Prediction of Erythemato-Squamous Diseases Using Machine Learning
Applications of Machine Learning and Deep Learning on Biological Data(2023)
摘要
In the field of dermatology, the “Differential diagnosis of Erythemato-Squamous Diseases (ESD)” is a major challenge. The ESD consists of six different classes i.e., “psoriasis, seborrheic dermatitis, lichen planus, pityriasis rosea, chronic dermatitis and pityriasis rubra pilaris”. The condition frequently causes skin redness (also known as erythema), due to reduction and loss of squamous cells of skin. The most common reasons are genetic or environmental influences. The condition is especially common throughout certain stages of life, like in early adolescence or in late childhood. The problem is seen as a challenging one in the field of dermatology as one disease type may initially exhibit traits of another before developing its own distinct characteristics. The problem becomes more difficult as they share many histopathological and clinical features. In order to perform the disease diagnosis, usually clinical and histopathological checks are performed on patients. Clinical testing use non-invasive assessment of symptoms such as size, position, and presence of color, pustules, and other features. In order to discover the likely viral origins, histopathological studies necessitate the extraction of skin samples, i.e., biopsies. Such an approach is very much time consuming, expensive, and difficult for dermatologists to detect the disease easily. According to the literature, the “differential diagnosis of ESDs” is widely explored as a data science and Machine Learning (ML) topic. ML in healthcare and biological sciences is becoming more widely used nowadays. In this chapter, we propose to implement different ML classifiers to detect a particular case of ESD. The comparative analysis of different classifiers shall be carried out using performance metrics namely, Accuracy, Sensitivity and Specificity. The dataset for the problem is freely available at “UCI Machine Learning repository maintained by Center for Machine Learning and Intelligent Systems at the University of California, Irvine”. The dataset retrieved consists of 34 characteristics of which 33 are linearly valued and one holds a nominal value. Except age and family history features, rest of the features are divided into histopathological and clinical categories. Before model training, the data pre-processing or feature engineering shall be carried out. In the end to check consistency of the model, cross validation shall be carried out.
更多查看译文
关键词
machine learning,diseases,prediction,erythemato-squamous
AI 理解论文
溯源树
样例
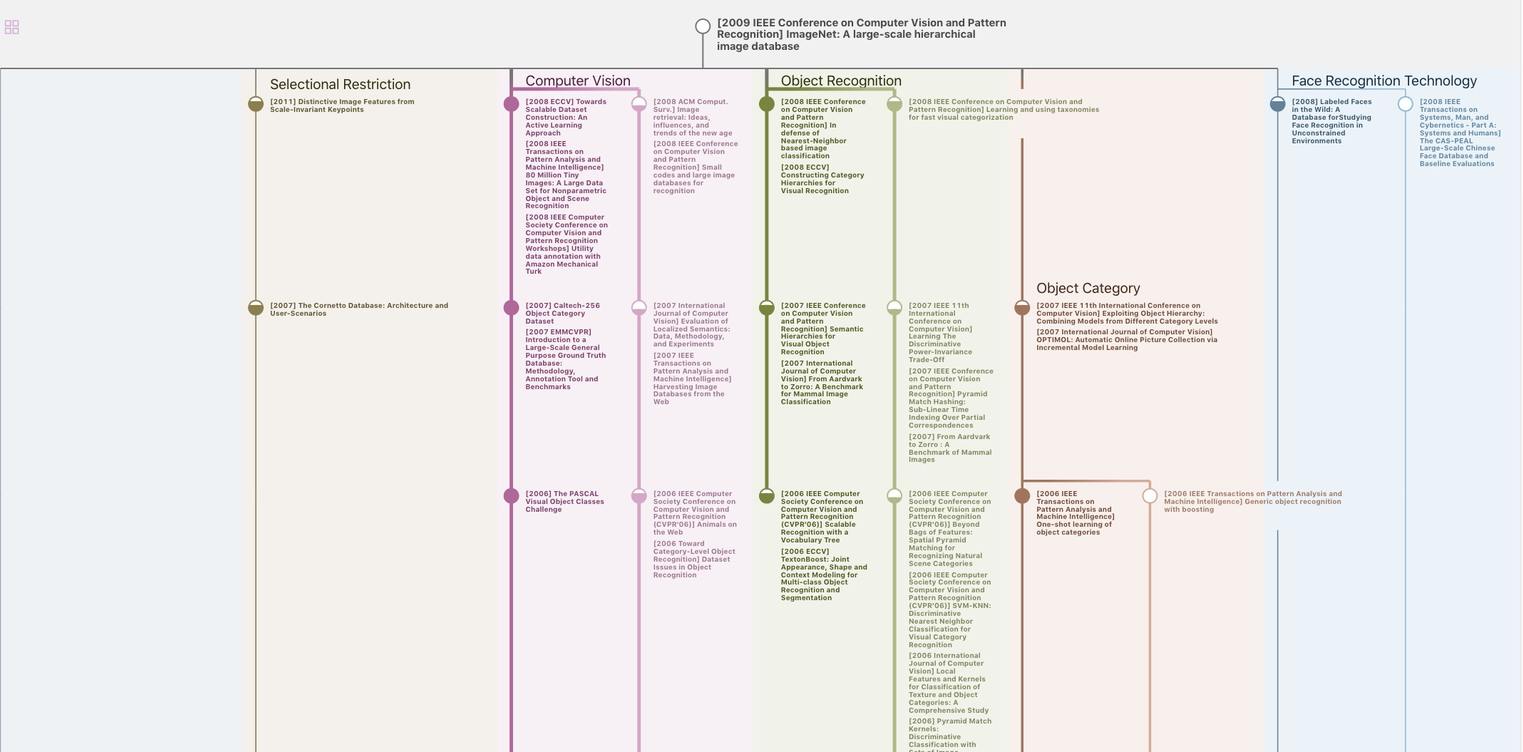
生成溯源树,研究论文发展脉络
Chat Paper
正在生成论文摘要