Surrogate-Assisted Environmental Selection for Fast Hypervolume-Based Many-Objective Optimization
IEEE TRANSACTIONS ON EVOLUTIONARY COMPUTATION(2024)
摘要
Hypervolume (HV)-based evolutionary algorithms have been widely used to handle many-objective optimization problems. In such algorithms, HV-based environmental selection (HVES), which aims at selecting a subpopulation with the maximal HV from the current population, plays a crucial role in guiding evolution. However, the computation time of HV increases exponentially with the number of objectives, making the HVES task an expensive optimization problem. In this article, we propose an efficient surrogate-assisted greedy inclusion algorithm to deal with computationally expensive HVES tasks. It uses a lightweight surrogate model, radial basis function network, to replace the most time-consuming calculations. In addition, an L-1 -norm distance-based filter is performed as a preselection operator to reduce the search space and avoid some unnecessary calculations. Considering the inevitable approximation errors of surrogate models, we also design an online sampling strategy to enhance the reliability of selected solutions. The proposed algorithm is tested on two types of datasets and compared with six state-of-the-art greedy algorithms. Experimental results show that the proposed algorithm performs excellently on most datasets.
更多查看译文
关键词
Environmental selection,hypervolume (HV) approximation,many-objective optimization,surrogate-assisted optimization algorithms
AI 理解论文
溯源树
样例
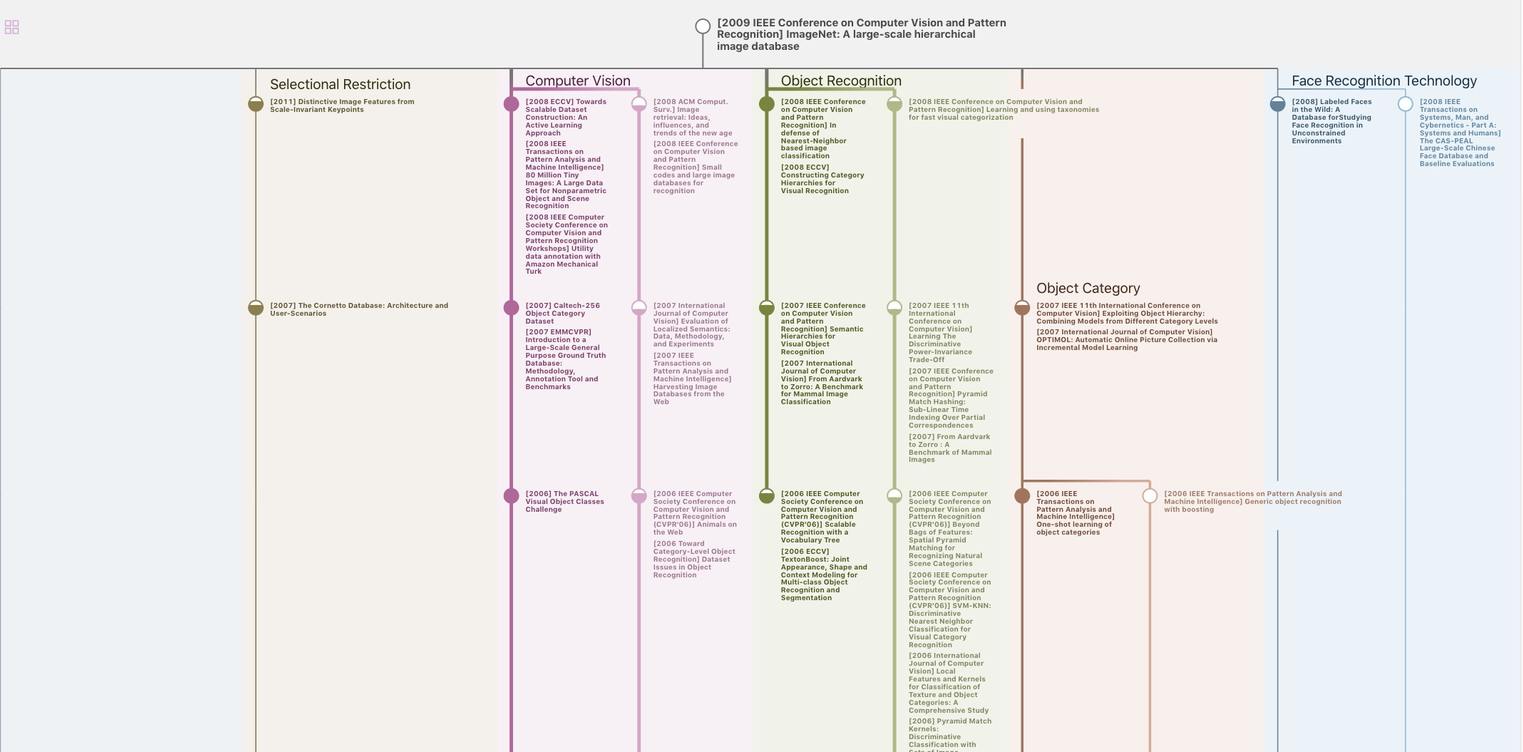
生成溯源树,研究论文发展脉络
Chat Paper
正在生成论文摘要