A Novel Appointed-Time neural networks Control for a Class of High-Order Non-Strict-Feedback Nonlinear Systems with Input Quantization
2022 34th Chinese Control and Decision Conference (CCDC)(2022)
摘要
This paper investigates the problem of appointed-time neural networks based control technique for a large family of high-order non-strict-feedback nonlinear systems with input quantization. A novel appointed-time performance constraint function is designed to guarantee the tracking error converges to a small interval within the user pre-specified time. What’s more, the RBF neural networks are used to handle with the unknown sections of the system. Besides, the problem of input quantization is solved by introducing a separate lemma which converts the quantized input into a "linear-like" form. All the signals remain bounded, and the tracking error converges to a vere low level eventually. Finally, a simulation example proves the effectiveness of the proposed scheme.
更多查看译文
关键词
neural networks control,nonlinear systems,input quantization,appointed-time,high-order,non-strict-feedback
AI 理解论文
溯源树
样例
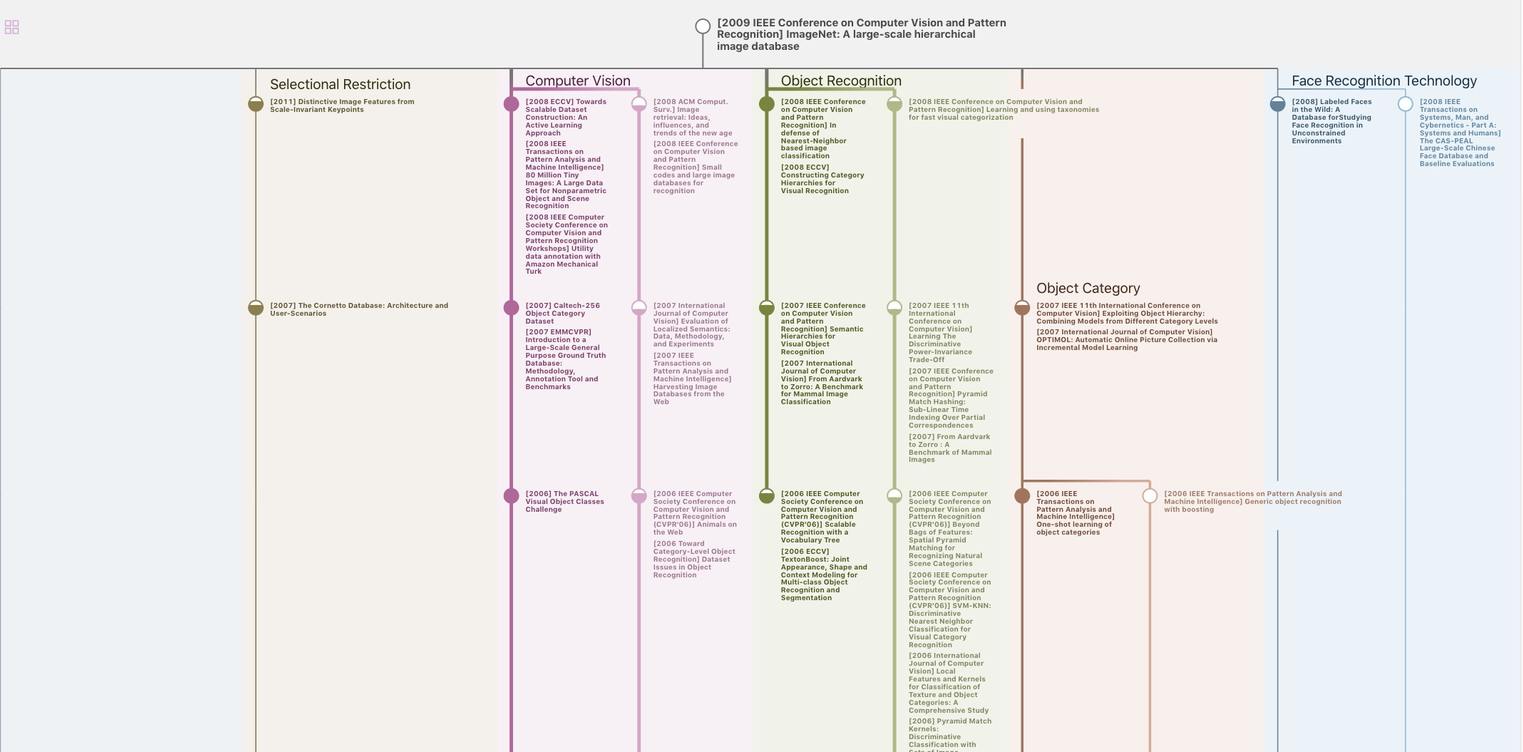
生成溯源树,研究论文发展脉络
Chat Paper
正在生成论文摘要