Prediction and theoretical investigation of dehydrogenation enthalpy of V-Ti-Cr-Fe alloy using machine learning and density functional theory
INTERNATIONAL JOURNAL OF HYDROGEN ENERGY(2024)
摘要
The dehydrogenation enthalpy, a crucial thermodynamic parameter, determines the dif-ficulty of hydrogen desorption in V-Ti-Cr-Fe alloy, but it is unaffordable to predict and modify the dehydrogenation enthalpy and investigate the synergistic effects of structural and chemical parameters relying on conventional experimental methods. In this paper, an extreme gradient boosting (Xgboost) model with feature selection is developed, which can obtain a relative error under 15% in prediction on most of the data. Moreover, the genetic algorithm is utilized for optimizing alloy composition having suitable dehydrogenation enthalpy. The accuracy of prediction and optimization is demonstrated by the subsequent experimental validation, where the error between calculation and experiment is within 4.5%. Density function theory (DFT) is used to analyze the key features provided by ma-chine learning, with results suggesting that the volumes, valence electrons, and bulk module factors have a significant impact. This research supplies an efficient way of using machine learning to predict and optimize the dehydrogenation enthalpy. In addition, DFT calculation also validates the feature importance outputted by machine learning and re-veals the principle of feature effects at the microscopic aspect. (c) 2023 Hydrogen Energy Publications LLC. Published by Elsevier Ltd. All rights reserved.
更多查看译文
关键词
Dehydrogenation enthalpy,V-Ti-Cr-Fe alloy,Machine learning,Density function theory
AI 理解论文
溯源树
样例
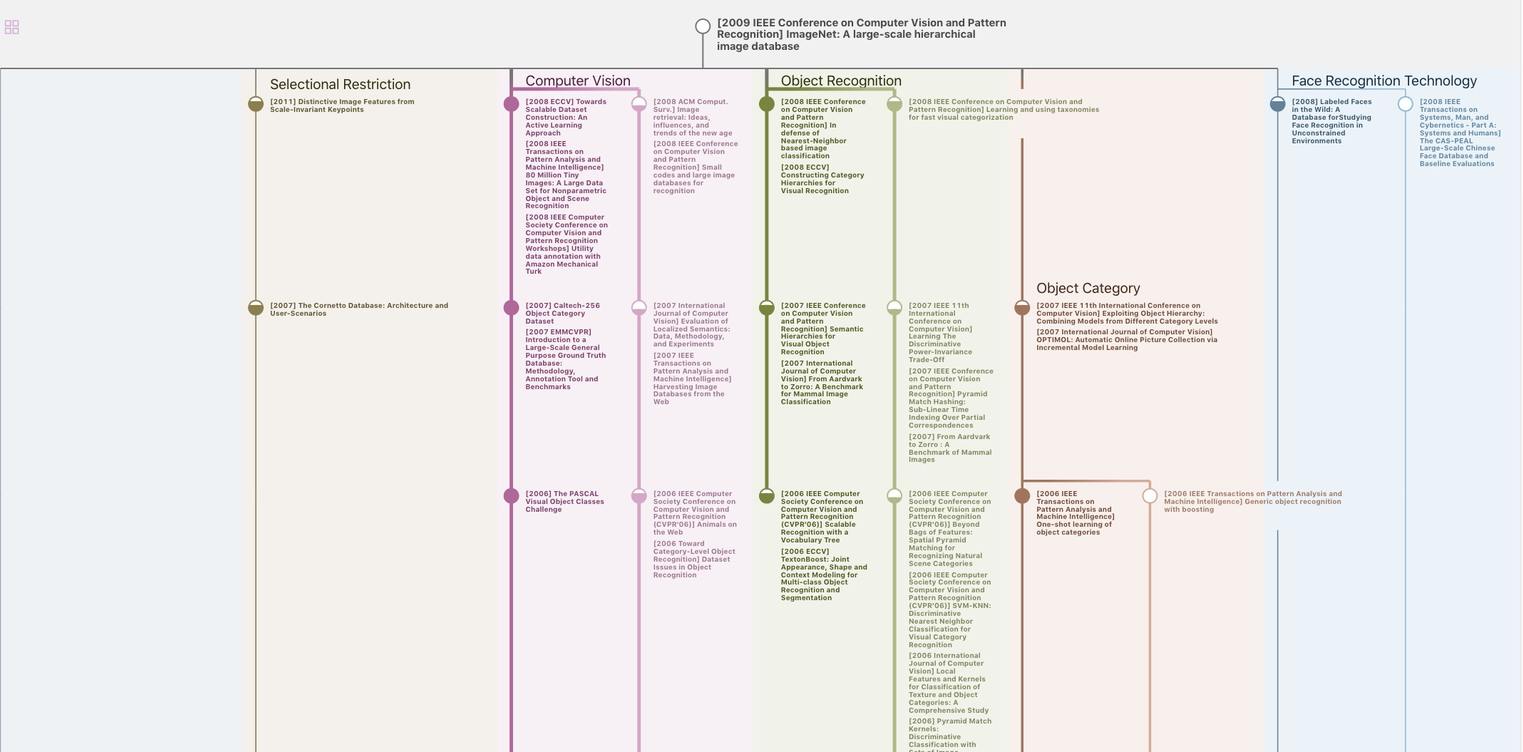
生成溯源树,研究论文发展脉络
Chat Paper
正在生成论文摘要