Weakly supervised segmentation for plant disease using class activation maps and weak annotation labels
International Conference on Geographic Information and Remote Sensing Technology (GIRST 2022)(2023)
摘要
The segmentation of crop disease is an important task for plant image processing. Fully supervised segmentation methods are commonly used to segment disease on plant leaves, and a large amount of manually annotated information is needed. In contrast, weakly supervised segmentation methods have the advantages of reducing the workload of manual annotation. In this study, a weakly supervised segmentation method for plant disease was proposed for the segmentation of rust in apple rust images using the procedure below. A classification model was trained using images of both diseased and healthy leaves, and class activation maps (CAMs) of disease were obtained as pseudo labels for a further segmentation of the disease. The segmentation method using CAMs only achieved an Intersection over Union (IoU) score of 0.45. Then point-auxiliary labels and line-auxiliary labels of disease were also constructed to improve the accuracy of the disease segmentation. The IoU values resulting from the two schemes – the point-auxiliary labels + CAMs and line-auxiliary labels + CAMs methods reached 0.64 and 0.74, respectively. Moreover, the proposed method was also applied to the segmentation of disease on whole leaves. Furthermore, the proportion of disease in whole leaves can be also obtained, and the coefficient of the correlation between the obtained disease proportion and true disease proportion was 0.89. The results suggest the feasibility of the proposed method to segment plant disease and evaluate the severity degree of the plant disease.
更多查看译文
关键词
segmentation,class activation maps,plant disease,weakly
AI 理解论文
溯源树
样例
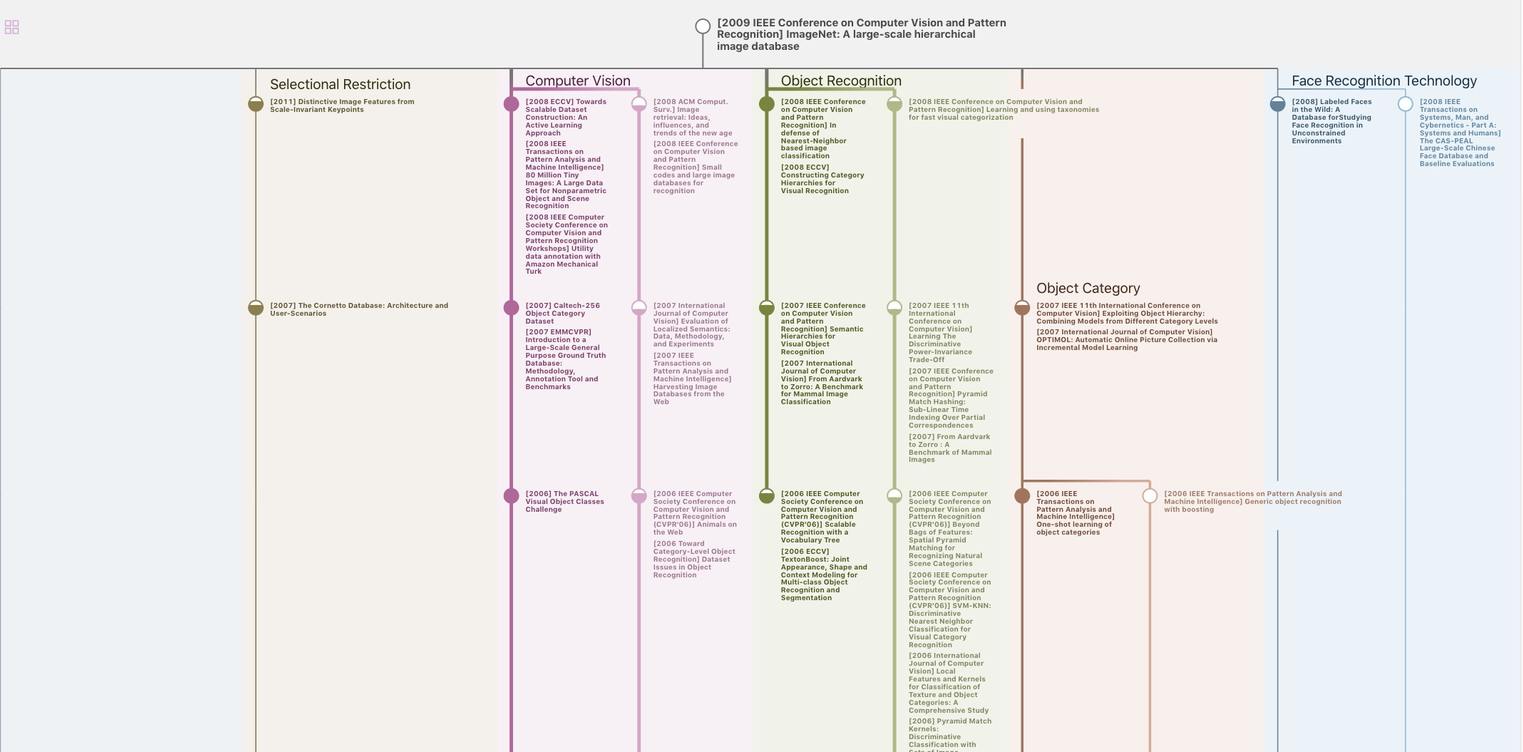
生成溯源树,研究论文发展脉络
Chat Paper
正在生成论文摘要