Next Job Application Prediction by Leveraging Textual Information, Metadata, and Personalized-Attention Mechanism
Deep Learning Applications, Volume 4Advances in Intelligent Systems and Computing(2022)
摘要
Prediction of the next job application is one of the most important use-cases of job recommender systems. This work proposes to use next-item recommendation methods to model job seekers’ career preferences to more accurately discover the next job postings they may apply for. Our proposed model, Personalized-Attention Next-Application Prediction (PANAP), consists of three modules. The first module learns job posting representations from textual content and metadata attributes in an unsupervised way. The second module learns job seeker representations from job application records and metadata attributes. It includes a personalized-attention mechanism that adapts the importance of each job in the learned career preference representation to the profile of a specific job seeker. The third module then models the Next-Application Prediction task as a top-K search process based on the similarity of representations. Text content plays a major role in describing job postings, and how the job content is encoded affects the model performance. Therefore, we explore the utility of different content encoding methods. In addition, geographic location is another essential factor influencing the preferences of job seekers in the recruitment domain. We also explore the influence of geographic location on the model performance from the perspective of negative sampling strategies.
更多查看译文
关键词
prediction,textual information,application,job,personalized-attention
AI 理解论文
溯源树
样例
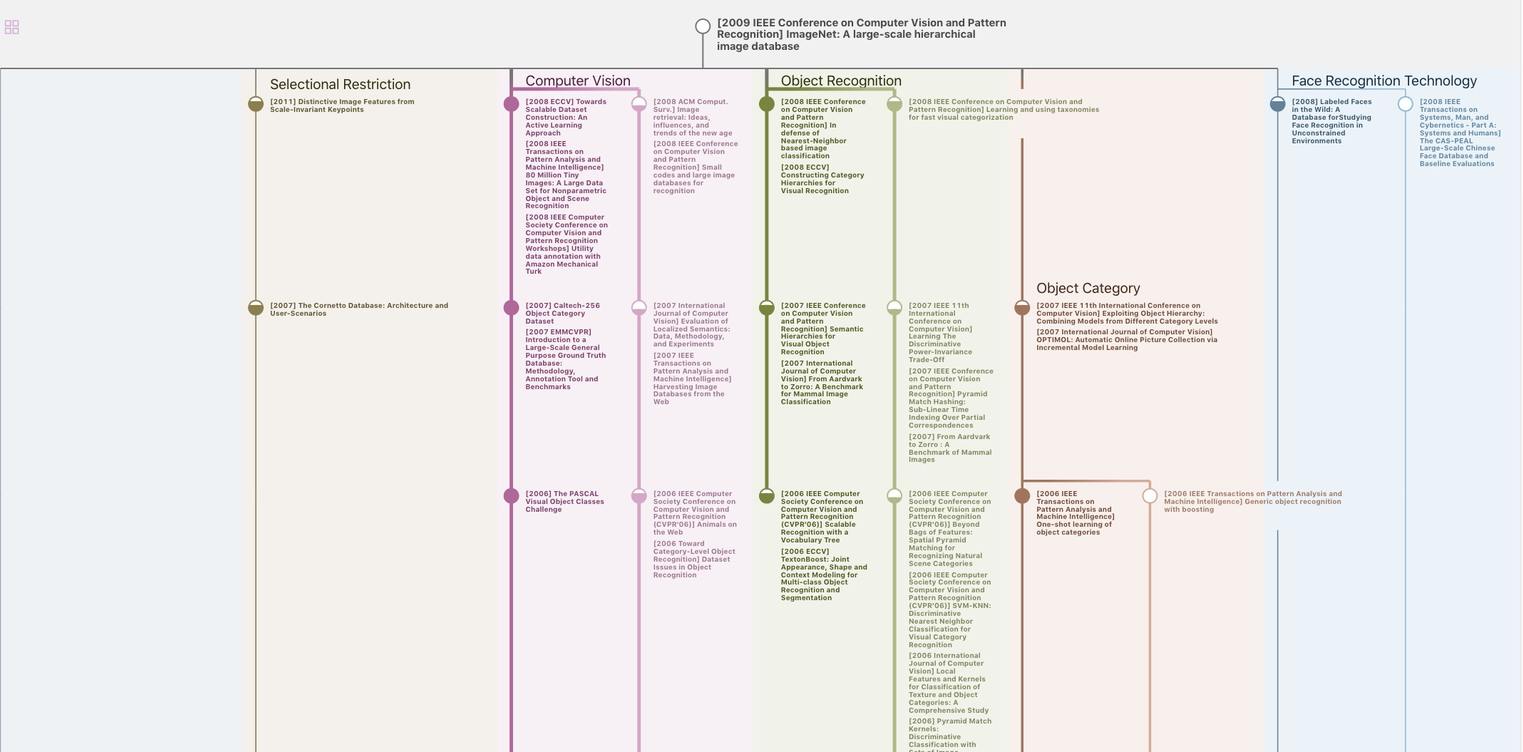
生成溯源树,研究论文发展脉络
Chat Paper
正在生成论文摘要