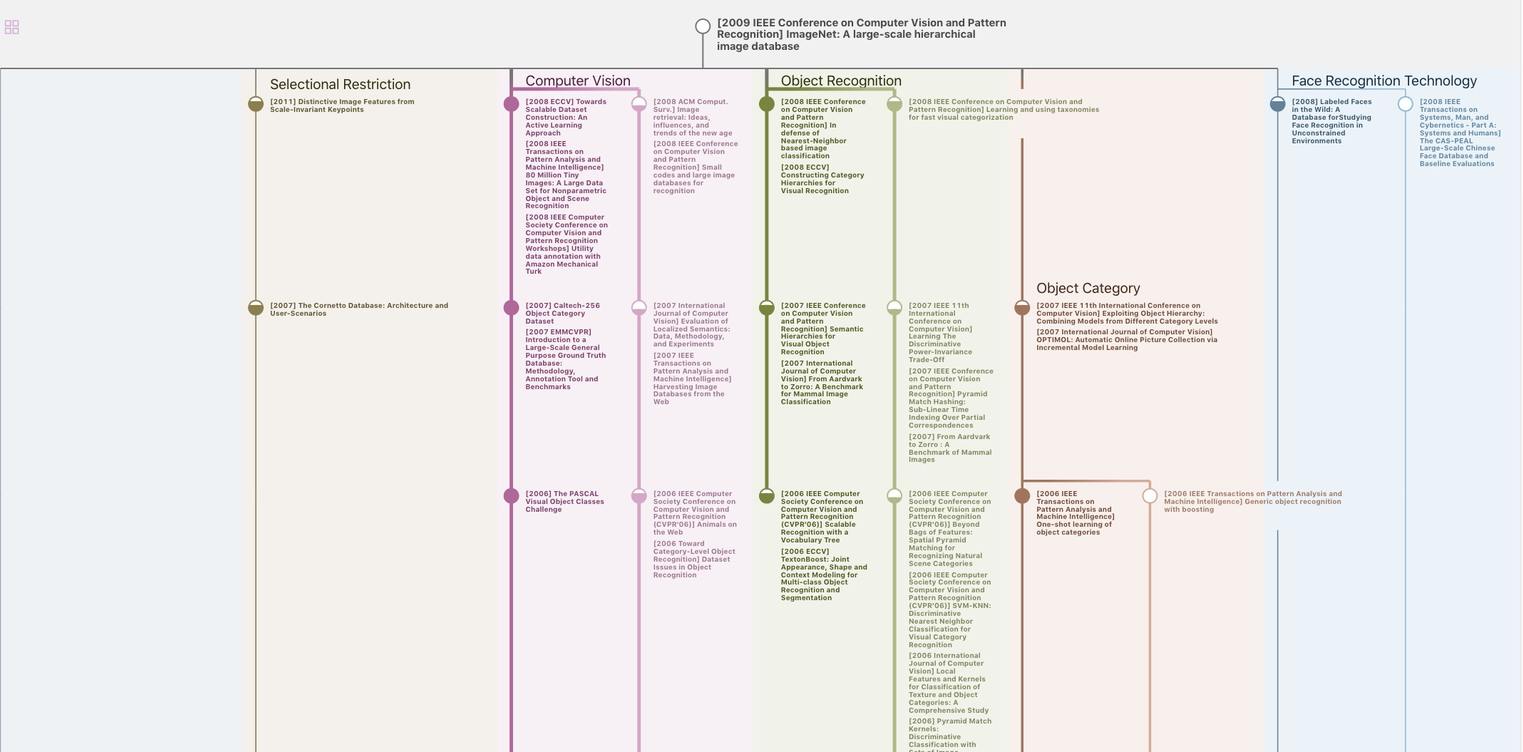
生成溯源树,研究论文发展脉络
Chat Paper
正在生成论文摘要