Fault diagnosis in chemical process systems via Meta-Learning
2022 34th Chinese Control and Decision Conference (CCDC)(2022)
摘要
In the field of chemical process fault diagnosis, there is a common objective problem of less effective fault samples. In many practical chemical process fault application scenarios, the acquisition cost of fault samples is usually very high, and it may be time-consuming and unsafe to collect enough data samples for each fault class. This paper introduces the idea of meta-learning into the field of chemical process systems, and propose few-shot fault diagnosis model based on Meta-Agnostic Meta-Learning (MAML). By realizing the method of automatic learning inner-loop learning rate, the model is optimized to make it converge faster and reduce over-fitting. Finally, the Tennessee Eastman Process (TEP) experiment verifies the performance of the model. The experimental results show that this MAML-LR model has better generalization ability and convergence speed than the MAML-Based model, and it has a good effect on few-shot fault diagnosis.
更多查看译文
关键词
Fault diagnosis,Chemical process systems,Meta learning,Few-shot classification,and Tennessee Eastman Process
AI 理解论文
溯源树
样例
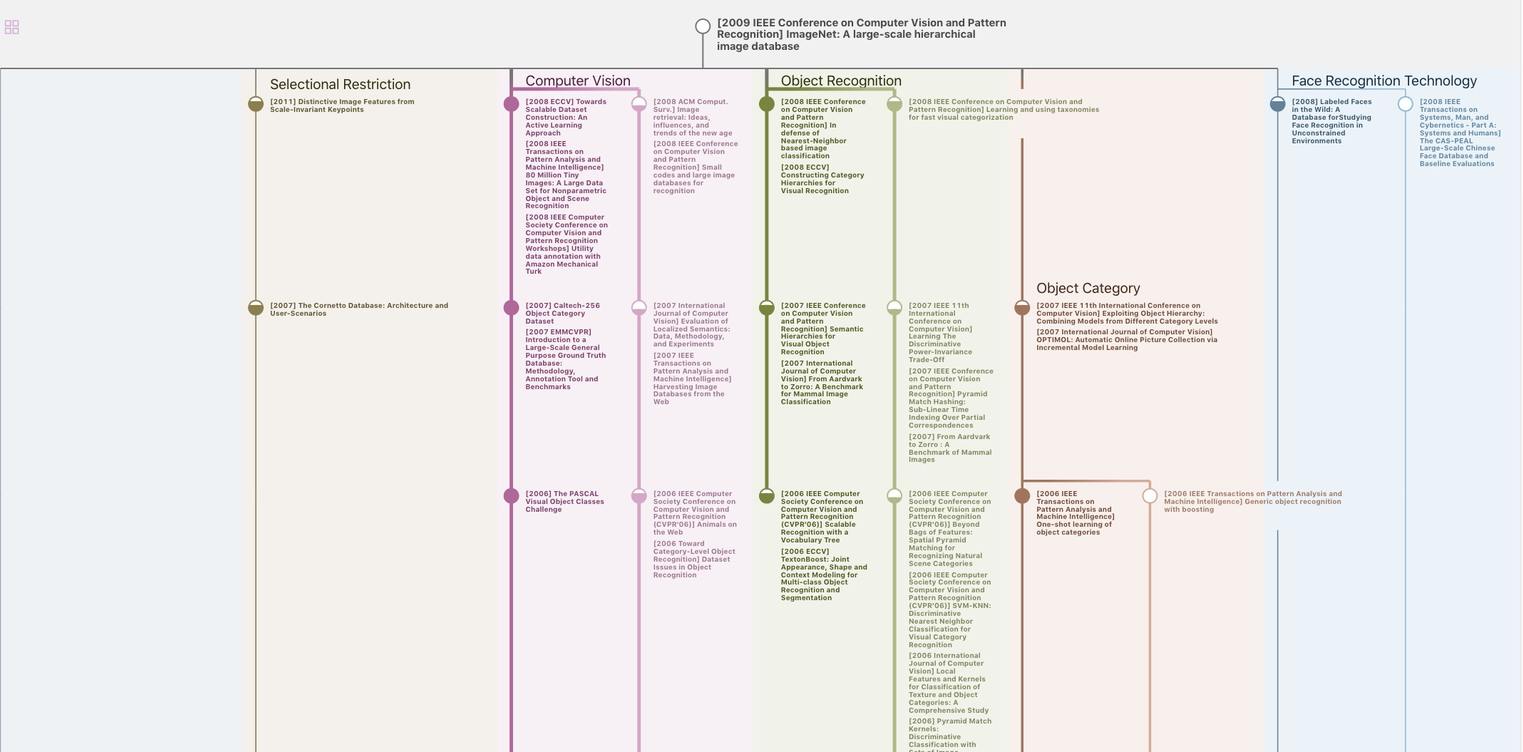
生成溯源树,研究论文发展脉络
Chat Paper
正在生成论文摘要