Thermoacoustic stability prediction using Deep Learning
INTER-NOISE and NOISE-CON Congress and Conference Proceedings(2023)
摘要
Thermoacoustic instabilities are an undesirable physical phenomenon that can occur in a wide range of combustors such as gas turbines, rocket engines, and boilers. These instabilities are typically loud, vibration-inducing, and can increase parietal heat transfer in the combustion chamber. As a consequence, mechanical fatigue increases and can sometimes lead to a catastrophic failure of the combustor. A well-established formalism to predict thermoacoustic stability is based on network models where the combustor is represented by a sequence of connected acoustic modules. The frequency of the modes appearing inside the combustor are then given by the eigenvalues of a characteristic equation obtained using conservation equations. This approach has been successfully used to predict the stability of a variety of combustors. However, this operation needs to be repeated many times in order to optimise the shape of an unstable combustor at an early design stage. One option to reduce the computational cost of predicting the thermoacoustic stability of a given configuration is to use a data-driven approach as opposed to a physics-based approach. In the former approach, a Machine Learning algorithm is trained to discriminate between thermoacoustically stable and unstable combustors using examples generated by a (physics-based) acoustic network model. The ML model is then able to predict the thermoacoustic stability of an unknown configuration much faster than a traditional acoustic network model and with a very high accuracy. This approach has been validated in a previous study using classical Machine Learning algorithms, but it is restricted to somewhat simple geometries. The objective of this study is to investigate whether Deep Learning architectures can be used to generalise those results to complex geometries with a large number of elements.
更多查看译文
关键词
thermoacoustic stability prediction,deep learning
AI 理解论文
溯源树
样例
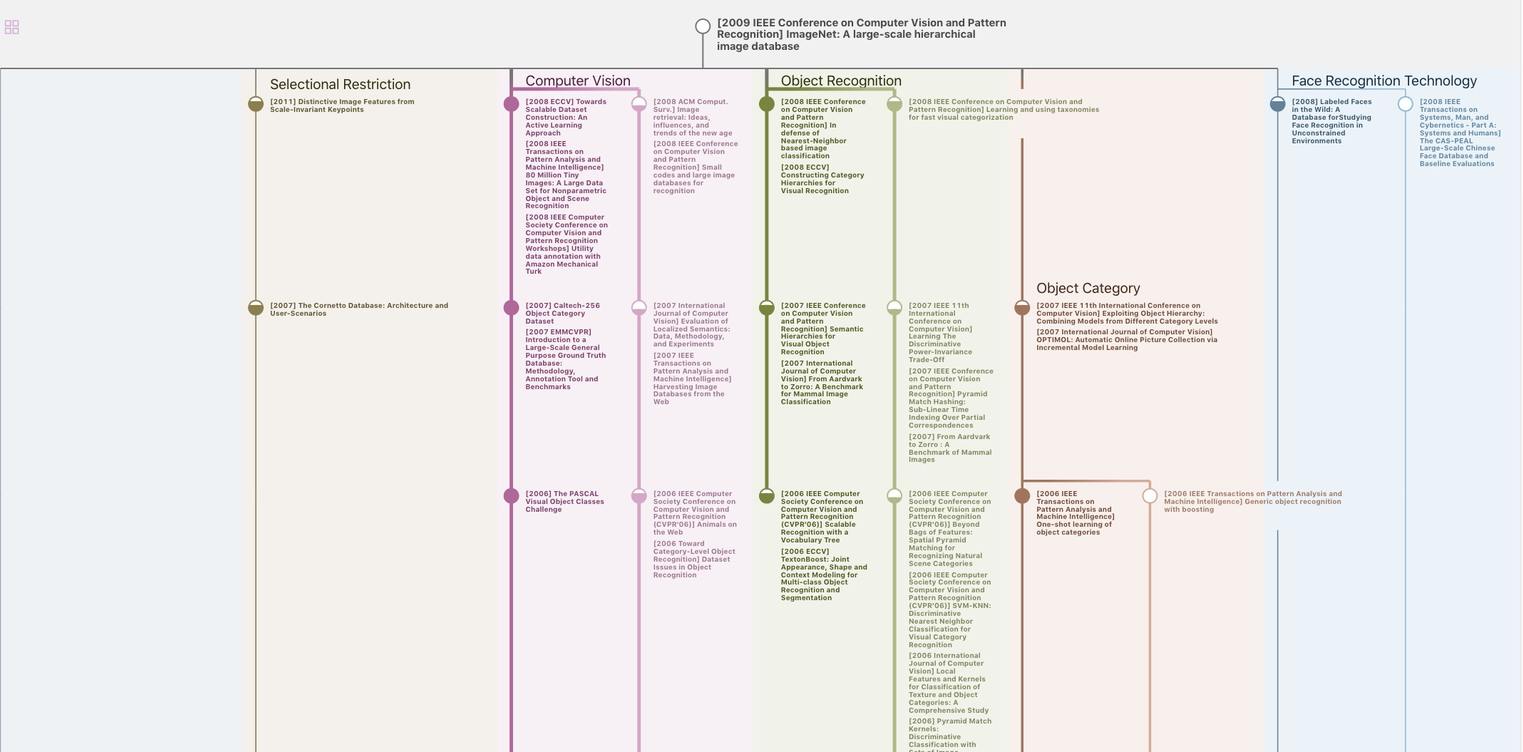
生成溯源树,研究论文发展脉络
Chat Paper
正在生成论文摘要