On the Performance of Bayesian Generalized Dissimilarity Model Estimator
Mathematics and Statistics(2023)
摘要
The Generalized Dissimilarity Model (GDM) is an extension of Generalized Linear Model (GLM) that is used to describe and estimate biological pairwise dissimilarities following a binomial process in response to environmental gradients. Some improvement has been made to accommodate the uncertainty quantity of GDM by applying resampling scheme such as Bayesian Bootstrap (BBGDM). Because there is an ecological assumption in the GDM, it is reasonable to use a proper Bayesian approach rather than resampling method to obtain better modelling and inference results. Similar to other GLM techniques, the GDM also employs a link function, such as the logit link function that is commonly used for the binomial regression model. By using this link, a Bayesian approach to GDM framework which called Bayesian GDM (BGDM) can be constructed. In this paper, we aim to evaluate the estimators' performance of Bayesian Generalized Dissimilarity Model (BGDM) in relative to BBGDM. Our study revealed that the performance of BGDM estimator outperformed that of BBGDM, especially in term of unbiasedness and efficiency. However, the BGDM estimators failed to meet consistency property. Moreover, the application of the BGDM to a real case study indicates that its inferential abilities are superior to the preceding model.
更多查看译文
关键词
model
AI 理解论文
溯源树
样例
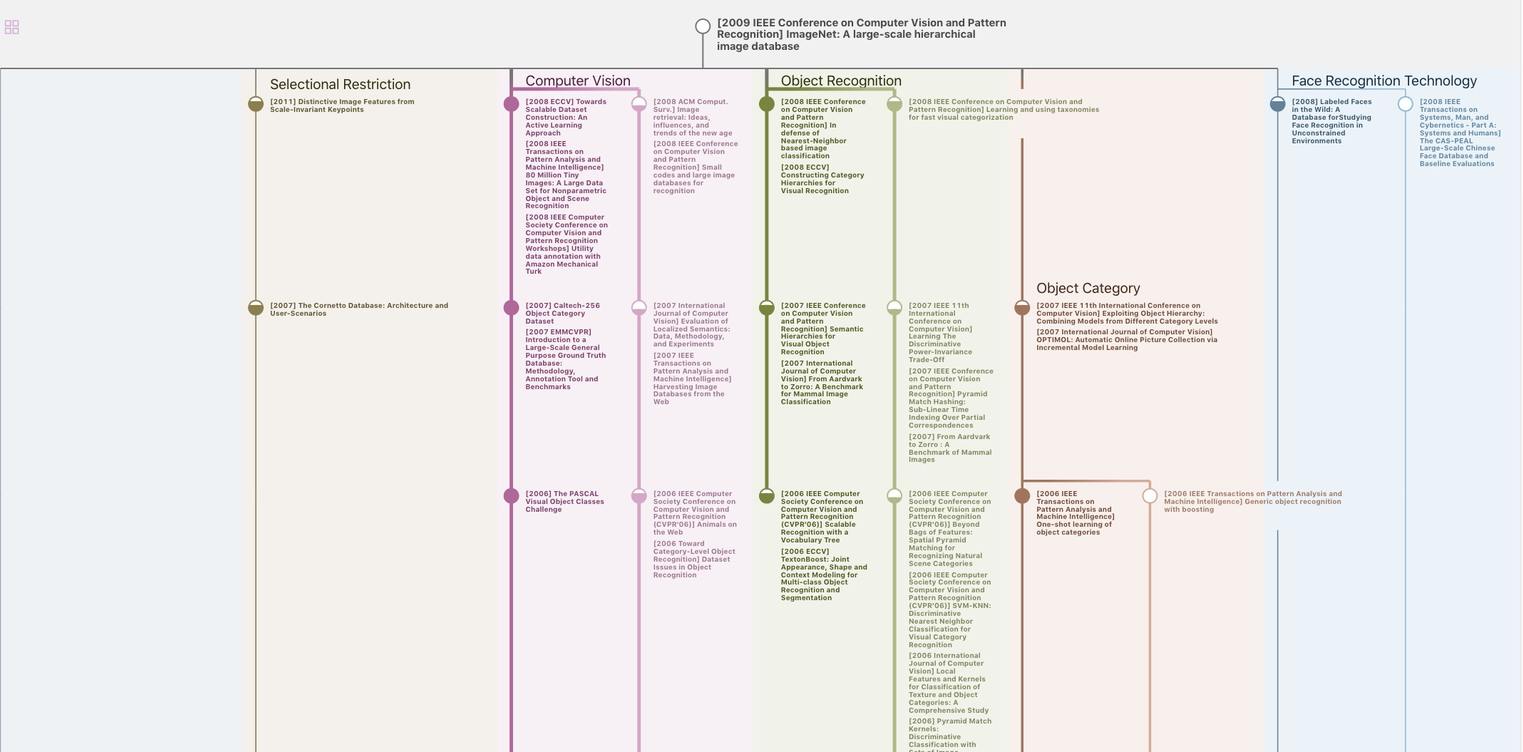
生成溯源树,研究论文发展脉络
Chat Paper
正在生成论文摘要