Noise Resilient Learning for Attack Detection in Smart Grid PMU Infrastructure.
IEEE Trans. Dependable Secur. Comput.(2024)
摘要
Falsified data from compromised Phasor Measurement Units (PMUs) in a smart grid induce Energy Management Systems (EMS) to have an inaccurate estimation of the state of the grid, disrupting various operations of the power grid. Moreover, the PMUs deployed at the distribution layer of a smart grid show dynamic fluctuations in their data streams, which make it extremely challenging to design effective learning frameworks for anomaly based attack detection. In this paper, we propose a noise resilient learning framework for anomaly based attack detection specifically for distribution layer PMU infrastructure, that show real time indicators of data falsifications attacks while offsetting the effect of false alarms caused by the noise. Specifically, we propose a feature extraction framework that uses some Pythagorean Means of the active power from a cluster of PMUs, reducing multi-dimensional nature of the PMU data streams via quick Big Data summarization. We also propose a robust and noise resilient methodology for learning thresholds based on generalized robust estimation theory of our invariant feature. We experimentally validate our approach and demonstrate improved reliability performance using two completely different datasets collected from real distribution level PMU infrastructures.
更多查看译文
关键词
Anomaly Detection,Big Data Management,Learning based PMU Security,Smart Grid Security
AI 理解论文
溯源树
样例
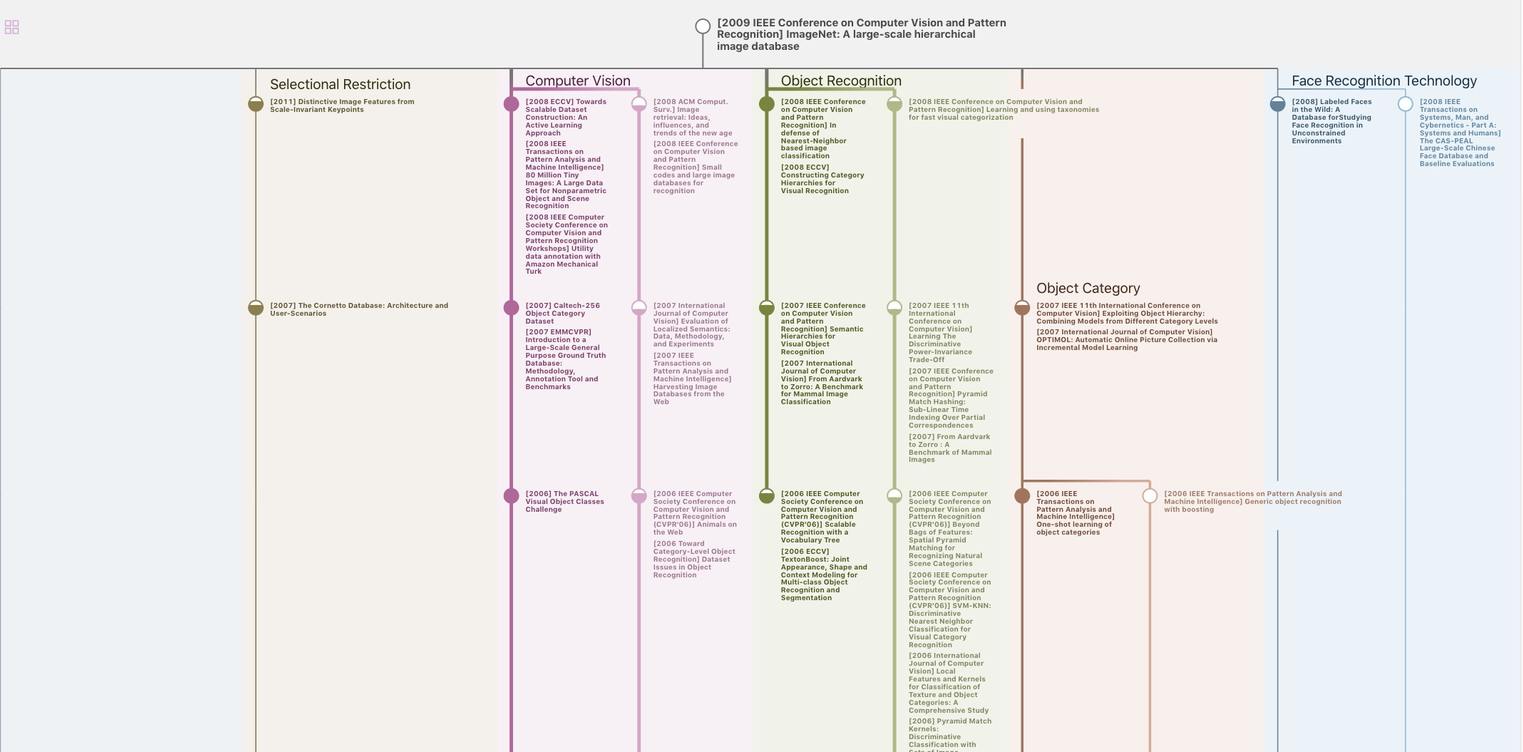
生成溯源树,研究论文发展脉络
Chat Paper
正在生成论文摘要