Cell morphology-guided de novo hit design by conditioning GANs on phenotypic image features
Digital Discovery(2023)
摘要
Developing novel bioactive molecules is time-consuming, costly and rarely successful. As a mitigation strategy, we utilize, for the first time, cellular morphology to directly guide the de novo design of small molecules. We trained a conditional generative adversarial network on a set of 30 000 compounds using their cell painting morphological profiles as conditioning. Our model was able to learn chemistry-morphology relationships and influence the generated chemical space according to the morphological profile. We provide evidence for the targeted generation of known agonists when conditioning on gene overexpression profiles, even though no information on biological targets was used during training. Based on a target-agnostic readout, our approach facilitates knowledge transfer between biological pathways and can be used to design bioactives for many targets under one unified framework. Prospective application of this proof-of-concept to larger chemical spaces promises great potential for hit generation in drug and phytopharmaceutical discovery and chemical safety.
更多查看译文
关键词
gans,phenotypic image features,cell,morphology-guided
AI 理解论文
溯源树
样例
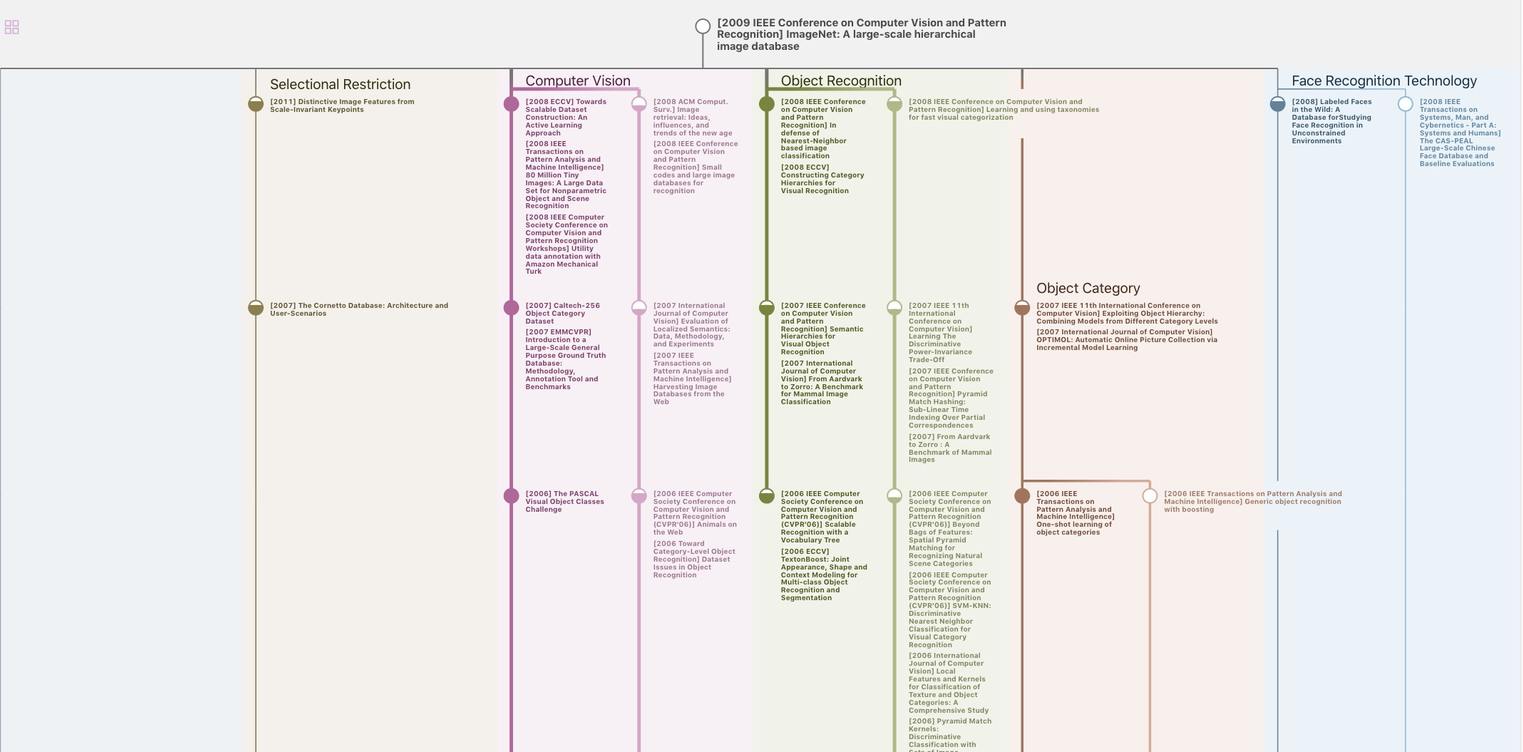
生成溯源树,研究论文发展脉络
Chat Paper
正在生成论文摘要