Sparse Convolutional Neural Networks for particle classification in ProtoDUNE-SP events
Journal of Physics: Conference Series(2023)
摘要
Deep Learning (DL) methods and Computer Vision are becoming important tools for event reconstruction in particle physics detectors. In this work, we report on the use of submanifold sparse convolutional neural networks (SparseNets) for the classification of track and shower hits from a DUNE prototype liquid-argon detector at CERN (ProtoDUNE-SP). By taking advantage of the three-dimensional nature of the problem we use a set of nine input features to classify sparse and locally dense hits associated to track or shower particles. The SparseNet has been trained on a test sample and shows promising results: efficiencies and purities greater than 90%. This has also been achieved with a considerable speedup and substantially less resource utilization with respect to other DL networks such as graph neural networks. This method offers great scalability advantages for future large neutrino detectors such as the planned DUNE experiment.
更多查看译文
关键词
particle classification,events,neural networks
AI 理解论文
溯源树
样例
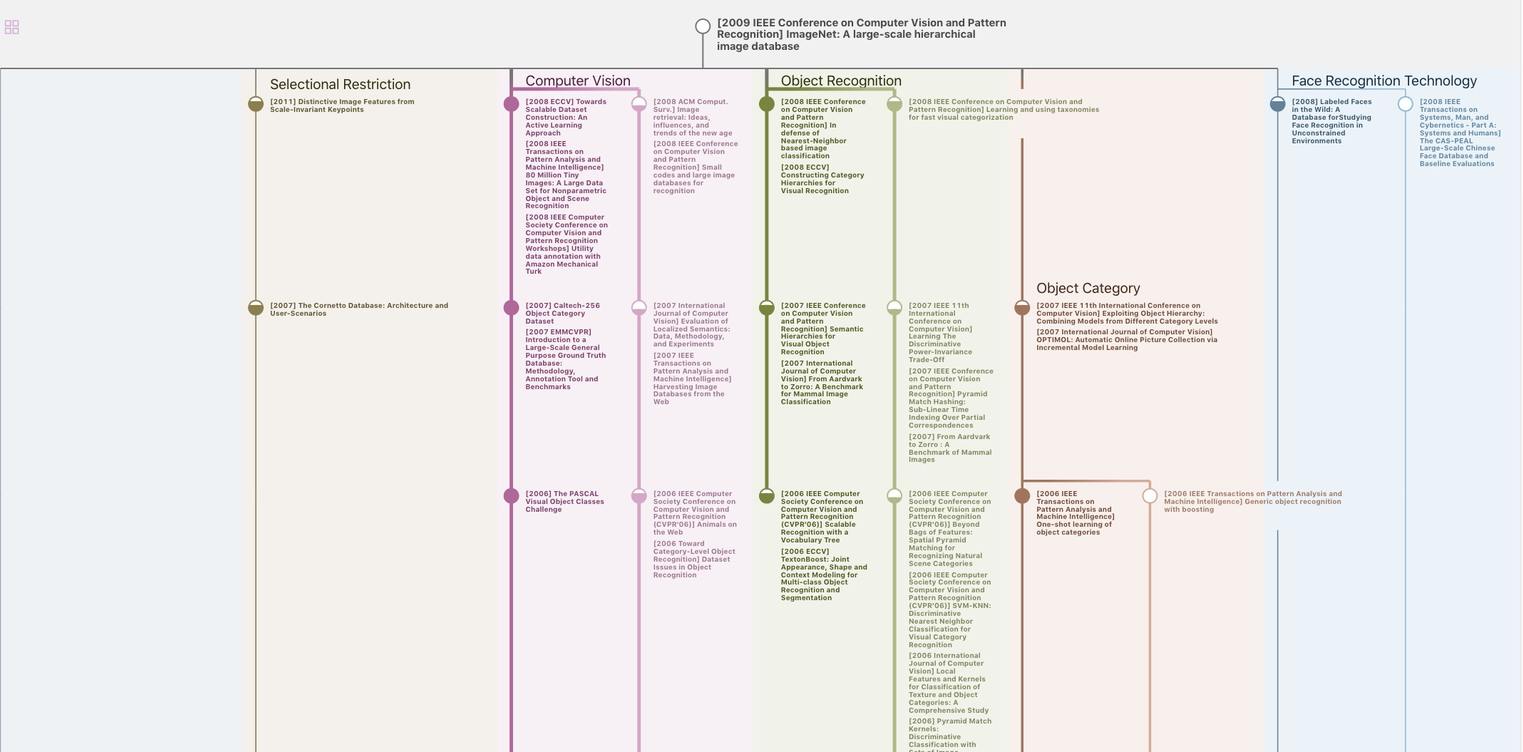
生成溯源树,研究论文发展脉络
Chat Paper
正在生成论文摘要