A Battery Aging Mode Identification Framework: Encompassing Multiple Cell Chemistries, Electrode Designs, and Use Conditions
ECS Meeting Abstracts(2022)
摘要
A key challenge for Lithium-ion batteries (LiB) is the capability to manage battery performance and predict the battery’s lifetime, which are crucial information for optimum operation of electric-vehicles (EVs). In that regard, early detection of battery-aging phenomena and their implications on performance is necessary for maintaining warranty and avoiding safety-related liabilities. However, rapid diagnosis of the root causes of battery degradation has not yet been well-developed nor studied upon a broad mixture of cell designs and use cases. Here, a machine-learning (ML)- based framework is presented that aims at identifying aging modes or mechanisms during battery cycling. Cycle-by-cycle electrochemical signatures (e.g., end-of-rest voltages, columbic efficiency, and capacity fade) were analyzed then correlated with aging modes, such as loss of active materials in the cathode (LAMPE) or loss of Li inventory (LLI). Multiple battery designs and use conditions were analyzed to develop this ML framework, including 44 single layer pouch cells representing two cathode chemistries (NMC532 and NMC811), two electrode loadings (low and moderate), and five charging rates (1, 4, 4.5, 6, and 9C CC-CV profiles). Predominant aging behaviors depend on both the cell design and use. Aging behaviors can be classified into a combination of LAMPE and LLI. A classification accuracy of 90% was achieved using only the first 200aging cycles, and the percentage of LAMPE was predicted with 4.3% of error after 600 cycles. As seen here, the overall aging behavior of the battery was found to be dependent on cathode chemistry, electrode build, and usage conditions. This ML framework is promising for battery developers to save the time and effort that is typically associated with prolonged testing. The ML framework will also advise on innovative design strategies to counter specific degradation modes, thus significantly accelerating the development cycle for building longer lasting batteries. From the end user’s perspective, this aging-mode diagnosis framework enables the ability to fine-tune battery service scenarios to minimize degradations thereby improving both lifetime and safety in EVs or stationary storage systems. Figure 1
更多查看译文
关键词
electrode designs,multiple cell chemistries,aging
AI 理解论文
溯源树
样例
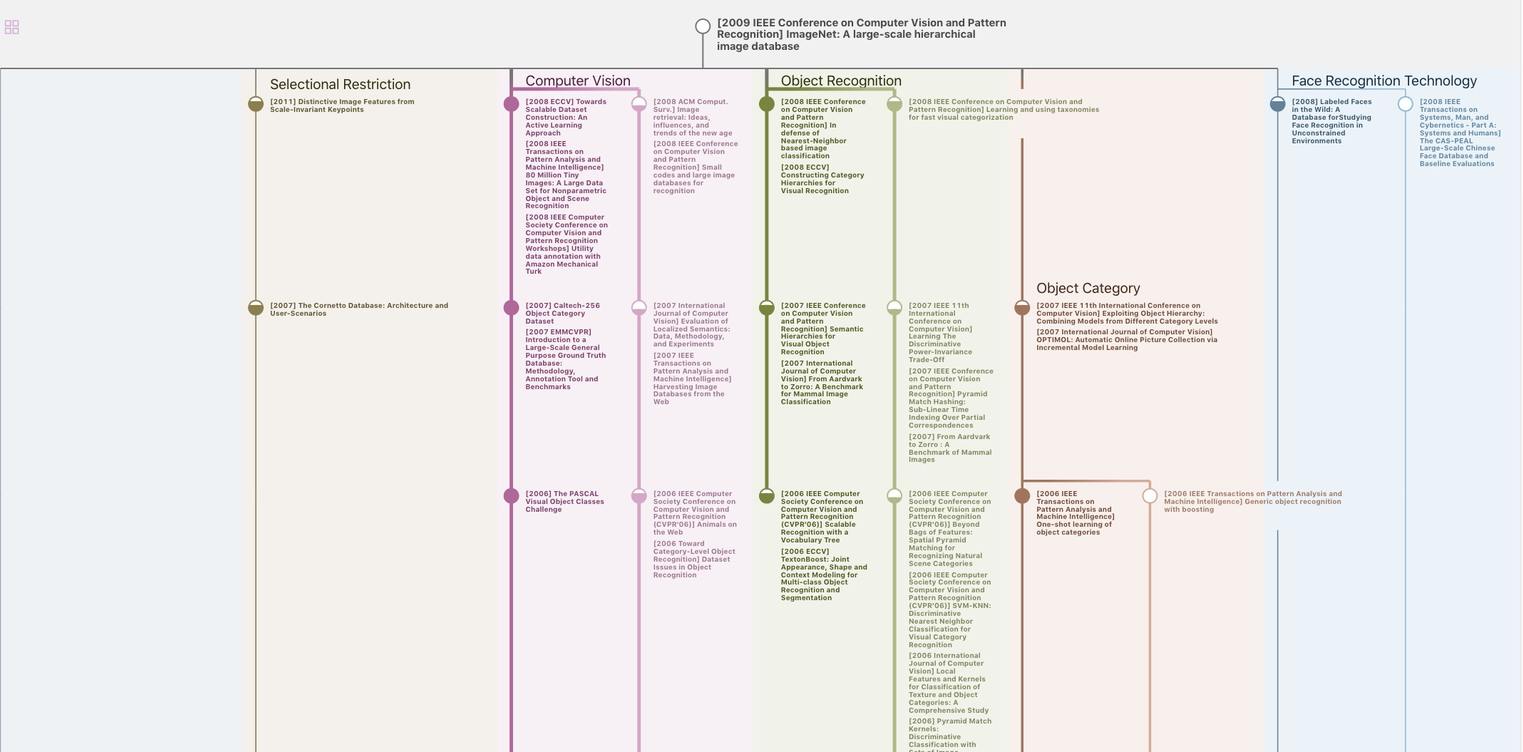
生成溯源树,研究论文发展脉络
Chat Paper
正在生成论文摘要