Reinforcement Learning Based Control of an Organic Rankine Cycle Waste Heat Recovery System Over a Drive Cycle for Heavy-Duty Diesel Engines
ASME 2022 ICE Forward Conference(2022)
摘要
Abstract Organic Rankine cycle (ORC) waste heat recovery (WHR) systems are of interest in the automotive sector because of their ability to convert exhaust heat into usable energy, increasing system-level brake thermal efficiency. Interest is particularly strong in the heavy-duty market as these vehicles have large footprints and heat outputs that can accommodate ORC-WHR systems. ORC-WHR systems are in the early stages of development and as a result the effectiveness of ORC-based systems throughout transient drive cycles can be difficult to quantify. One of the primary barriers preventing insight into transient performance is the lack of control maturity that exists prior to hardware implementation. Early development of control strategies allows for insights to be integrated into design earlier in the process, potentially streamlining the development cycle. ORC control development is hindered by the physical complexity of the boiler and the modeling of associated dynamics. Creating a model that captures the warm-up, power-generation, and cool-down operating regimes requires the use of a finite volume-based model (FVM) which is computationally infeasible to use with optimal control techniques such as model predictive control or dynamic programming. If the model is switched to a control oriented moving boundary model (MBM) the ability to govern the warmup and cool-down operating regimes is eliminated. This research proposes the use of a model-free reinforcement learning (RL) algorithm to create a control strategy using a FVM system model. The model-free nature of RL allows it to be trained/calibrated on models of unlimited complexity with the resulting controller capable of real-time operation. This eliminates the need for model reduction during control strategy generation and allows for the controller to handle all operating regimes the system will encounter. This study utilizes a deep deterministic policy gradient (DDPG) RL agent trained on a FVM of an ORC-WHR system. The RL agent is trained over a heavy-duty drive cycle and is capable of extracting net positive work over a transient drive cycle.
更多查看译文
AI 理解论文
溯源树
样例
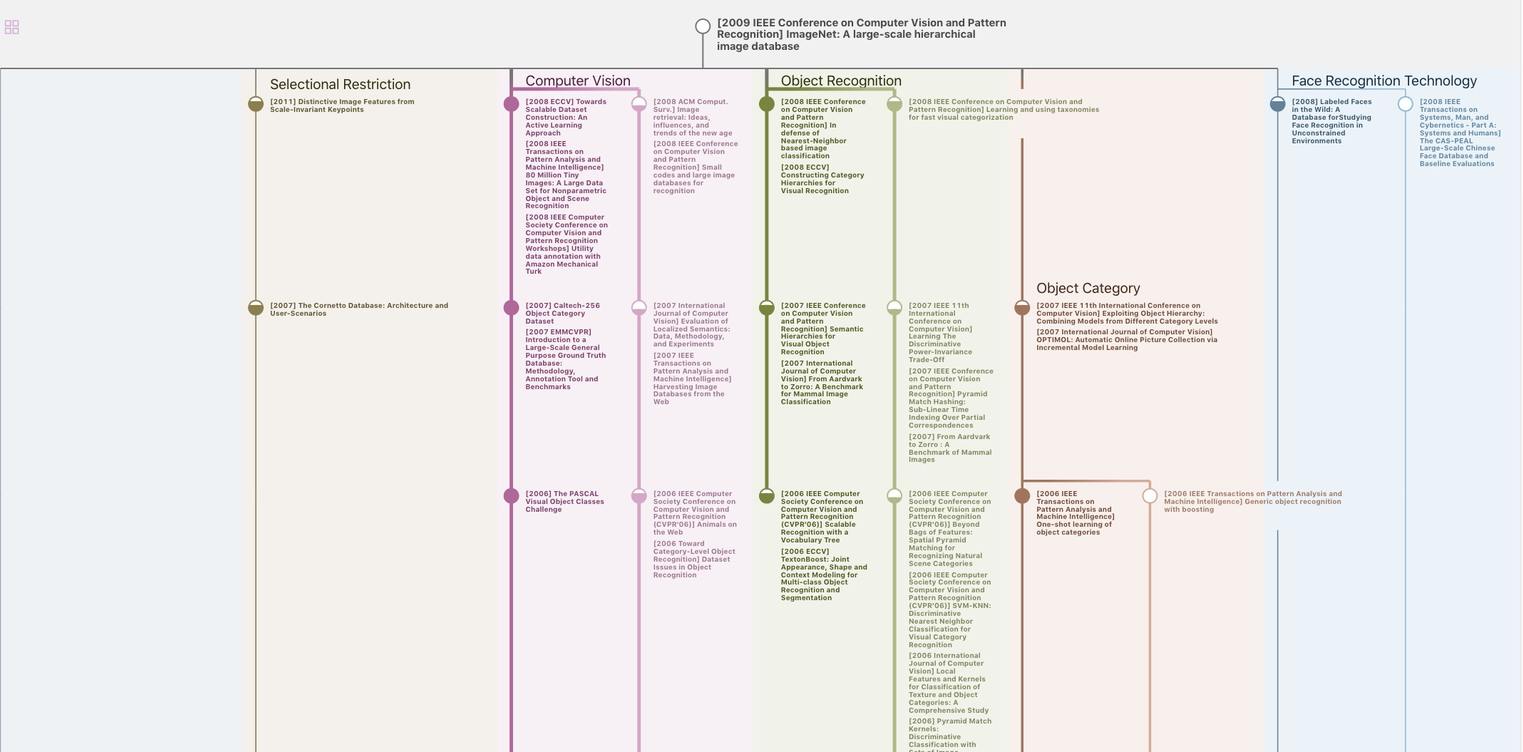
生成溯源树,研究论文发展脉络
Chat Paper
正在生成论文摘要