Multiscale patch-based feature graphs for image classification
EXPERT SYSTEMS WITH APPLICATIONS(2024)
摘要
Deep learning architectures have demonstrated outstanding results in image classification in the last few years. However, applying sophisticated neural network architectures in small datasets remains challenging. In this context, transfer learning is a promising approach for dealing with this scenario. Generally, the available pre-trained architectures adopt a standard fixed input, which usually implies resizing and cropping the input images in the preprocessing phase, causing information loss. Besides, images present visual features in different scales in real-world scenarios, and most common approaches do not consider this fact. In this paper, we propose an approach that applies transfer learning for dealing with small datasets and leverages visual features extracted by pre-trained models from different scales. We based our approach on graph convolutional networks (GCN) that take graphs representing the images in different scales as input and whose nodes are characterized by features extracted by pre-trained models from regular image patches of different scales. Since GCN can deal with graphs with different numbers of nodes, our approach can deal naturally with images of heterogeneous sizes without discarding relevant information. We evaluated our approach in two datasets: a set of geological images and a publicly available dataset, both presenting characteristics that challenge traditional approaches. We tested our approach by adopting three different pre-trained models as feature extractors: two efficient pre-trained CNN models (DenseNet and ResNeXt) and one Vision Transformer (CLIP). We compared our approach with two conventional approaches for dealing with image classification. The experiments show that our approach achieves better results than the conventional approaches for this task.
更多查看译文
关键词
Image classification,Transfer learning,Feature extraction,Multiscale
AI 理解论文
溯源树
样例
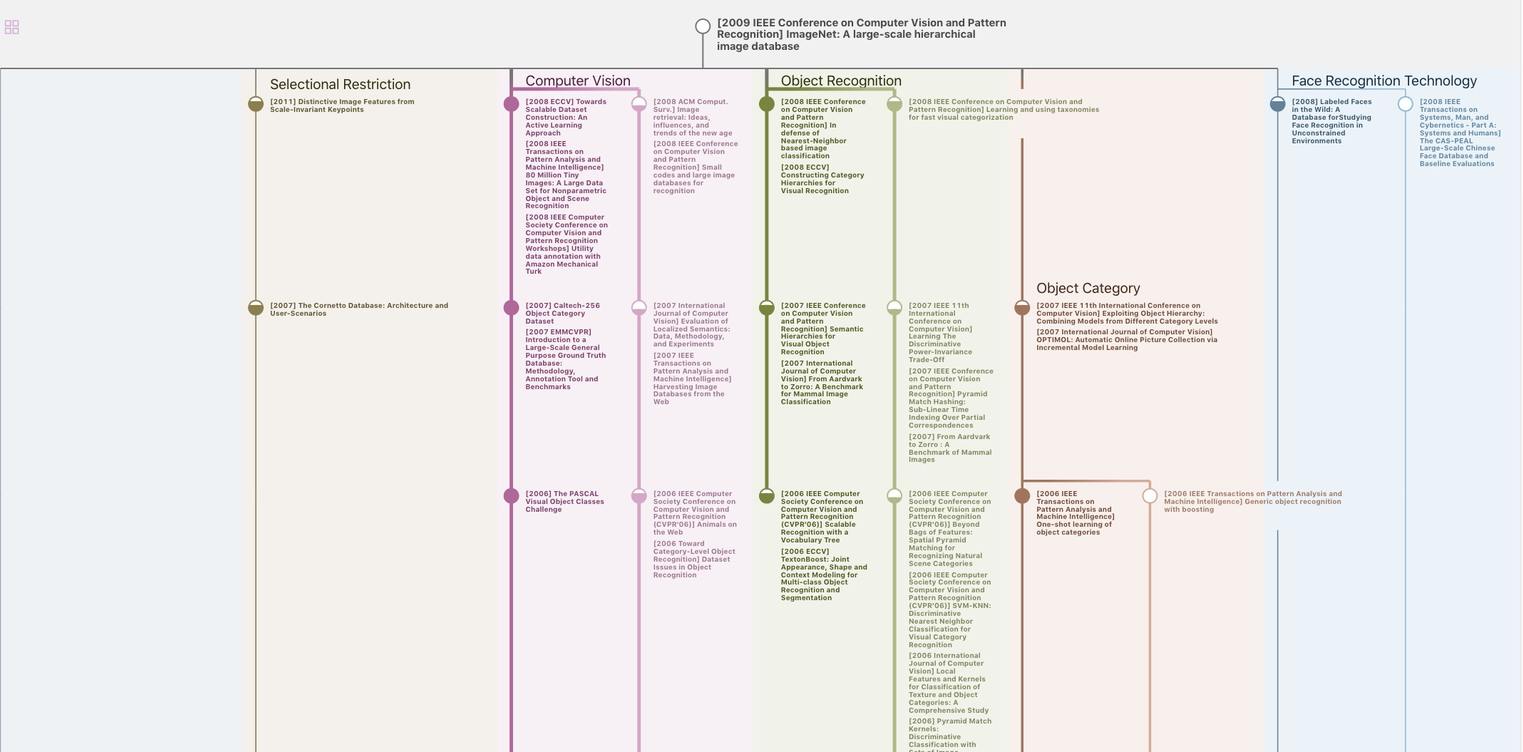
生成溯源树,研究论文发展脉络
Chat Paper
正在生成论文摘要