Death comes but why: A multi-task memory-fused prediction for accurate and explainable illness severity in ICUs
WORLD WIDE WEB-INTERNET AND WEB INFORMATION SYSTEMS(2023)
摘要
Predicting the severity of an illness is crucial in intensive care units (ICUs) if a patient‘s life is to be saved. The existing prediction methods often fail to provide sufficient evidence for time-critical decisions required in dynamic and changing ICU environments. In this research, a new method called MM-RNN (multi-task memory-fused recurrent neural network) was developed to predict the severity of illnesses in intensive care units (ICUs). MM-RNN aims to address this issue by not only predicting illness severity but also generating an evidence-based explanation of how the prediction was made. The architecture of MM-RNN consists of task-specific phased LSTMs and a delta memory network that captures asynchronous feature correlations within and between multiple organ systems. The multi-task nature of MM-RNN allows it to provide an evidence-based explanation of its predictions, along with illness severity scores and a heatmap of the patient’s changing condition. The results of comparison with state-of-the-art methods on real-world clinical data show that MM-RNN delivers more accurate predictions of illness severity with the added benefit of providing evidence-based justifications.
更多查看译文
关键词
Personalized healthcare,Illness severity prediction,Explainable prediction,Time series
AI 理解论文
溯源树
样例
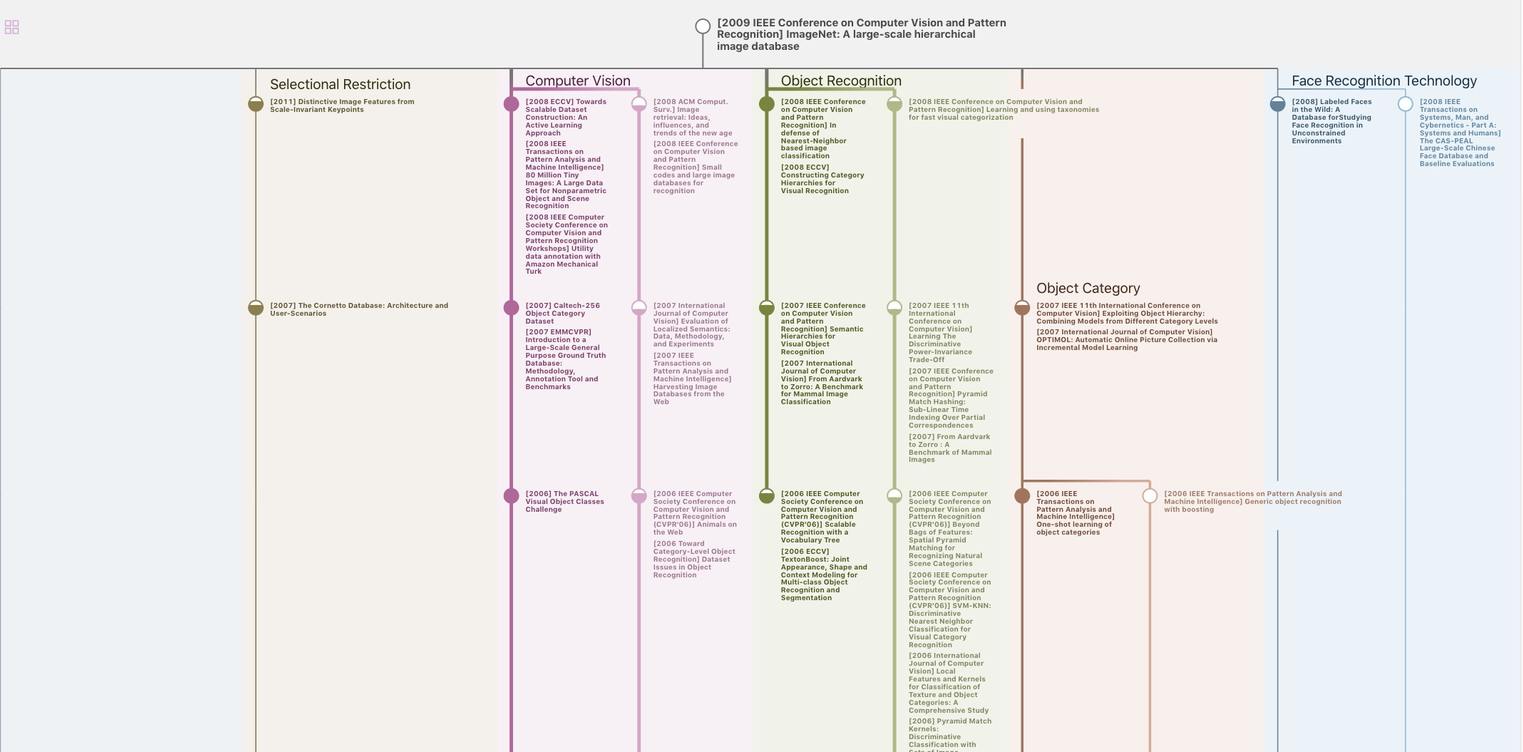
生成溯源树,研究论文发展脉络
Chat Paper
正在生成论文摘要